AROA - Attention Refinement One-Stage Anchor-Free Detector for Objects in Remote Sensing Imagery.
ICIG(2021)
摘要
Object detection in remote sensing images is a typical computer vision application, which has broad requirements in practice. Recently, attention mechanisms have been widely utilized in a diverse range of visual tasks such as object detection and semantic segmentation. Aimed at the characteristics of remote sensing objects such as rotation variations and inter-class similarity, a question we ask is, what kind of attention mechanism do we really need? In this article, we propose a novel attention refinement one-stage anchor-free object detector (AROA) that leverages attention mechanisms to refine the performance of remote sensing object detection in a one-stage anchor-free network framework. Specifically, we first design an asymmetric spatial self-attention (A S 2 A) mechanism to capture rich long-range spatial contexts and eliminate the rotate distortion. Then, to solve the issue of inter-class similarity and boost the multiclass identification capability, we propose a channel attention mechanism, named chain-connected channel attention ( C 3 A), which connects the adjacent attention blocks like a chain and dramatically mines the channel relationships. In addition, we also introduce an IoU-wise module (IM) to strengthen the correlation between localization and classification branches and filter out the detected boxes with low positioning quality. Extensive experimental results on the DOTA and NWPU VHR-10 datasets demonstrate the effectiveness of the proposed AROA.
更多查看译文
关键词
Object detection, Remote sensing images, Attention mechanisms, Anchor-free, One-stage
AI 理解论文
溯源树
样例
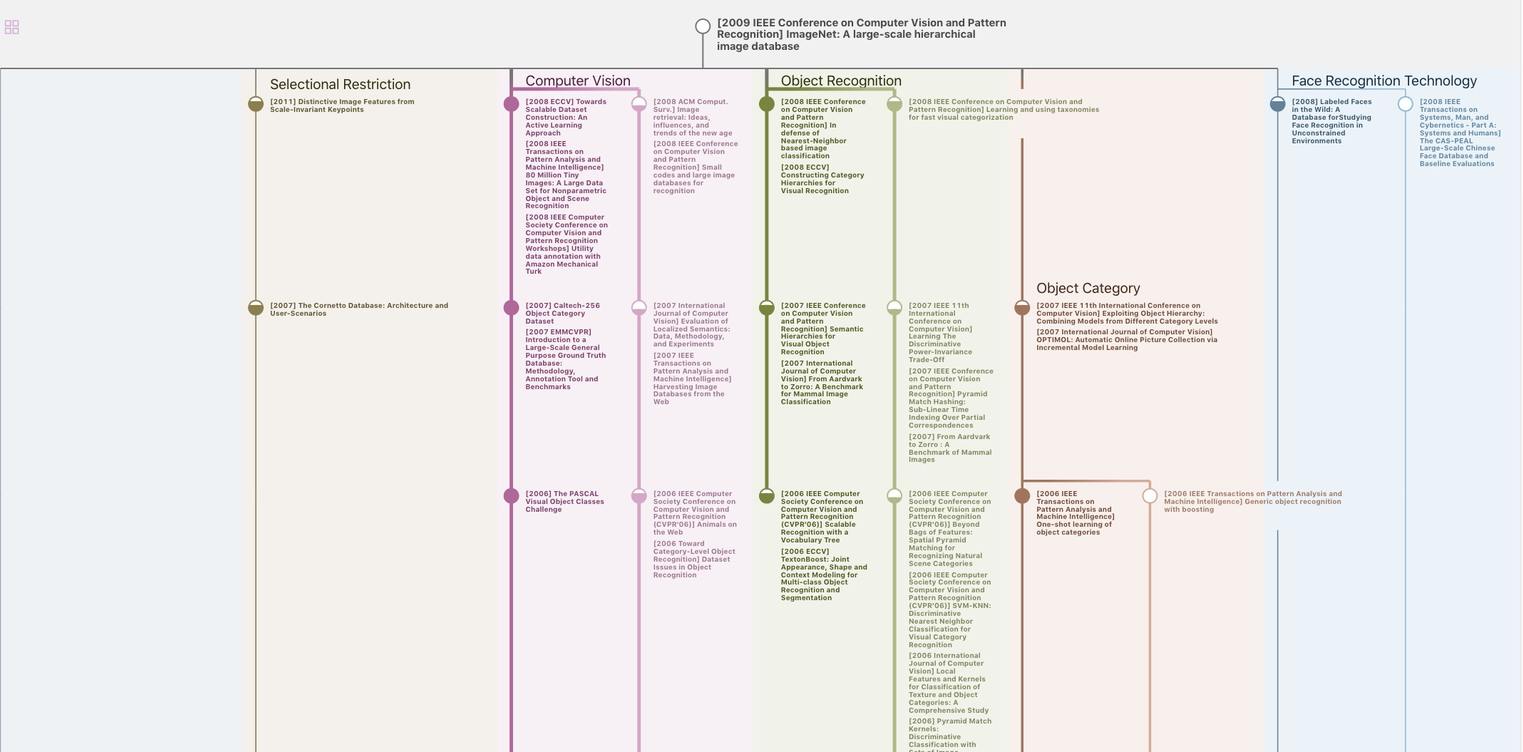
生成溯源树,研究论文发展脉络
Chat Paper
正在生成论文摘要