LRGAN: Visual anomaly detection using GAN with locality-preferred recoding
Journal of Visual Communication and Image Representation(2021)
摘要
Deep neural networks, including deep auto-encoder (DAE) and generative adversarial networks (GAN), have been extensively applied for visual anomaly detection. These models generally assume that reconstruction errors should be lower for normal samples but higher for anomalies. However, it has been found that DAE based models can sometimes reconstruct anomalies very well and thus result in false alarms or misdetections. To address this problem, we propose a model using GAN with locality-preferred recoding, named LRGAN. LRGAN is inspired by the observation that both normal and abnormal samples are not completely scattered throughout the latent space but clustered separately at some local regions. Therefore, a locality-preferred recoding (LR) module is designed to compulsively represent the latent vectors of anomalies by normal ones. As a result, reconstructions of anomalies will approximate to normal samples and corresponding residuals can thus be enlarged. To partly avoid latent vectors of normal samples being recoded, we further present an improved model using GAN with an adaptive LR (ALR) module, named LRGAN+. ALR applies the clustering algorithm to generate a more compact codebook; more importantly, it helps LRGAN + automatically skip the LR module for possible normal samples with a threshold strategy. Our proposed method is evaluated on two public datasets (i.e., MNIST and CIFAR-10) and one real-world industrial dataset (i.e., Fasteners), considering both one-class and multi-class anomaly detection protocols. Experimental results demonstrate that LRGAN is comparable with state-of-the-art methods and LRGAN + outperforms these methods on all datasets.
更多查看译文
关键词
Visual anomaly detection,GAN,Locality,Recoding
AI 理解论文
溯源树
样例
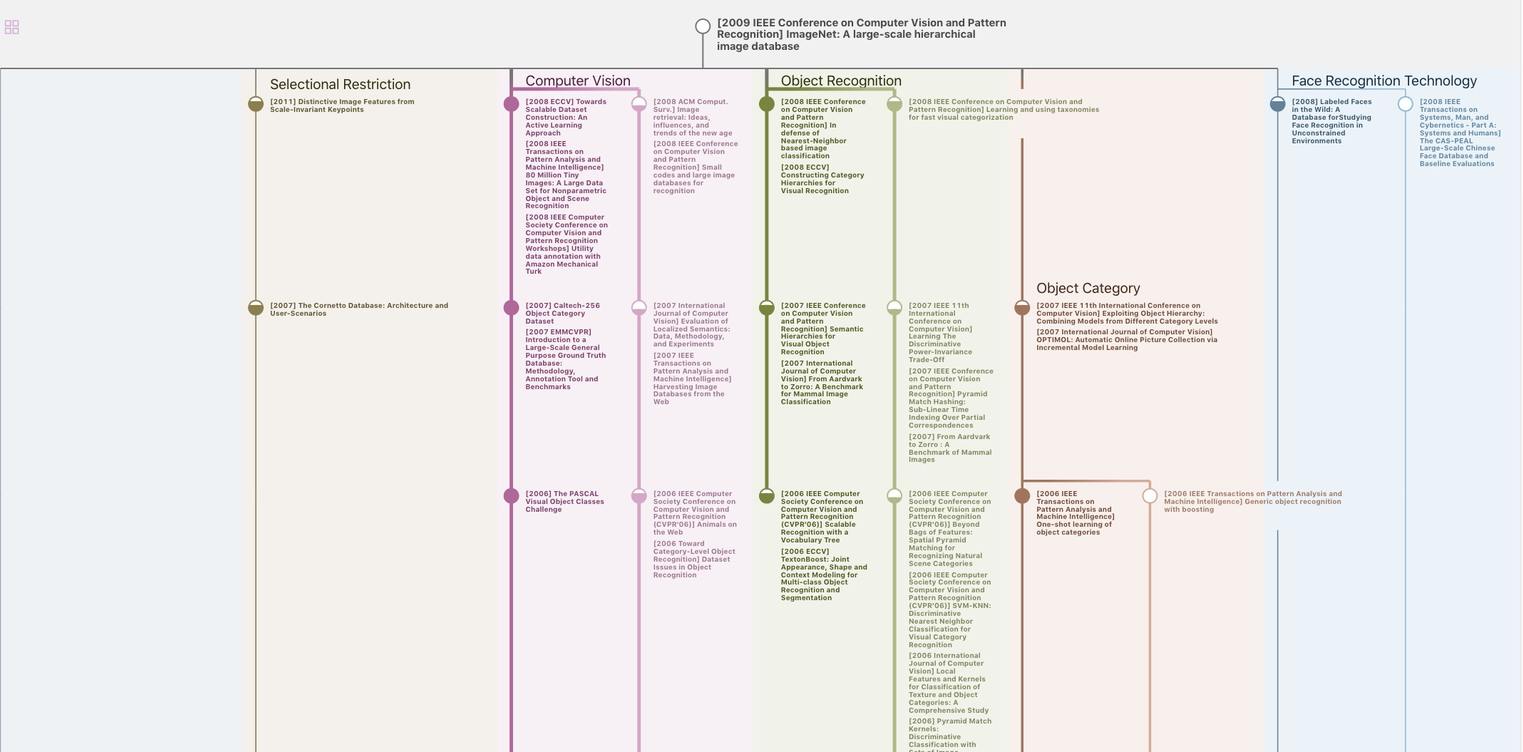
生成溯源树,研究论文发展脉络
Chat Paper
正在生成论文摘要