Regression-based estimation of heterogeneous treatment effects when extending inferences from a randomized trial to a target population
European Journal of Epidemiology(2023)
摘要
Most work on extending (generalizing or transporting) inferences from a randomized trial to a target population has focused on estimating average treatment effects (i.e., averaged over the target population’s covariate distribution). Yet, in the presence of strong effect modification by baseline covariates, the average treatment effect in the target population may be less relevant for guiding treatment decisions. Instead, the conditional average treatment effect (CATE) as a function of key effect modifiers may be a more useful estimand. Recent work on estimating target population CATEs using baseline covariate, treatment, and outcome data from the trial and covariate data from the target population only allows for the examination of heterogeneity over distinct subgroups. We describe flexible pseudo-outcome regression modeling methods for estimating target population CATEs conditional on discrete or continuous baseline covariates when the trial is embedded in a sample from the target population (i.e., in nested trial designs). We construct pointwise confidence intervals for the CATE at a specific value of the effect modifiers and uniform confidence bands for the CATE function. Last, we illustrate the methods using data from the Coronary Artery Surgery Study (CASS) to estimate CATEs given history of myocardial infarction and baseline ejection fraction value in the target population of all trial-eligible patients with stable ischemic heart disease.
更多查看译文
关键词
heterogeneous treatment effects,inferences,estimation,trial,regression-based
AI 理解论文
溯源树
样例
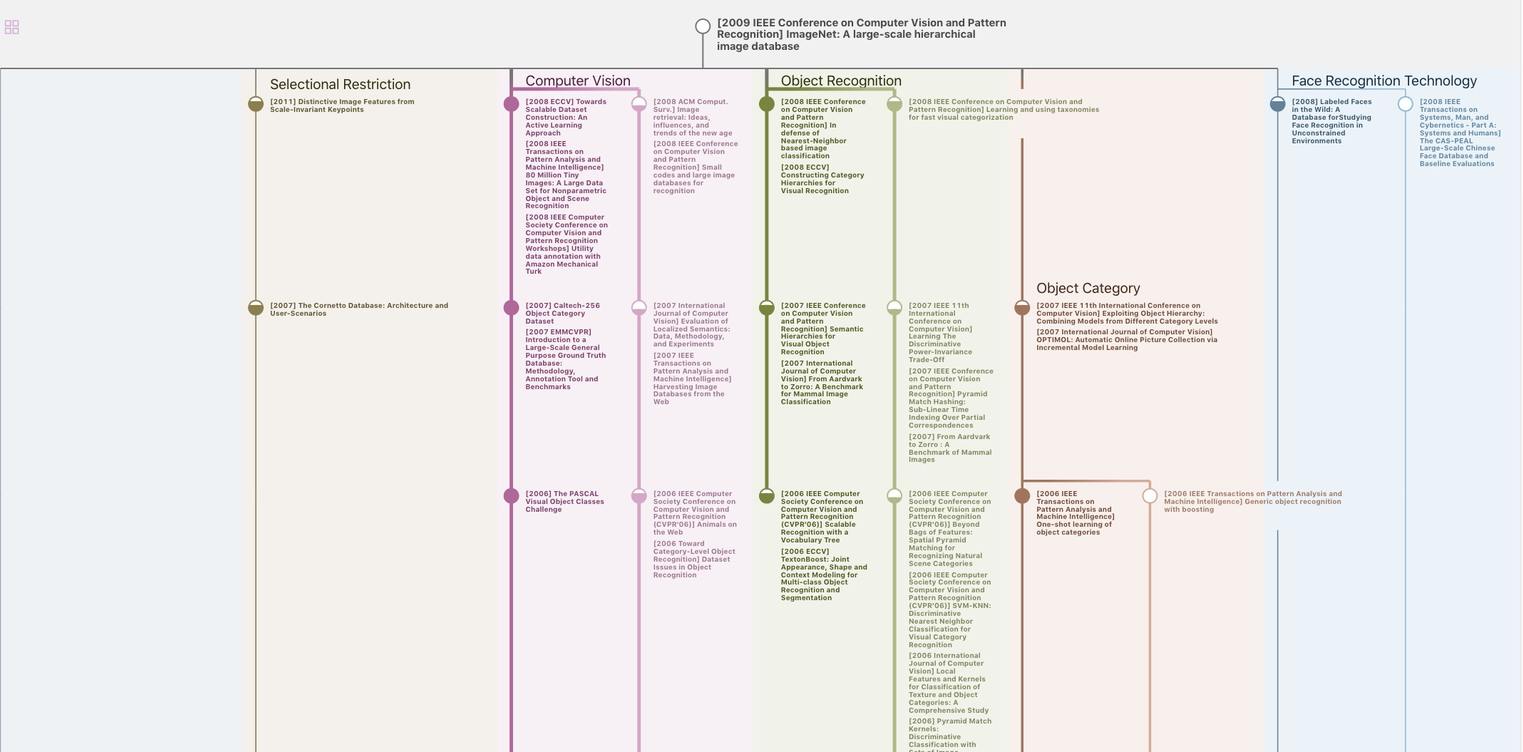
生成溯源树,研究论文发展脉络
Chat Paper
正在生成论文摘要