Extended Dynamic Mode Decomposition With Dictionary Learning Using Neural Ordinary Differential Equations
IEICE NONLINEAR THEORY AND ITS APPLICATIONS(2021)
摘要
Nonlinear phenomena can be analyzed via linear techniques using operator-theoretic approaches. Data-driven method called the extended dynamic mode decomposition (EDMD) and its variants, which approximate the Koopman operator associated with the nonlinear phenomena, have been rapidly developing by incorporating machine learning methods. Neural ordinary differential equations (NODEs), which are a neural network equipped with a continuum of layers, and have high parameter and memory efficiencies, have been proposed. In this paper, we propose an algorithm to perform EDMD using NODEs. NODEs are used to find a parameter-efficient dictionary which provides a good finite-dimensional approximation of the Koopman operator. We show the superiority of the parameter efficiency of the proposed method through numerical experiments.
更多查看译文
关键词
time series analysis, Koopman operator, extended dynamic mode decomposition, machine learning, dictionary learning, neural ordinary differential equations
AI 理解论文
溯源树
样例
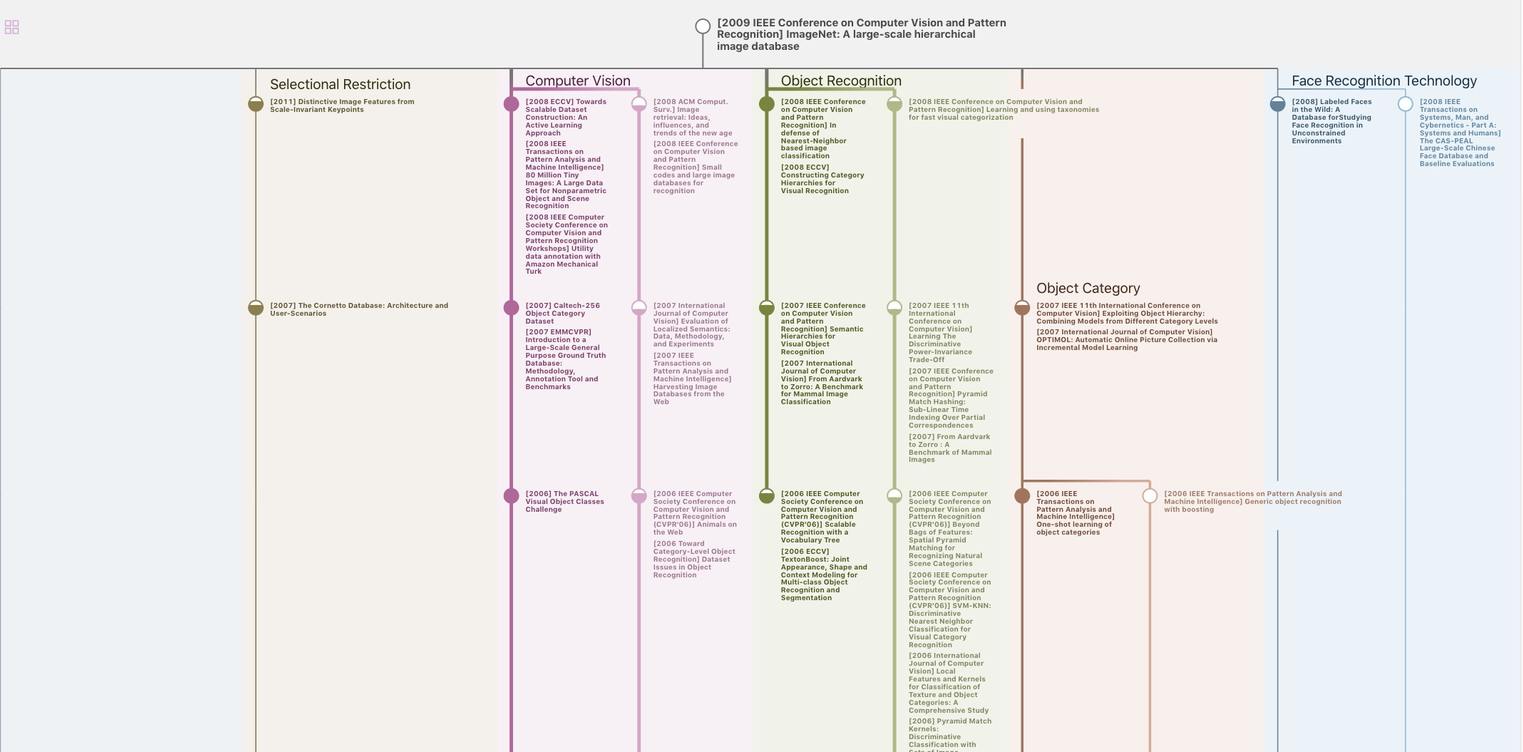
生成溯源树,研究论文发展脉络
Chat Paper
正在生成论文摘要