Network Friendly Recommendations: Optimizing for Long Viewing Sessions
IEEE Transactions on Mobile Computing(2023)
摘要
Caching algorithms try to predict content popularity, and place the content closer to the users. Additionally, nowadays requests are increasingly driven by recommendation systems (RS). These important trends, point to the following:
make RSs favor locally cached content
, this way operators reduce network costs, and users get better streaming rates. Nevertheless, this process should preserve the quality of the recommendations (QoR). In this work, we propose a Markov Chain model for a stochastic, recommendation-driven
sequence
of requests, and formulate the problem of selecting high quality recommendations that minimize the network cost
in the long run
. While the original optimization problem is non-convex, it can be convexified through a series of transformations. Moreover, we extend our framework for users who show preference in some positions of the recommendations’ list. To our best knowledge, this is the first work to provide an optimal polynomial-time algorithm for these problems. Finally, testing our algorithms on real datasets suggests significant potential, e.g.,
$2\times$
improvement compared to baseline recommendations, and 80% compared to a greedy network-friendly-RS (which optimizes the cost for I.I.D. requests), while preserving at least 90% of the original QoR. Finally, we show that taking position preference into account leads to additional performance gains.
更多查看译文
关键词
Recommendation systems,caching,modeling,optimization
AI 理解论文
溯源树
样例
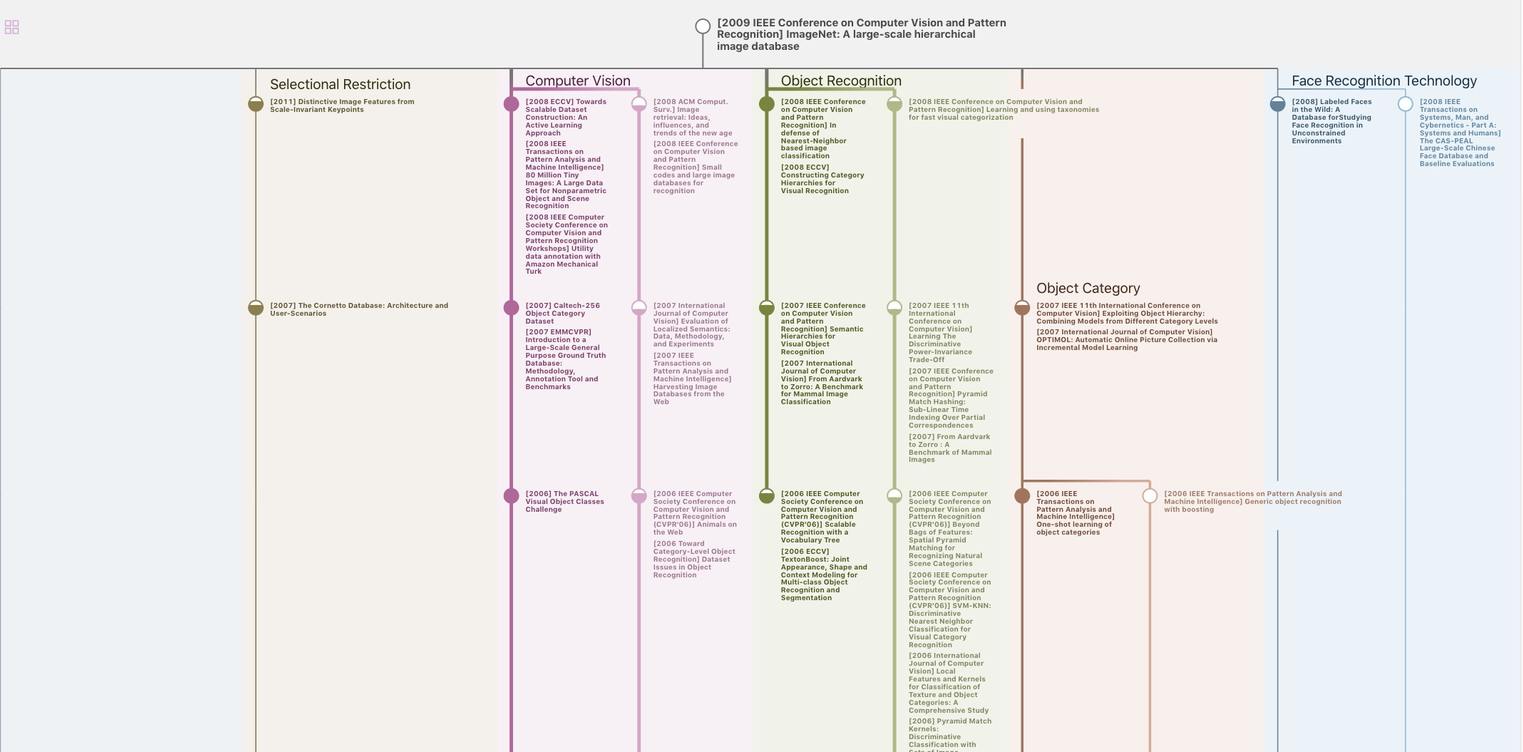
生成溯源树,研究论文发展脉络
Chat Paper
正在生成论文摘要