LiterallyWikidata - A Benchmark for Knowledge Graph Completion Using Literals
SEMANTIC WEB - ISWC 2021(2021)
摘要
In order to transform a Knowledge Graph (KG) into a low dimensional vector space, it is beneficial to preserve as much semantics as possible from the different components of the KG. Hence, some link prediction approaches have been proposed so far which leverage literals in addition to the commonly used links between entities. However, the procedures followed to create the existing datasets do not pay attention to literals. Therefore, this study presents a set of KG completion benchmark datasets extracted from Wikidata and Wikipedia, named LiterallyWikidata. It has been prepared with the main focus on providing benchmark datasets for multimodal KG Embedding (KGE) models, specifically for models using numeric and/or text literals. Hence, the benchmark is novel as compared to the existing datasets in terms of properly handling literals for those multimodal KGE models. LiterallyWikidata contains three datasets which vary both in size and structure. Benchmarking experiments on the task of link prediction have been conducted on LiterallyWikidata with extensively tuned unimodal/multimodal KGE models. The datasets are available at https://doi.org/10.5281/zenodo.4701190.
更多查看译文
关键词
Knowledge graph completion, Knowledge graph embedding, Link prediction, Literals, Benchmark dataset
AI 理解论文
溯源树
样例
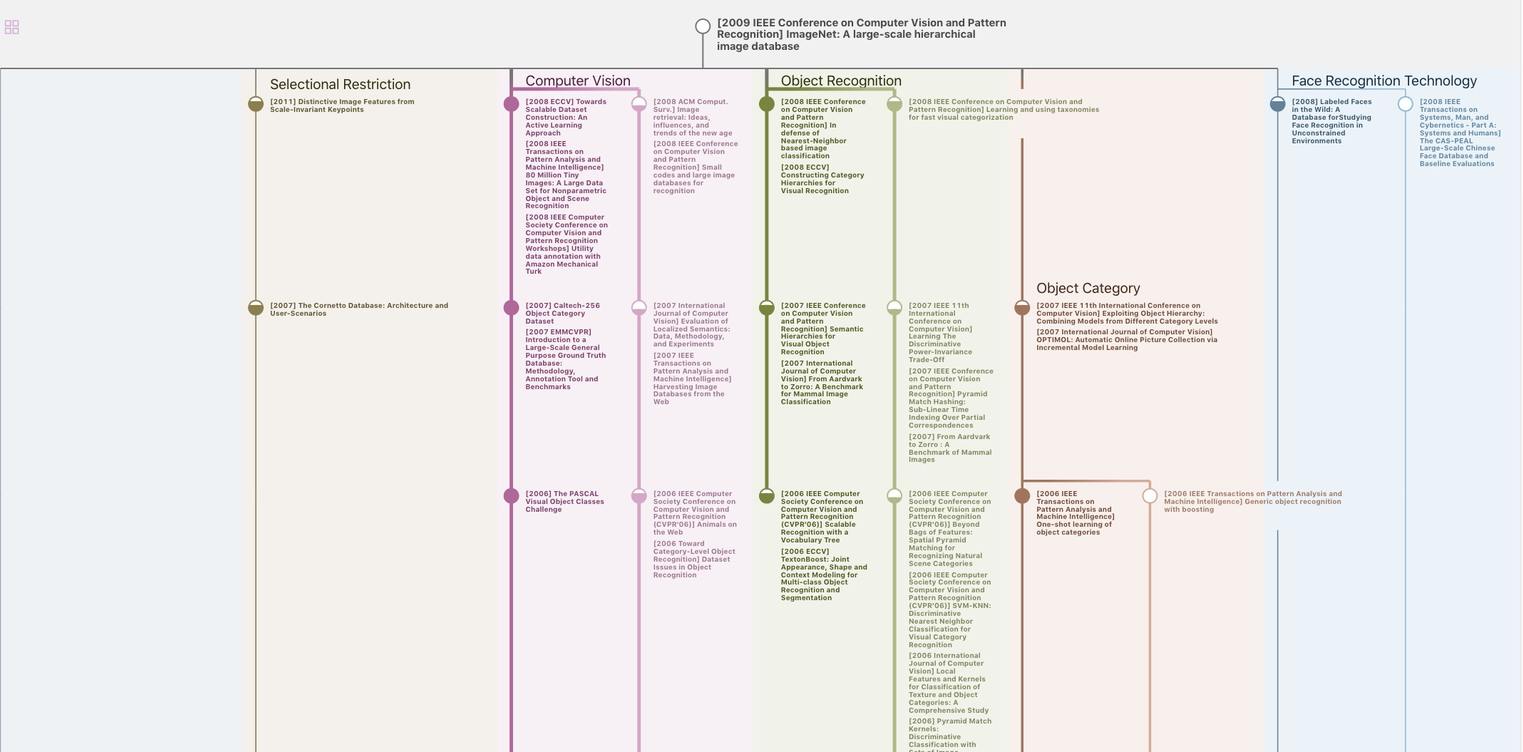
生成溯源树,研究论文发展脉络
Chat Paper
正在生成论文摘要