Physics-Informed Neural Networks for AC Optimal Power Flow
ELECTRIC POWER SYSTEMS RESEARCH(2022)
摘要
This paper introduces, for the first time to our knowledge, physics-informed neural networks to accurately estimate the AC-Optimal Power Flow (AC-OPF) result and delivers rigorous guarantees about their performance. Power system operators, along with several other actors, are increasingly using Optimal Power Flow (OPF) algorithms for a wide number of applications, including planning and real-time operations. However, in its original form, the AC OPF problem is often challenging to solve as it is non-linear and non-convex. Besides the large number of approximations and relaxations, recent efforts have also been focusing on Machine Learning approaches, especially neural networks. So far, however, these approaches have only partially considered the wide number of physical models available during training. And, more importantly, they have offered no guarantees about potential constraint violations of their output. Our approach (i) introduces a framework to incorporate AC power flow equations inside neural network training and (ii) integrates methods that rigorously determine and reduce the worst-case constraint violations across the entire input domain, while maintaining the optimality of the prediction. We demonstrate how physics-informed neural networks achieve higher accuracy and lower constraint violations than standard neural networks, and show how we can further reduce the worst-case violations for all neural networks.
更多查看译文
关键词
AC-OPF, Physics-informed neural network, Worst-case guarantees
AI 理解论文
溯源树
样例
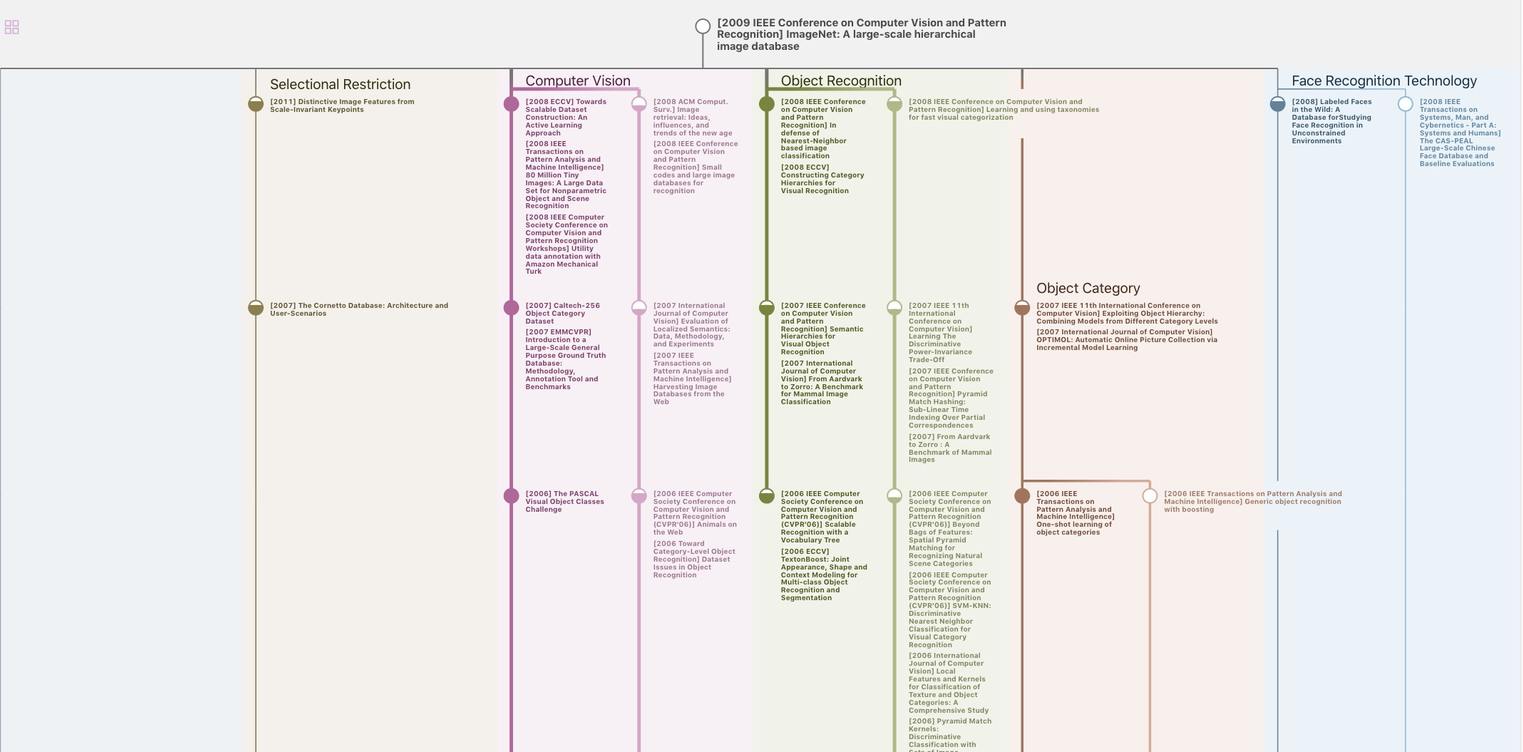
生成溯源树,研究论文发展脉络
Chat Paper
正在生成论文摘要