Development And Validation Of Machine-Learning Clear-Sky Detection Method Using 1-Min Irradiance Data And Sky Imagers At A Polluted Suburban Site, Xianghe
REMOTE SENSING(2021)
摘要
Clear-sky detection (CSD) is of critical importance in solar energy applications and surface radiative budget studies. Existing CSD methods are not sufficiently validated due to the lack of high-temporal resolution and long-term CSD ground observations, especially at polluted sites. Using five-year high resolution ground-based solar radiation data and visual inspected Total Sky Imager (TSI) measurements at polluted Xianghe, a suburban site, this study validated 17 existing CSD methods and developed a new CSD model based on a machine-learning algorithm (Random Forest: RF). The propagation of systematic errors from input data to the calculated global horizontal irradiance (GHI) is confirmed with Mean Absolute Error (MAE) increased by 99.7% (from 20.00 to 39.93 W center dot m(-2)). Through qualitative evaluation, the novel Bright-Sun method outperforms the other traditional CSD methods at Xianghe site, with high accuracy score 0.73 and 0.92 under clear and cloudy conditions, respectively. The RF CSD model developed by one-year irradiance and TSI data shows more robust performance, with clear/cloudy-sky accuracy score of 0.78/0.88. Overall, the Bright-Sun and RF CSD models perform satisfactorily at heavy polluted sites. Further analysis shows the RF CSD model built with only GHI-related parameters can still achieve a mean accuracy score of 0.81, which indicates RF CSD models have the potential in dealing with sites only providing GHI observations.
更多查看译文
关键词
clear sky detection, surface irradiance, random forest, total sky imager, bright-sun
AI 理解论文
溯源树
样例
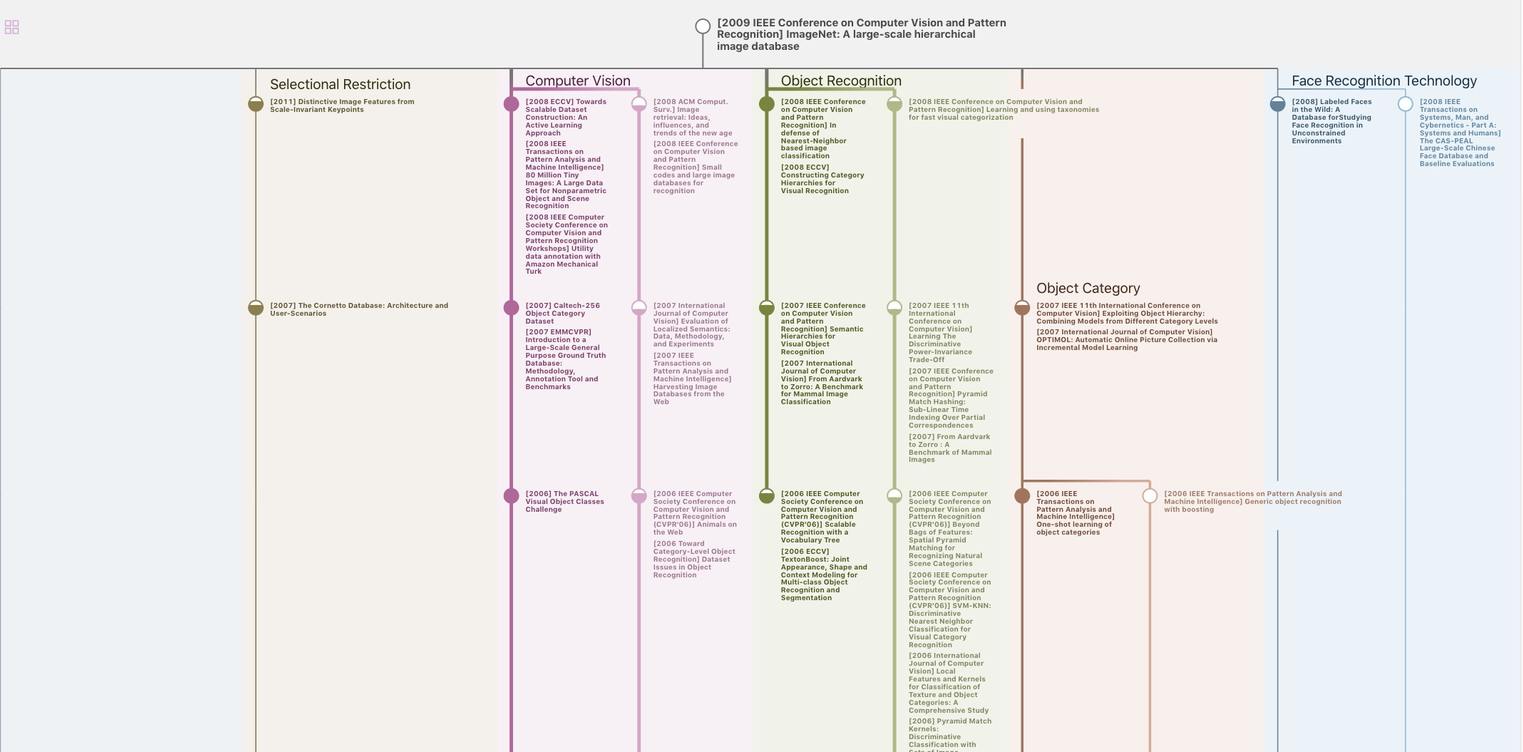
生成溯源树,研究论文发展脉络
Chat Paper
正在生成论文摘要