SMATE: Semi-Supervised Spatio-Temporal Representation Learning on Multivariate Time Series
2021 21ST IEEE INTERNATIONAL CONFERENCE ON DATA MINING (ICDM 2021)(2021)
摘要
Learning from Multivariate Time Series (MTS) has attracted widespread attention in recent years. In particular, label shortage is a real challenge for the classification task on MTS, considering its complex dimensional and sequential data structure. Unlike self-training and positive unlabeled learning that rely on distance-based classifiers, in this paper, we propose SMATE, a novel semi-supervised model for learning the interpretable Spatio-Temporal representation from weakly labeled MTS. We validate empirically the learned representation on 30 public datasets from the UEA MTS archive. We compare it with 13 state-of-the-art baseline methods for fully supervised tasks and four baselines for semi-supervised tasks. The results show the reliability and efficiency of our proposed method.
更多查看译文
关键词
Neural Networks, Multivariate Time Series, Semi-supervised Learning, Representation Learning
AI 理解论文
溯源树
样例
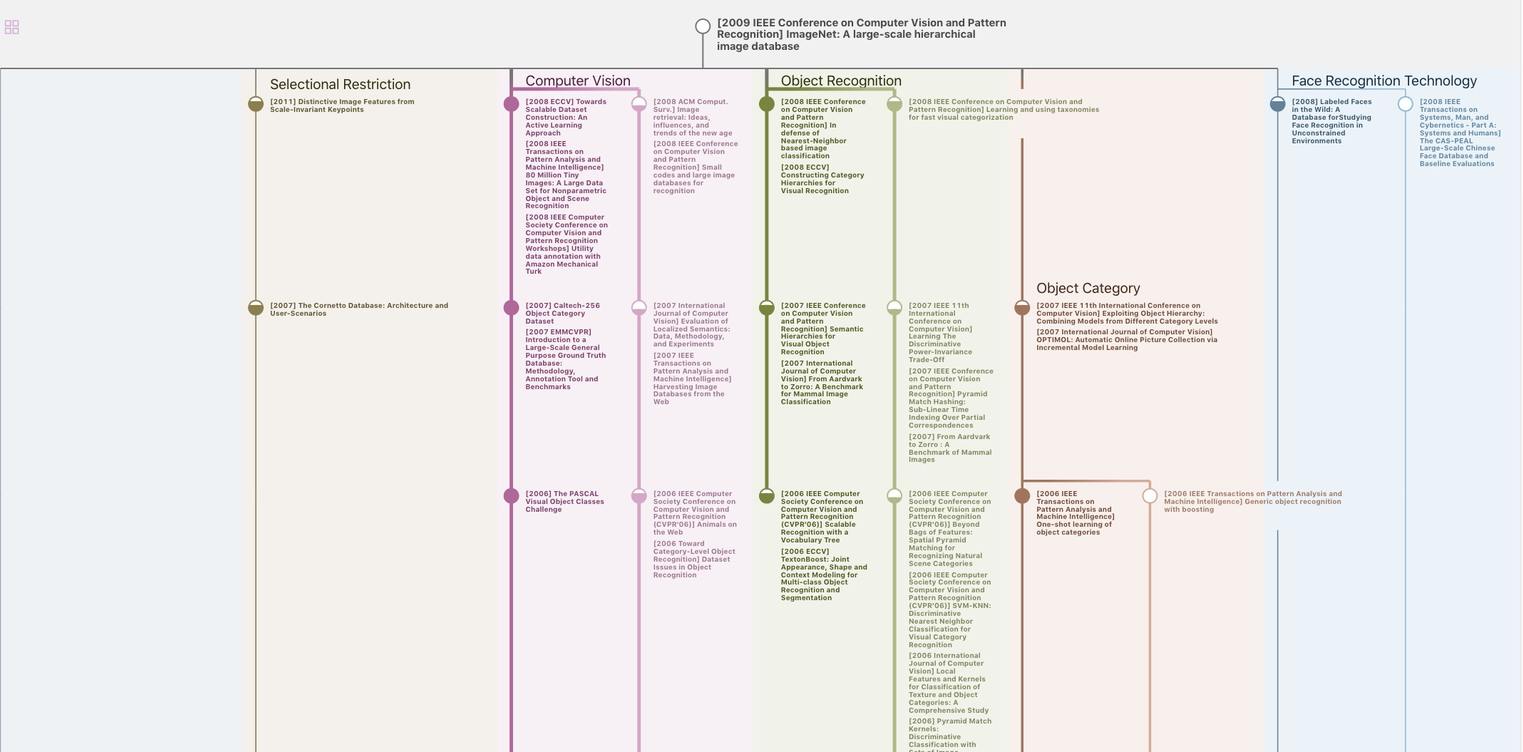
生成溯源树,研究论文发展脉络
Chat Paper
正在生成论文摘要