Bayesian optimization of multivariate genomic prediction models based on secondary traits for improved accuracy gains and phenotyping costs
Theoretical and Applied Genetics(2021)
摘要
Key message We propose a novel approach to the Bayesian optimization of multivariate genomic prediction models based on secondary traits to improve accuracy gains and phenotyping costs via efficient Pareto frontier estimation. Abstract Multivariate genomic prediction based on secondary traits, such as data from various omics technologies including high-throughput phenotyping (e.g., unmanned aerial vehicle-based remote sensing), has attracted much attention because it offers improved accuracy gains compared with genomic prediction based only on marker genotypes. Although there is a trade-off between accuracy gains and phenotyping costs of secondary traits, no attempt has been made to optimize these trade-offs. In this study, we propose a novel approach to optimize multivariate genomic prediction models for secondary traits measurable at early growth stages for improved accuracy gains and phenotyping costs. The proposed approach employs Bayesian optimization for efficient Pareto frontier estimation, representing the maximum accuracy at a given cost. The proposed approach successfully estimated the optimal secondary trait combinations across a range of costs while providing genomic predictions for only about 20 % of all possible combinations. The simulation results reflecting the characteristics of each scenario of the simulated target traits showed that the obtained optimal combinations were reasonable. Analysis of real-time target trait data showed that the proposed multivariate genomic prediction model had significantly superior accuracy compared to the univariate genomic prediction model.
更多查看译文
关键词
genomic prediction models,bayesian optimization,secondary traits,prediction models
AI 理解论文
溯源树
样例
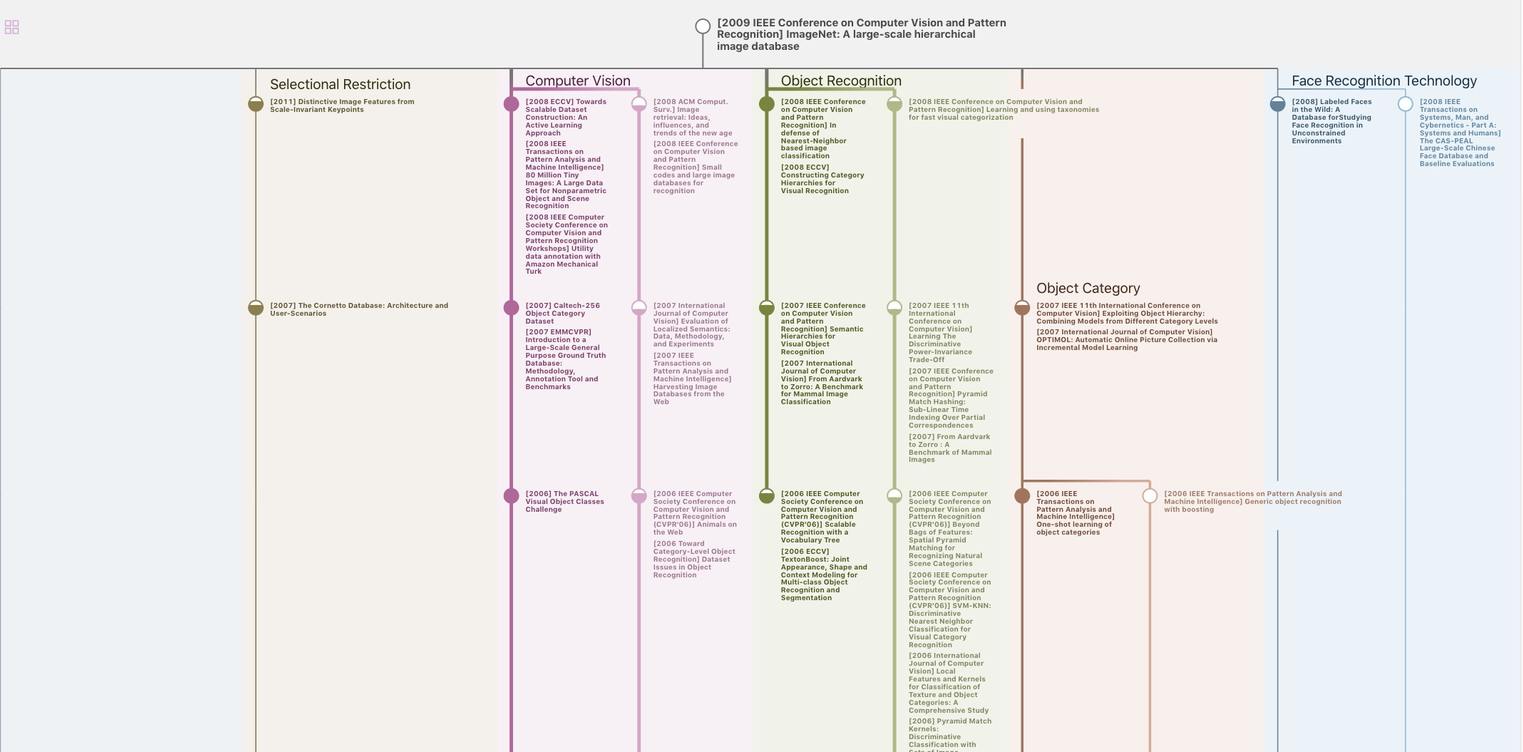
生成溯源树,研究论文发展脉络
Chat Paper
正在生成论文摘要