Accurate segmentation for different types of lung nodules on CT images using improved U-Net convolutional network
MEDICINE(2021)
摘要
Since lung nodules on computed tomography images can have different shapes, contours, textures or locations and may be attached to neighboring blood vessels or pleural surfaces, accurate segmentation is still challenging. In this study, we propose an accurate segmentation method based on an improved U-Net convolutional network for different types of lung nodules on computed tomography images. The first phase is to segment lung parenchyma and correct the lung contour by applying alpha-hull algorithm. The second phase is to extract image pairs of patches containing lung nodules in the center and the corresponding ground truth and build an improved U-Net network with introduction of batch normalization. A large number of experiments manifest that segmentation performance of Dice loss has superior results than mean square error and Binary_crossentropy loss. The alpha-hull algorithm and batch normalization can improve the segmentation performance effectively. Our best result for Dice similar coefficient (0.8623) is also more competitive than other state-of-the-art segmentation algorithms. In order to segment different types of lung nodules accurately, we propose an improved U-Net network, which can improve the segmentation accuracy effectively. Moreover, this work also has practical value in helping radiologists segment lung nodules and diagnose lung cancer.
更多查看译文
关键词
computed tomography (CT) image, contour correction, lung nodule, segmentation, U-Net
AI 理解论文
溯源树
样例
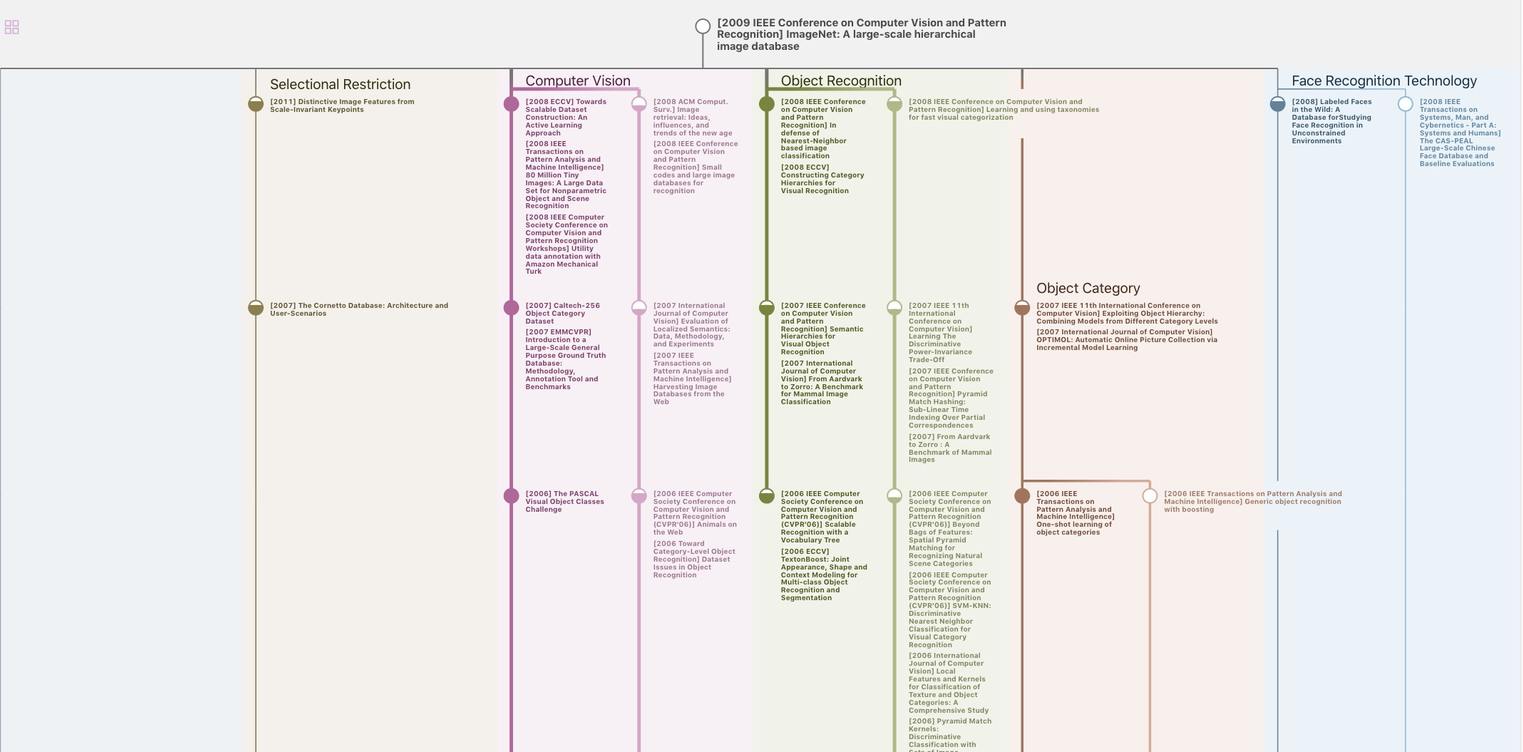
生成溯源树,研究论文发展脉络
Chat Paper
正在生成论文摘要