Trajectory Planning With Deep Reinforcement Learning in High-Level Action Spaces
arxiv(2023)
摘要
This article presents a technique for trajectory planning based on parameterized high-level actions. These high-level actions are subtrajectories that have variable shape and duration. The use of high-level actions can improve the performance of guidance algorithms. Specifically, we show how the use of high-level actions improves the performance of guidance policies that are generated via reinforcement learning (RL). RL has shown great promise for solving complex control, guidance, and coordination problems but can still suffer from long training times and poor performance. This work shows how the use of high-level actions reduces the required number of training steps and increases the path performance of an RL-trained guidance policy. We demonstrate the method on a space-shuttle guidance example. We show the proposed method increases the path performance (latitude range) by 18% compared with a baseline RL implementation. Similarly, we show the proposed method achieves steady state during training with approximately 75% fewer training steps. We also show how the guidance policy enables effective performance in an obstacle field. Finally, this article develops a loss function term for policy-gradient-based deep RL, which is analogous to an antiwindup mechanism in feedback control. We demonstrate that the inclusion of this term in the underlying optimization increases the average policy return in our numerical example.
更多查看译文
关键词
deep reinforcement learning,planning,action spaces,trajectory,high-level
AI 理解论文
溯源树
样例
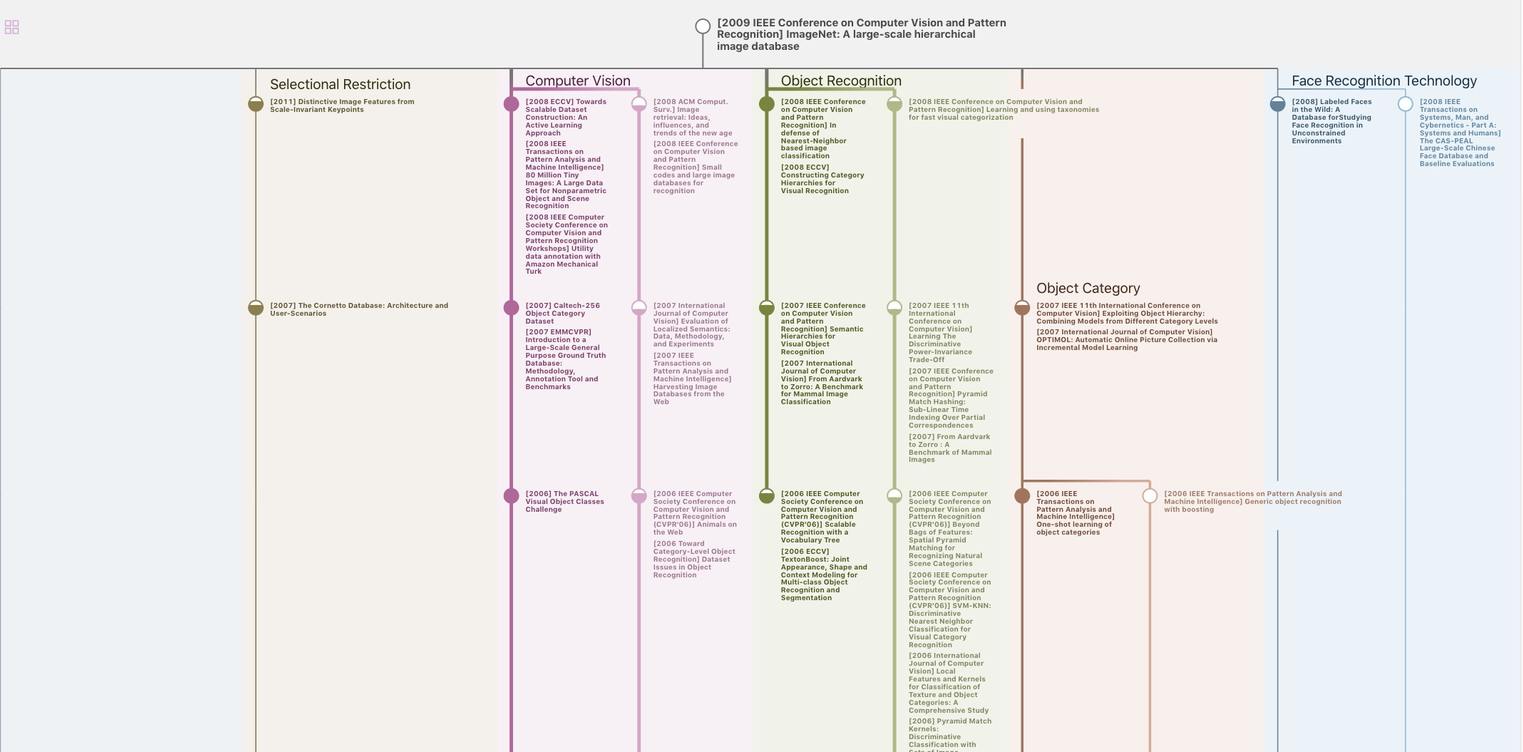
生成溯源树,研究论文发展脉络
Chat Paper
正在生成论文摘要