2D score-based estimation of heterogeneous treatment effects
arxiv(2023)
摘要
Statisticians show growing interest in estimating and analyzing heterogeneity in causal effects in observational studies. However, there usually exists a trade-off between accuracy and interpretability for developing a desirable estimator for treatment effects, especially in the case when there are a large number of features in estimation. To make efforts to address the issue, we propose a score-based framework for estimating the conditional average treatment effect (CATE) function in this article. The framework integrates two components: (i) leverage the joint use of propensity and prognostic scores in a matching algorithm to obtain a proxy of the heterogeneous treatment effects for each observation and (ii) utilize nonparametric regression trees to construct an estimator for the CATE function conditioning on the two scores. The method naturally stratifies treatment effects into subgroups over a 2d grid whose axis are the propensity and prognostic scores. We conduct benchmark experiments on multiple simulated data and demonstrate clear advantages of the proposed estimator over state-of-the-art methods. We also evaluate empirical performance in real-life settings, using two observational data from a clinical trial and a complex social survey, and interpret policy implications following the numerical results.
更多查看译文
关键词
heterogeneous treatment effects,2d,estimation,score-based
AI 理解论文
溯源树
样例
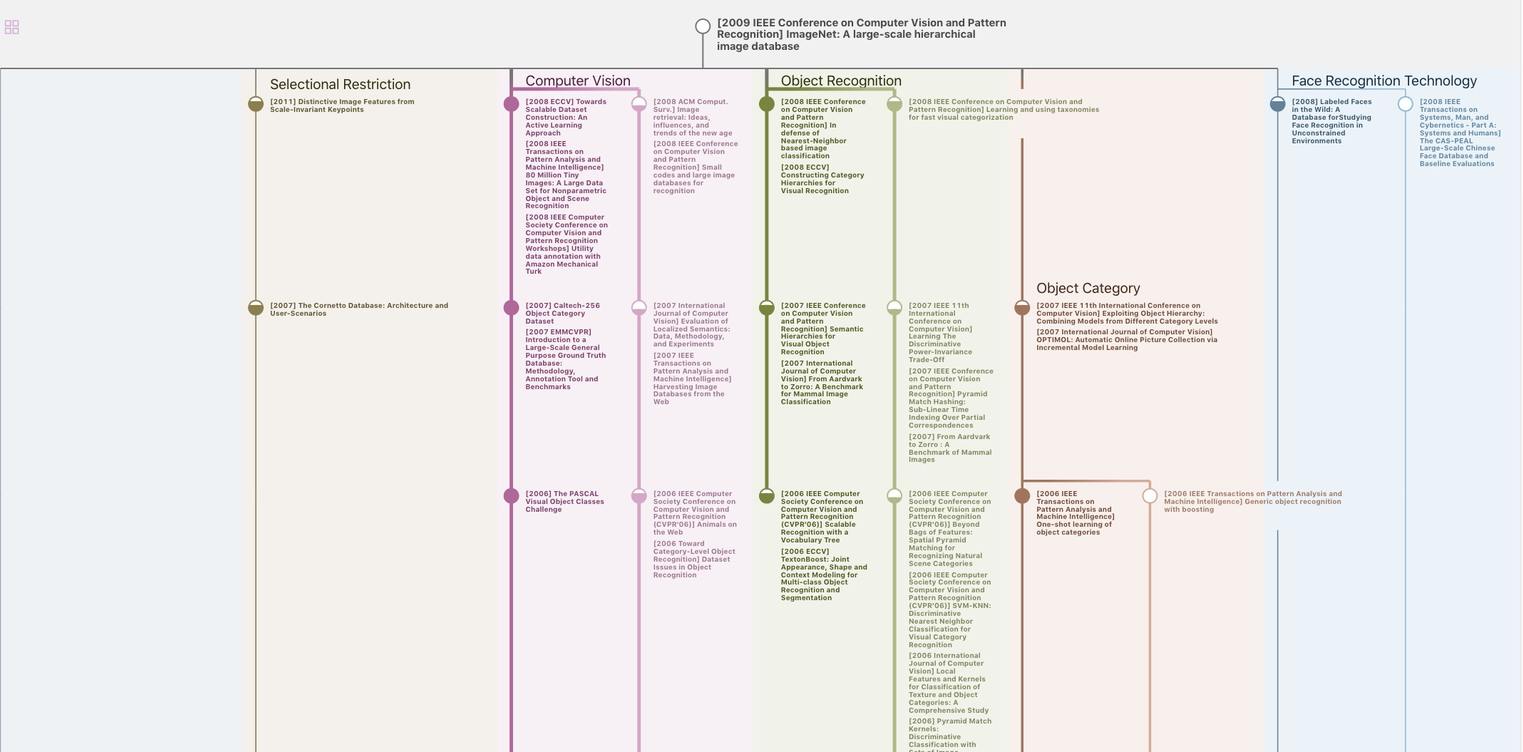
生成溯源树,研究论文发展脉络
Chat Paper
正在生成论文摘要