Self-Supervised Generative Style Transfer for One-Shot Medical Image Segmentation
2022 IEEE WINTER CONFERENCE ON APPLICATIONS OF COMPUTER VISION (WACV 2022)(2022)
摘要
In medical image segmentation, supervised deep networks' success comes at the cost of requiring abundant labeled data. While asking domain experts to annotate only one or a few of the cohort's images is feasible, annotating all available images is impractical. This issue is further exacerbated when pre-trained deep networks are exposed to a new image dataset from an unfamiliar distribution. Using available open-source data for ad-hoc transfer learning or hand-tuned techniques for data augmentation only provides suboptimal solutions. Motivated by atlas-based segmentation, we propose a novel volumetric self-supervised learning for data augmentation capable of synthesizing volumetric image-segmentation pairs via learning transformations from a single labeled atlas to the unlabeled data. Our work's central tenet benefits from a combined view of one-shot generative learning and the proposed self-supervised training strategy that cluster unlabeled volumetric images with similar styles together. Unlike previous methods, our method does not require input volumes at inference time to synthesize new images. Instead, it can generate diversified volumetric image-segmentation pairs from a prior distribution given a single or multi-site dataset. Augmented data generated by our method used to train the segmentation network provide significant improvements over state-of-the-art deep one-shot learning methods on the task of brain MRI segmentation. Ablation studies fisrther exemplified that the proposed appearance model and joint training are crucial to synthesize realistic examples compared to existing medical registration methods. The code, data, and models are available at https://github.com/devavratTomar/SST/.
更多查看译文
关键词
Medical Imaging/Imaging for Bioinformatics/Biological and Cell Microscopy Segmentation,Grouping and Shape,Transfer,Few-shot,Semi- and Un- supervised Learning
AI 理解论文
溯源树
样例
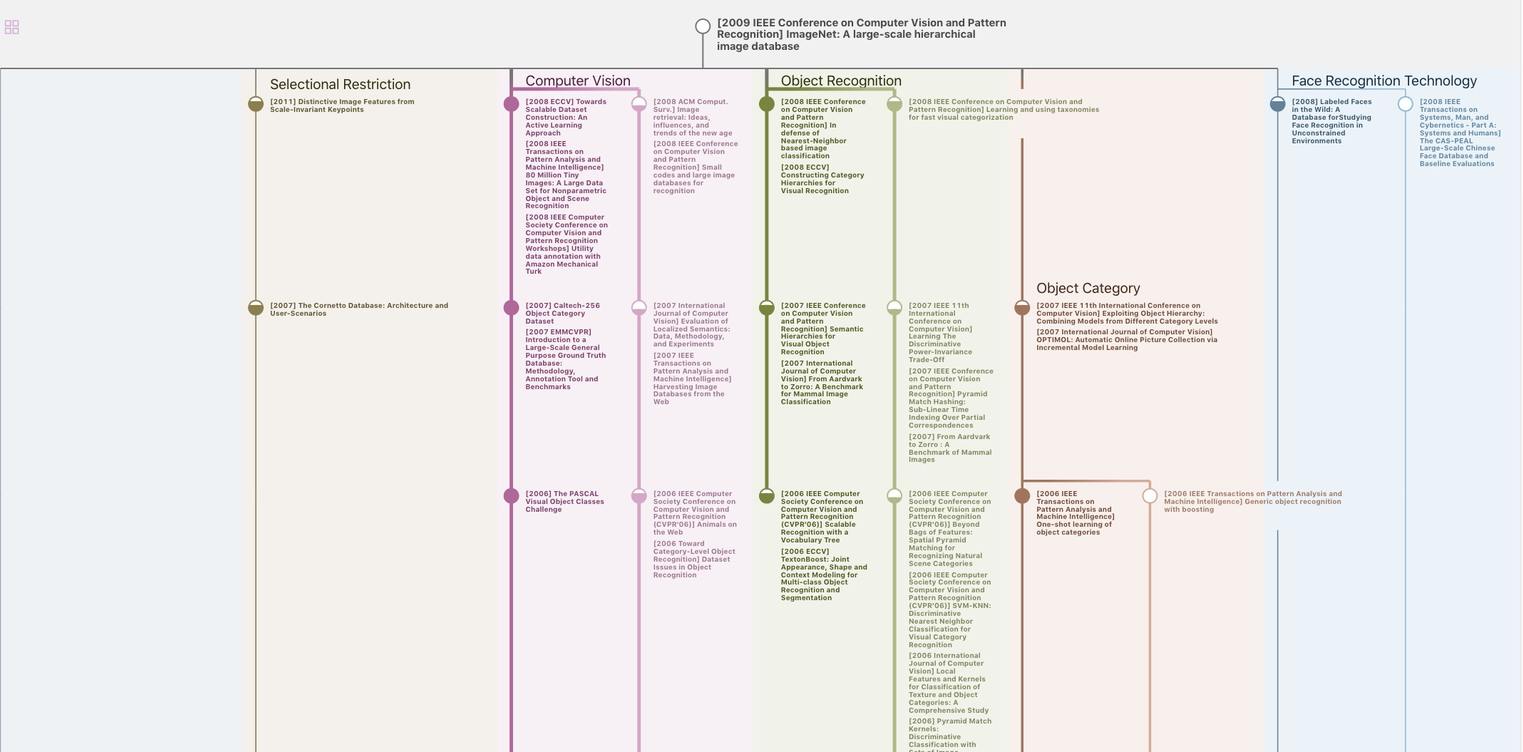
生成溯源树,研究论文发展脉络
Chat Paper
正在生成论文摘要