Mini-Me, You Complete Me! Data-Driven Drone Security via DNN-based Approximate Computing.
RAID(2021)
摘要
The safe operation of robotic aerial vehicles (RAV) requires effective security protection of their controllers against cyber-physical attacks. The frequency and sophistication of past attacks against such embedded platforms highlight the need for better defense mechanisms. Existing estimation-based control monitors have tradeoffs, with lightweight linear state estimators lacking sufficient coverage, and heavier data-driven learned models facing implementation and accuracy issues on a constrained real-time RAV. We present Mini-Me, a data-driven online monitoring framework that models the program-level control state dynamics to detect runtime data-oriented attacks against RAVs. Mini-Me leverages the internal dataflow information and control variable dependencies of RAV controller functions to train a neural network-based approximate model as the lightweight replica of the original controller programs. Mini-Me runs the minimal approximate model and detects malicious control state deviation by comparing the estimated outputs with those outputs calculated by the original controller program. We demonstrate Mini-Me on a widely adopted RAV physical model as well as popular RAV virtual models based on open-source firmware, ArduPilot and PX4, and show its effectiveness in detecting five types of attack cases with an average 0.34% space overhead and 2.6% runtime overhead.
更多查看译文
关键词
drone security,computing,data-driven,dnn-based
AI 理解论文
溯源树
样例
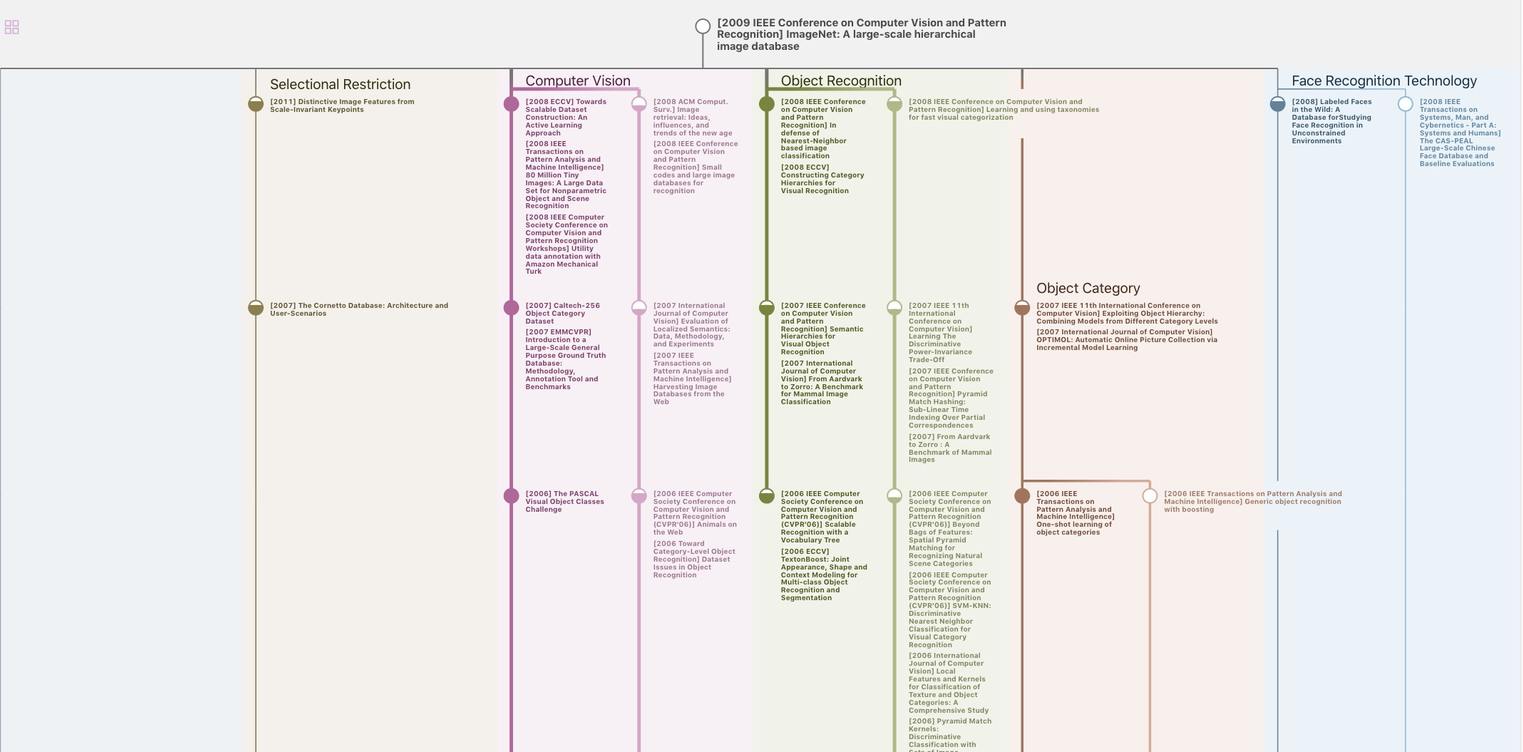
生成溯源树,研究论文发展脉络
Chat Paper
正在生成论文摘要