Real-Time Patient-Specific ECG Classification by 1D Self-Operational Neural Networks
IEEE TRANSACTIONS ON BIOMEDICAL ENGINEERING(2022)
摘要
Objective: Despitethe proliferation of numerous deep learning methods proposed for generic ECG classification and arrhythmia detection, compact systems with the real-time ability and high accuracy for classifying patient-specific ECG are still few. Particularly, the scarcity of patient-specific data poses an ultimate challenge to any classifier. Recently, compact 1D Convolutional Neural Networks (CNNs) have achieved the state-of-the-art performance level for the accurate classification of ventricular and supraventricular ectopic beats. However, several studies have demonstrated the fact that the learning performance of the conventional CNNs is limited because they are homogenous networks with a basic (linear) neuron model. In order to address this deficiency and further boost the patient-specific ECG classification performance, in this study, we propose 1D Self-organized Operational Neural Networks (1D Self-ONNs). Methods: Due to its self-organization capability, Self-ONNs have the utmost advantage and superiority over conventional ONNs where the prior operator search within the operator set library to find the best possible set of operators is entirely avoided. Results: Under AAMI recommendations and with minimal common training data used, over the entire MIT-BIH dataset 1D Self-ONNs have achieved 98% and 99.04% average accuracies, 76.6% and 93.7% average F1 scores on supra-ventricular and ventricular ectopic beat (VEB) classifications, respectively, which is the highest performance level ever reported. Conclusion: As the first study where 1D Self-ONNs are ever proposed for a classification task, our results over the MIT-BIH arrhythmia benchmark database demonstrate that 1D Self-ONNs can surpass 1D CNNs with a significant margin while having a similar computational complexity.
更多查看译文
关键词
Neurons,Electrocardiography,Training,Real-time systems,Kernel,Complexity theory,Task analysis,Patient-specific ECG classification,Operational Neural Networks,real-time heart monitoring,generative neuron
AI 理解论文
溯源树
样例
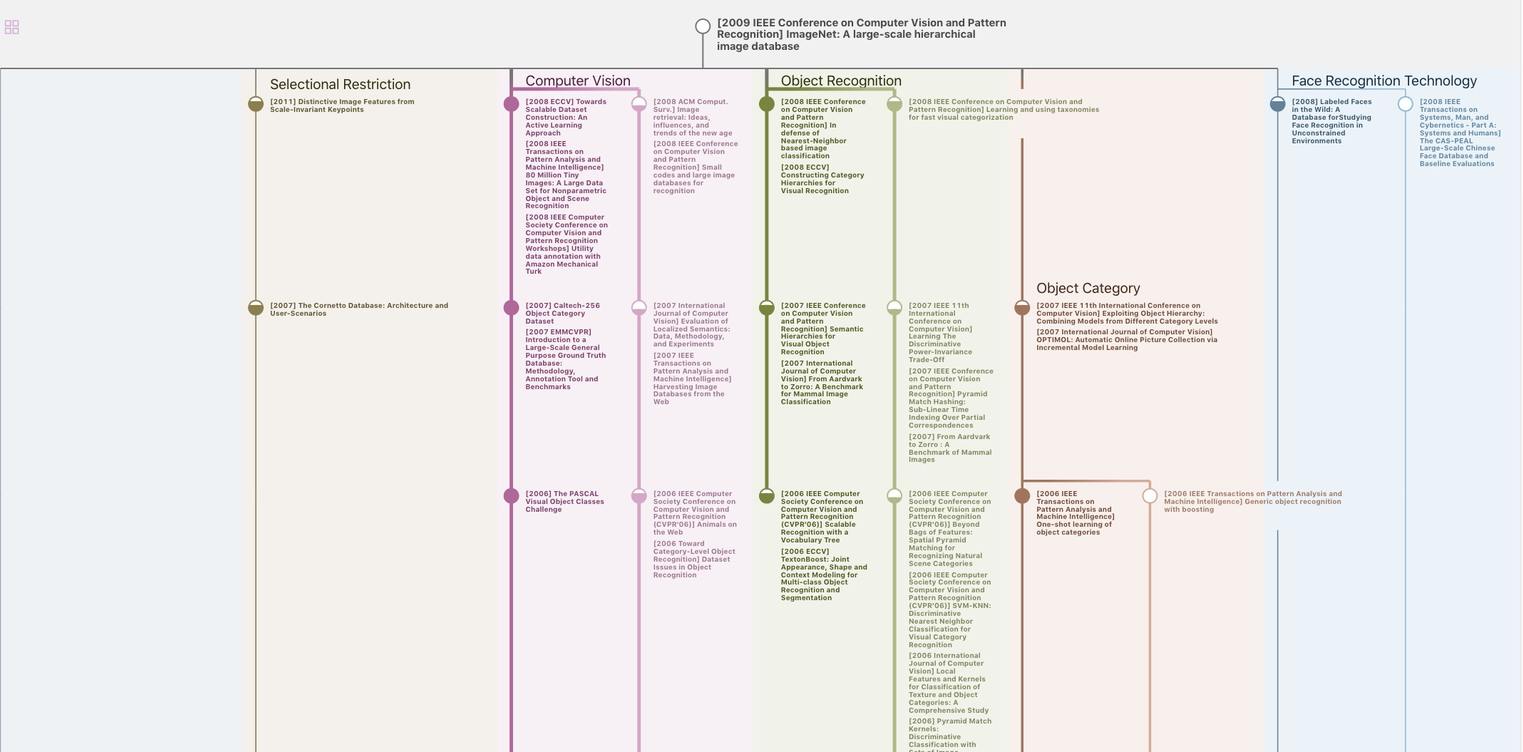
生成溯源树,研究论文发展脉络
Chat Paper
正在生成论文摘要