Visual cluster separation using high-dimensional sharpened dimensionality reduction
INFORMATION VISUALIZATION(2022)
摘要
Applying dimensionality reduction (DR) to large, high-dimensional data sets can be challenging when distinguishing the underlying high-dimensional data clusters in a 2D projection for exploratory analysis. We address this problem by first sharpening the clusters in the original high-dimensional data prior to the DR step using Local Gradient Clustering (LGC). We then project the sharpened data from the high-dimensional space to 2D by a user-selected DR method. The sharpening step aids this method to preserve cluster separation in the resulting 2D projection. With our method, end-users can label each distinct cluster to further analyze an otherwise unlabeled data set. Our "High-Dimensional Sharpened DR" (HD-SDR) method, tested on both synthetic and real-world data sets, is favorable to DR methods with poor cluster separation and yields a better visual cluster separation than these DR methods with no sharpening. Our method achieves good quality (measured by quality metrics) and scales computationally well with large high-dimensional data. To illustrate its concrete applications, we further apply HD-SDR on a recent astronomical catalog.
更多查看译文
关键词
High-dimensional data visualization, dimensionality reduction, clustering, astronomy
AI 理解论文
溯源树
样例
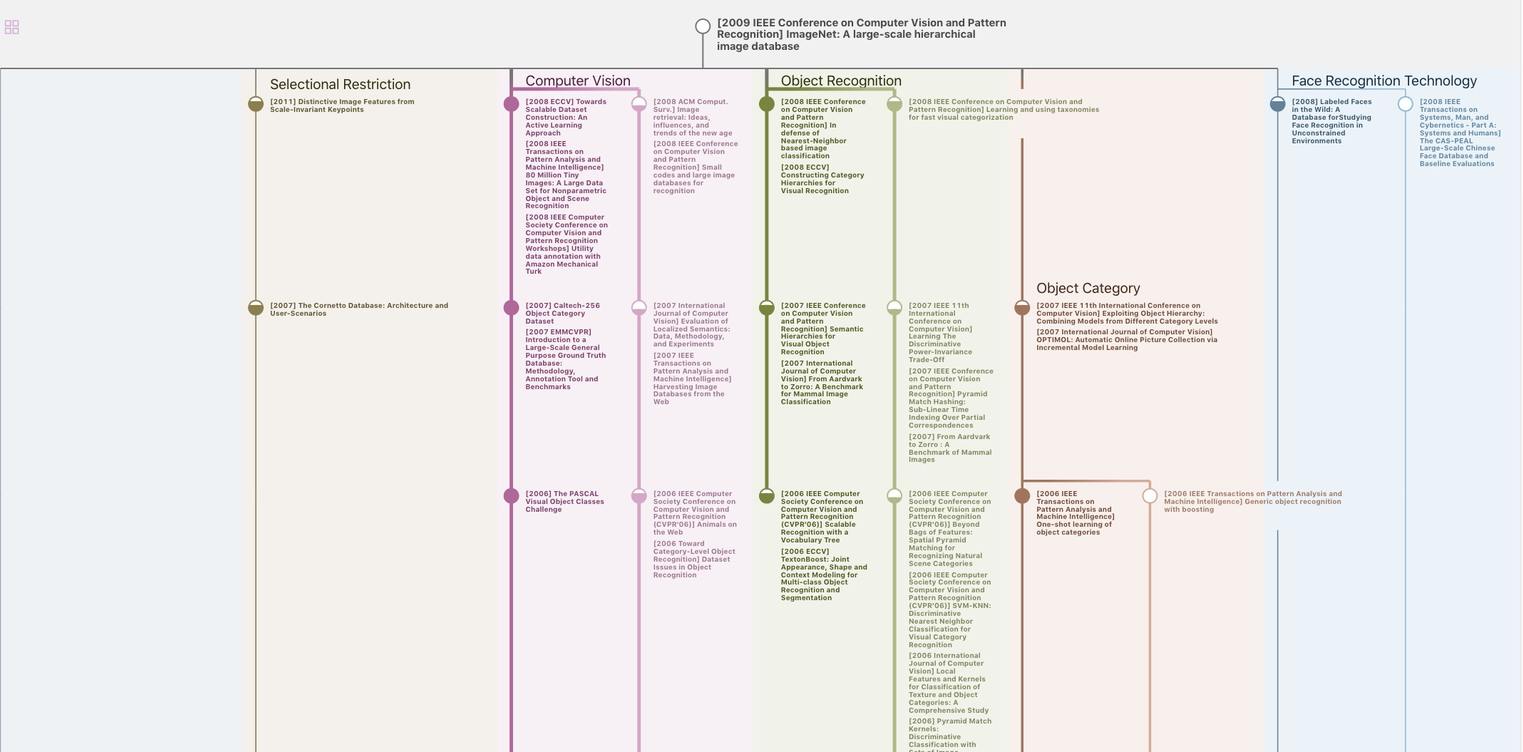
生成溯源树,研究论文发展脉络
Chat Paper
正在生成论文摘要