Comparing the Explainability and Performance of Reinforcement Learning and Genetic Fuzzy Systems for Safe Satellite Docking
EXPLAINABLE AI AND OTHER APPLICATIONS OF FUZZY TECHNIQUES, NAFIPS 2021(2022)
Abstract
As the number of satellites in orbit is increasing, a shift to autonomous operations is essential. Autonomous control systems have the potential to increase efficiency and decrease cost by reducing the ratio of human operators on the ground to spacecraft in orbit. Machine learning algorithms such as Reinforcement Learning (RL) and Genetic Fuzzy Systems (GFS) are excellent candidates to be applied to space systems, as they have demonstrated the ability to surpass human performance in complex, high dimensional systems. When applied to a physical system, an autonomous controller needs to be safe, effective, and explainable so that a human operator can trust that the controller will complete a task efficiently without making any mistakes. In this paper, the performance of controllers trained with both RL and GFS are compared. Both controllers implement a real time safety filter to ensure safety while training. While RL is shown to have very high performance, it suffers from a lack of explainability. GFS is shown to balance these two characteristics, as it has comparable performance and is much more explainable.
MoreTranslated text
AI Read Science
Must-Reading Tree
Example
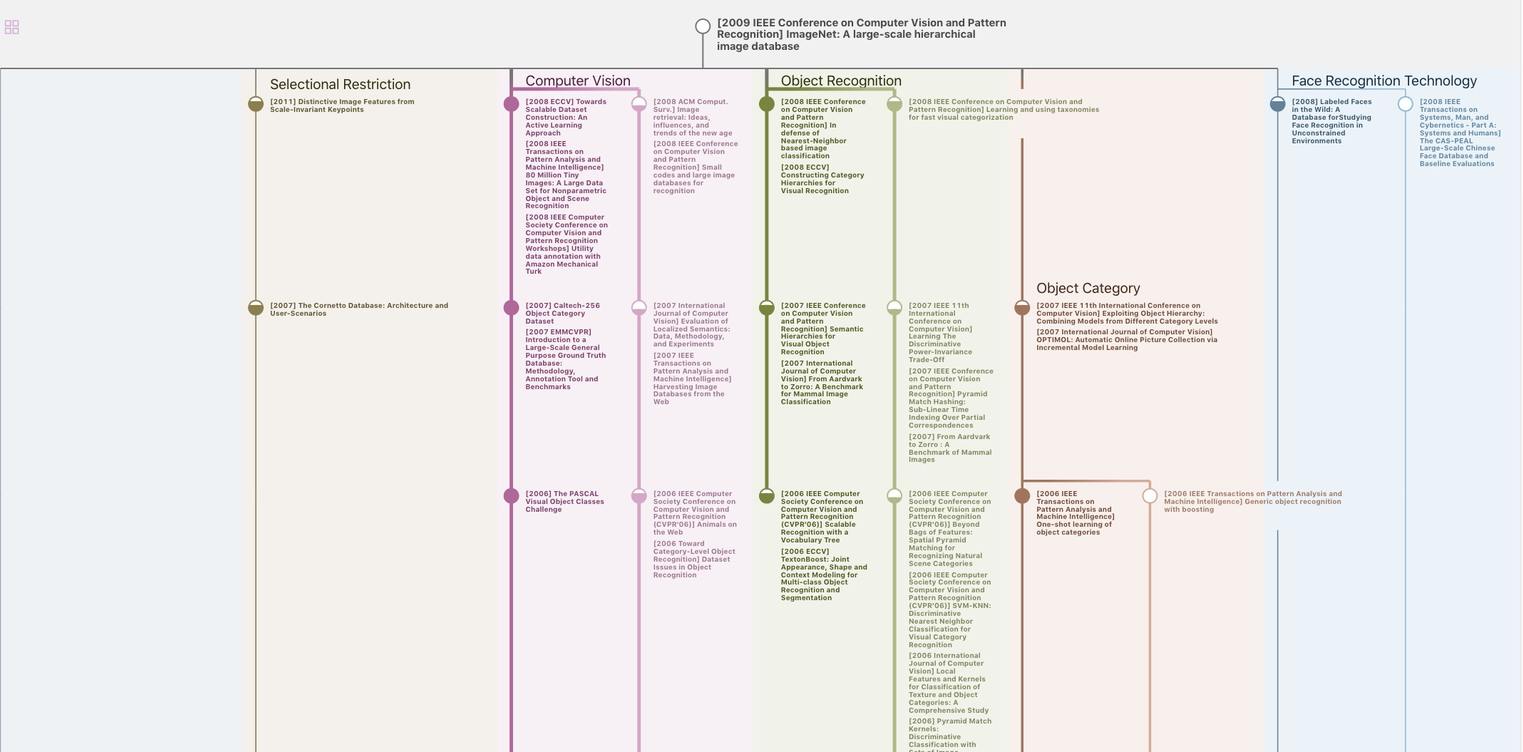
Generate MRT to find the research sequence of this paper
Chat Paper
Summary is being generated by the instructions you defined