On the Estimation of POD and LOD of Qualitative Microbiological Assays from a Multi-Laboratory Validation Study
JOURNAL OF AOAC INTERNATIONAL(2022)
摘要
Background Jarvis et al. in 2019 (J. AOAC Int. 102: 1617-1623) estimated the mean laboratory effect (mu), standard deviation of laboratory effects (sigma), probability of detection (POD), and level of detection (LOD) from a multi-laboratory validation study of qualitative microbiological assays using a random intercept complementary log-log model. Their approach estimated sigma based on a Laplace approximation to the likelihood function of the model, but estimated mu from a fixed effectmodel due to a limitation in the MS Excel spreadsheet which was used by the authors to develop a calculation tool. Objective We compared the estimates of mu and sigma from three approaches (the Laplace approximation that estimates mu and sigma simultaneously from the random intercept model, adaptive Gauss-Hermite quadrature (AGHQ), and the method of Jarvis et al.) and introduced an R Shiny app to implement the AGHQ using the widely used "lme4" R package. Methods We conducted a simulation study to compare the accuracy of the estimates of mu and sigma from the three approaches and compared the estimates of mu, sigma, LOD, etc. between the R Shiny app and the spreadsheet calculation tool developed by Jarvis et al. for an example dataset. Results Our simulation study shows that, while the three approaches produce similar estimates of sigma, the AGHQ has generally the best performance for estimating mu (and hence mean POD and LOD). The differences in the estimates between the R Shiny app and the spreadsheet were demonstrated using the example dataset. Conclusion The AGHQ is the best method for estimating mu, POD, and LOD.
更多查看译文
AI 理解论文
溯源树
样例
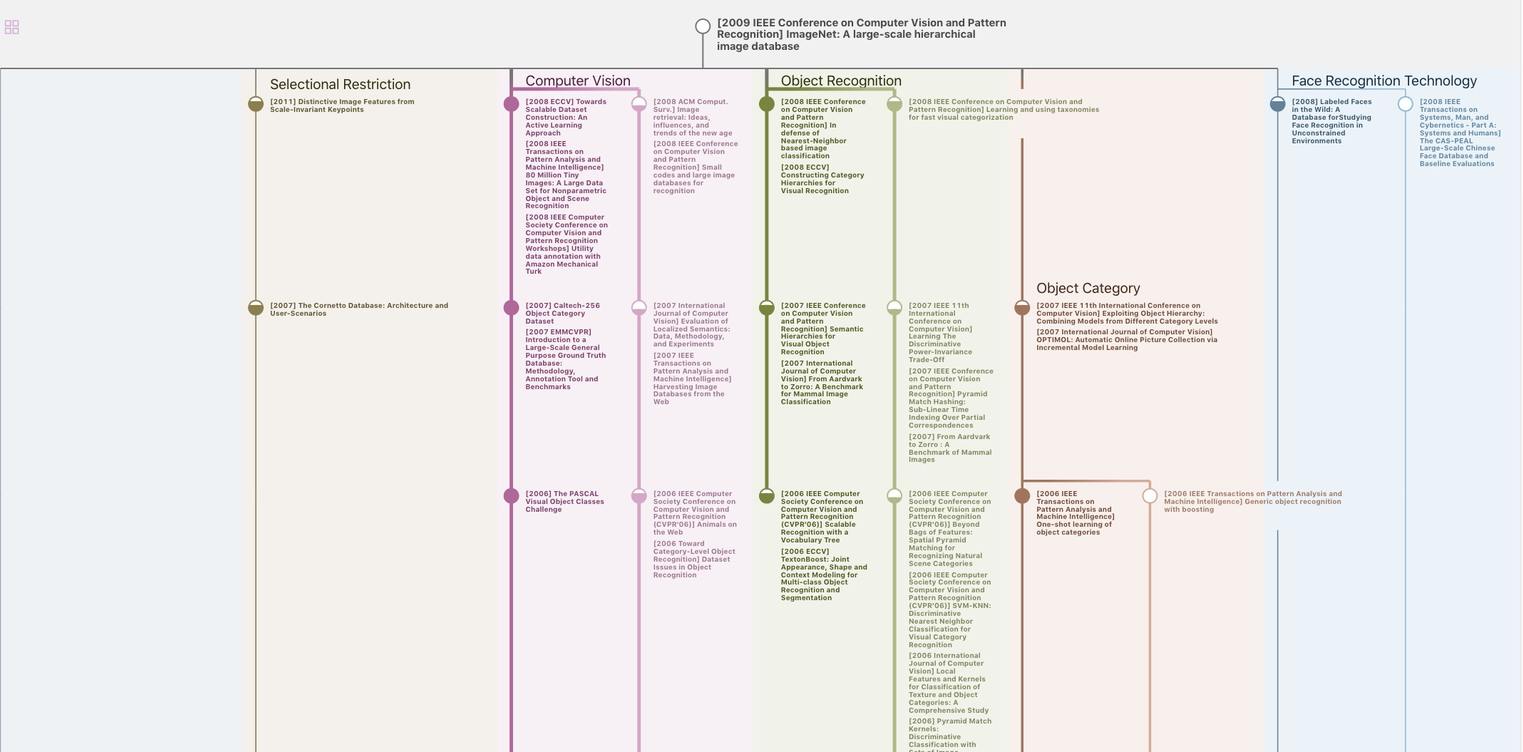
生成溯源树,研究论文发展脉络
Chat Paper
正在生成论文摘要