Combining Programmable Potentials and Neural Networks for Materials Problems.
AAAI Spring Symposium - MLPS(2021)
摘要
Force field methods can be used in molecular dynamics simulations to compute and infer macro-level properties of chemical or material systems. However, these force fields can often predict erroneous values due to not being accurate at the quantum level. The ideal would be able to simulate the system from first principles (quantum-level) and scale up to get the material properties. However, this is computationally infeasible. In this paper, we develop a Programmable Potentials methodology that utilizes high-fidelity QM/MM data sets to inform a molecular dynamics (MD) potential. This methodology uses encoding functions containing small neural networks that automatically learn the quantum-level interaction logic of the system from the data and uses these encoding functions to modify standard MD potentials, like LennardJones, which capture the correct long range behavior, but do poorly in the near range. We test the method on the adsorption of hydrocarbons in a zeolite framework. We show that the encoding functions, and the resulting model, generalize well outside their training set and that encoding functions trained with data from a simpler hydrocarbon such as CH4 can build good models for more complex hydrocarbons such as C2H6 and C3H8.
更多查看译文
关键词
Computational Chemistry,Molecular Simulations,Shape Selectivity,Structure Determination,Nanoparticles
AI 理解论文
溯源树
样例
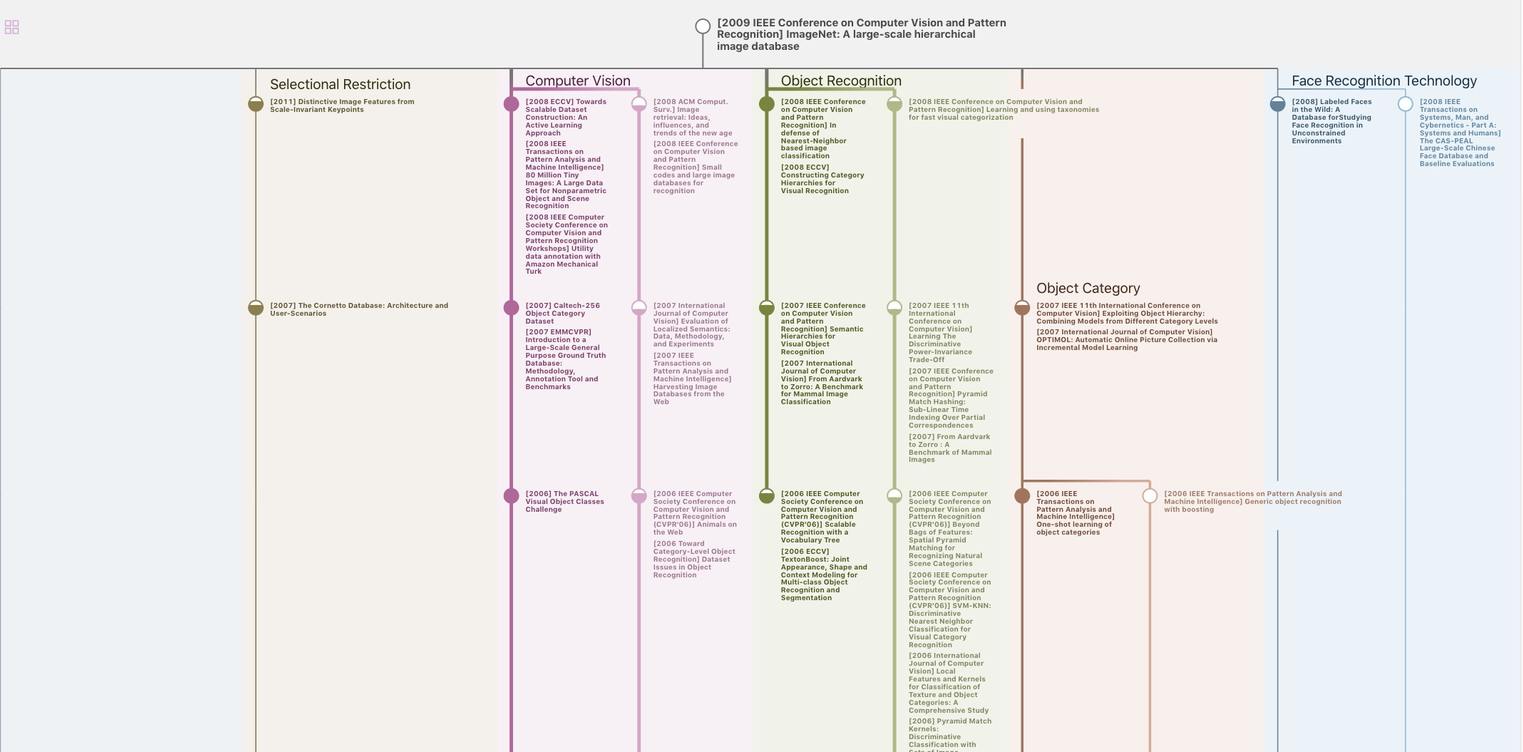
生成溯源树,研究论文发展脉络
Chat Paper
正在生成论文摘要