A Comparative Evaluation of Deep Learning Anomaly Detection Techniques on Semiconductor Multivariate Time Series Data.
CASE(2021)
摘要
In industrial processes, keeping equipment units in good operating conditions while reducing maintenance costs is one of the most important objectives to improve productivity. One of the ways to do so is to early detect equipment dysfunction. This can be done by analyzing the massive amounts of data collected via numerous sensors during production activities. Thanks to the advantages of distributed architecture and computation efficiency improvements, deep learning methods have gained much interest and have been investigated by researchers for thorough industrial data analysis, notably for anomaly detection. A comparative evaluation of data-driven deep learning methods used to detect anomalies occurring during equipment processing is proposed. This evaluation is done on a total of six methods, their ability to detect anomalies on raw sensor data, collected on semiconductor machines, with variable correlations and temporal dependencies is discussed. The evaluation gives an insight on the industrial performances on the methods and shows how the supervised learning methods outperform the other models with less training time but need labelled data meanwhile some self-supervised learning methods have good detection performances with training done on normal data only.
更多查看译文
关键词
Anomaly detection,data-driven methods,deep learning,multivariate analysis,raw sensor data,semiconductor manufacturing
AI 理解论文
溯源树
样例
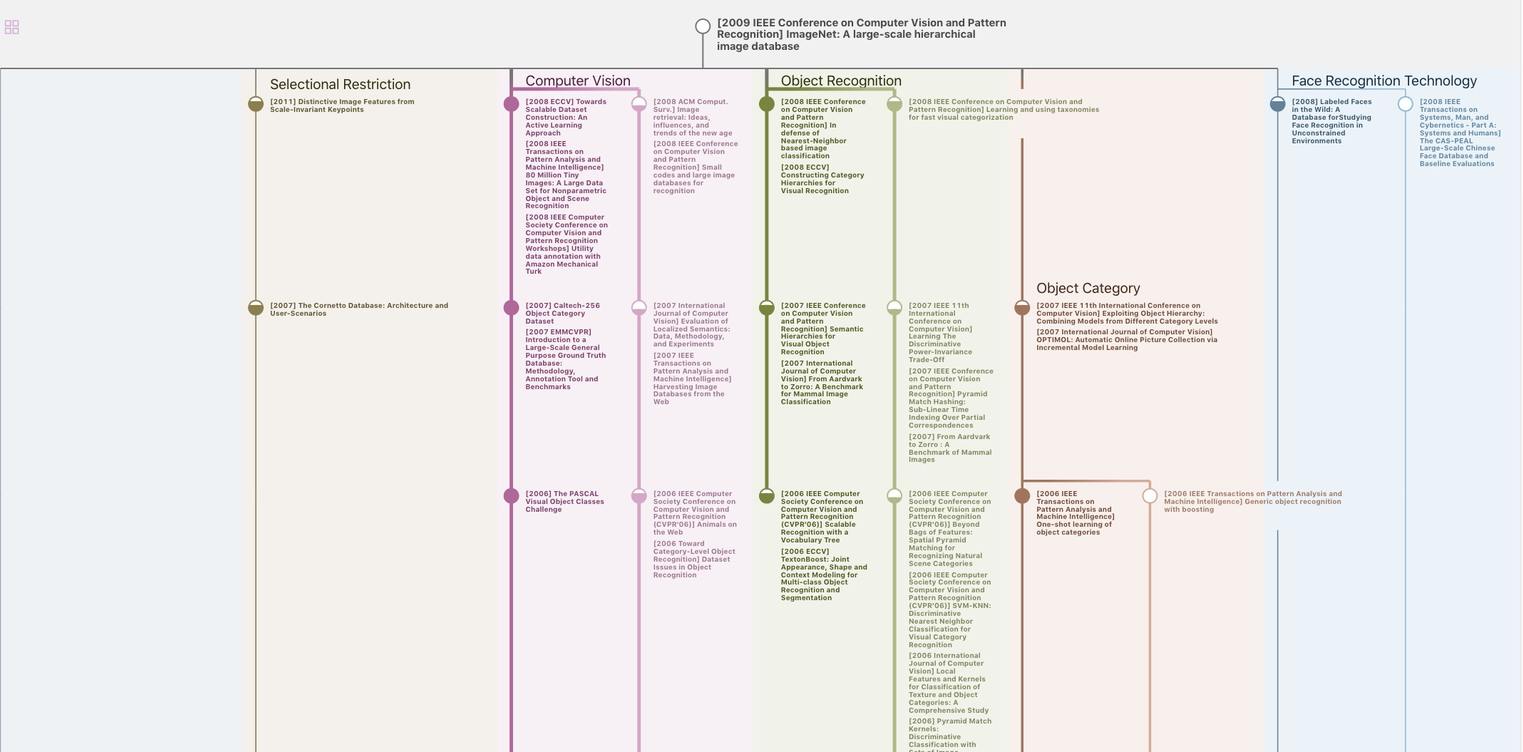
生成溯源树,研究论文发展脉络
Chat Paper
正在生成论文摘要