HERMAS: A Human Mobility Embedding Framework with Large-scale Cellular Signaling Data.
Proc. ACM Interact. Mob. Wearable Ubiquitous Technol.(2021)
摘要
Efficient representations for spatio-temporal cellular Signaling Data (SD) are essential for many human mobility applications. Traditional representation methods are mainly designed for GPS data with high spatio-temporal continuity, and thus will suffer from poor embedding performance due to the unique Ping Pong Effect in SD. To address this issue, we explore the opportunity offered by a large number of human mobility traces and mine the inherent neighboring tower connection patterns. More specifically, we design HERMAS, a novel representation learning framework for large-scale cellular SD with three steps: (1) extract rich context information in each trajectory, adding neighboring tower information as extra knowledge in each mobility observation; (2) design a sequence encoding model to aggregate the embedding of each observation; (3) obtain the embedding for a trajectory. We evaluate the performance of HERMAS based on two human mobility applications, i.e. trajectory similarity measurement and user profiling. We conduct evaluations based on a 30-day SD dataset with 130,612 users and 2,369,267 moving trajectories. Experimental results show that (1) for the trajectory similarity measurement application, HERMAS improves the Hitting Rate (HR@10) from 15.2% to 39.2%; (2) for the user profiling application, HERMAS improves the F1-score for around 9%. More importantly, HERMAS significantly improves the computation efficiency by over 30x.
更多查看译文
关键词
Signaling Data, Representation Learning, Trajectory Embedding, Regular Pattern Exploration
AI 理解论文
溯源树
样例
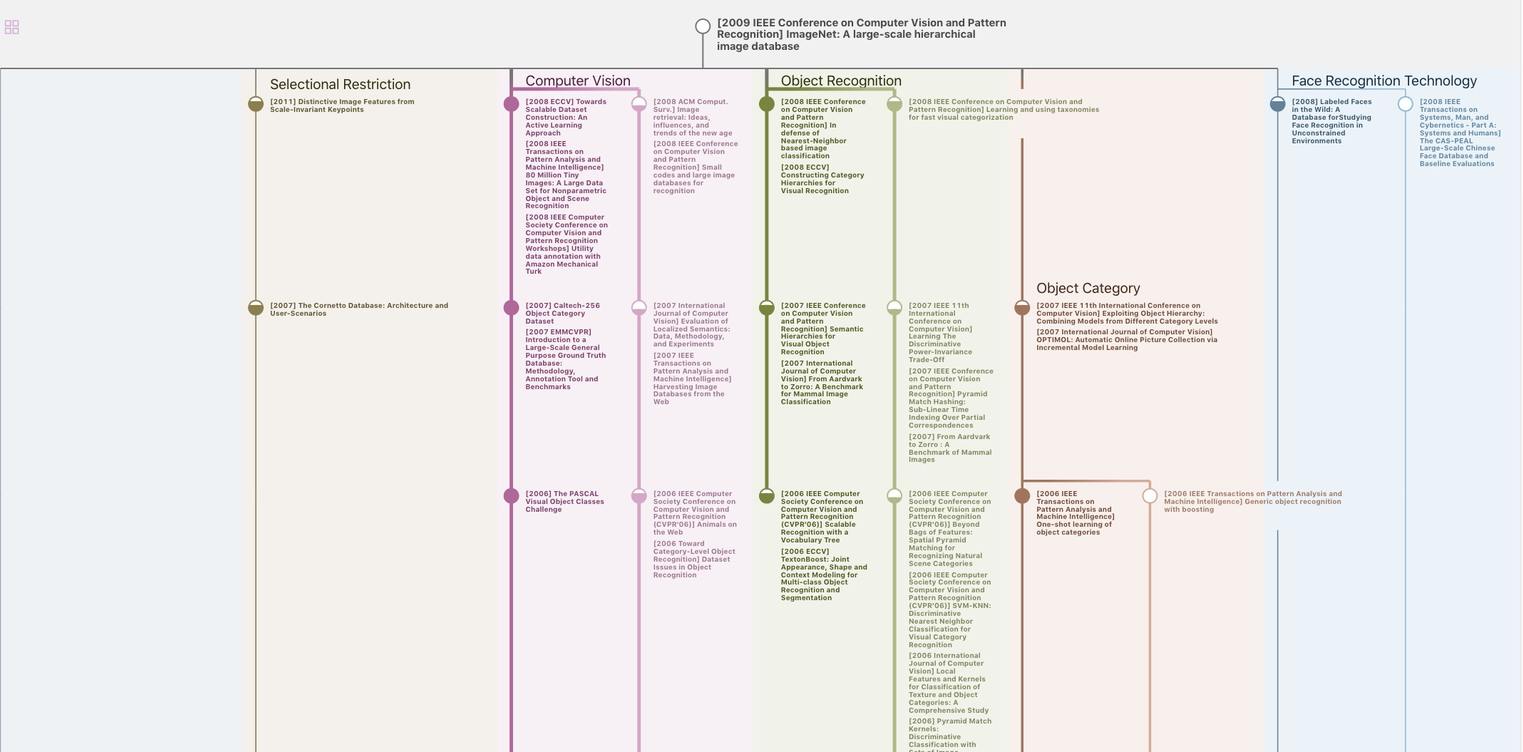
生成溯源树,研究论文发展脉络
Chat Paper
正在生成论文摘要