Predicting length of stay with administrative data from acute and emergency care - an embedding approach.
CASE(2021)
摘要
Hospital beds management is critical for the quality of patient care, while length of inpatient stay is often estimated empirically by physicians or chief nurses of medical wards. Providing an efficient method for forecasting the length of stay (LOS) is expected to improve resources and discharges planning. Predictions should be accurate and work for as many patients as possible, despite their heterogeneous profiles. In this work, a LOS prediction method based on deep learning and embeddings is developed by using generic hospital administrative data from a French national hospital discharge database, as well as emergency care. Data concerned 497 626 stays of 304 931 patients from 6 hospitals in Lyon, France, from 2011 to 2019. Results of a 5-fold cross-validation showed an accuracy of 0.73 and a kappa score of 0.67 for the embeddings method. This outperformed the baseline which used the raw input features directly.
更多查看译文
关键词
predicting length,emergency care,embedding approach,hospital beds management,patient care,inpatient stay,physicians,chief nurses,medical wards,discharges planning,heterogeneous profiles,LOS prediction method,deep learning,generic hospital administrative data,French national hospital discharge database,embeddings method
AI 理解论文
溯源树
样例
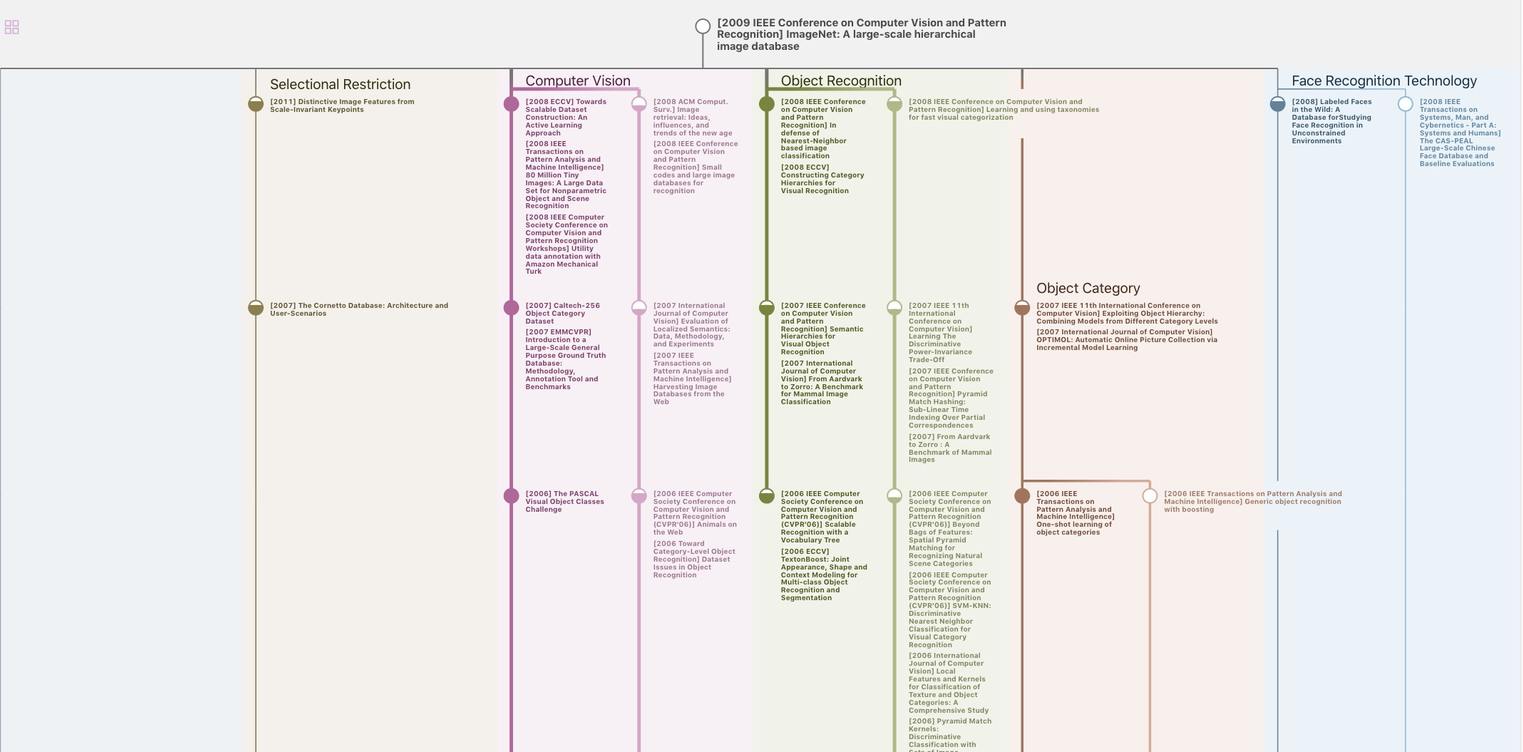
生成溯源树,研究论文发展脉络
Chat Paper
正在生成论文摘要