Can MOOC Instructor Be Portrayed by Semantic Features? Using Discourse and Clustering Analysis to Identify Lecture-Style of Instructors in MOOCs
FRONTIERS IN PSYCHOLOGY(2021)
摘要
Nowadays, most courses in massive open online course (MOOC) platforms are xMOOCs, which are based on the traditional instruction-driven principle. Course lecture is still the key component of the course. Thus, analyzing lectures of the instructors of xMOOCs would be helpful to evaluate the course quality and provide feedback to instructors and researchers. The current study aimed to portray the lecture styles of instructors in MOOCs from the perspective of natural language processing. Specifically, 129 course transcripts were downloaded from two major MOOC platforms. Two semantic analysis tools (linguistic inquiry and word count and Coh-Metrix) were used to extract semantic features including self-reference, tone, effect, cognitive words, cohesion, complex words, and sentence length. On the basis of the comments of students, course video review, and the results of cluster analysis, we found four different lecture styles: "perfect," "communicative," "balanced," and "serious." Significant differences were found between the different lecture styles within different disciplines for notes taking, discussion posts, and overall course satisfaction. Future studies could use fine-grained log data to verify the results of our study and explore how to use the results of natural language processing to improve the lecture of instructors in both MOOCs and traditional classes.
更多查看译文
关键词
semantic features,lecture style,MOOCs,LIWC,Coh-Metrix
AI 理解论文
溯源树
样例
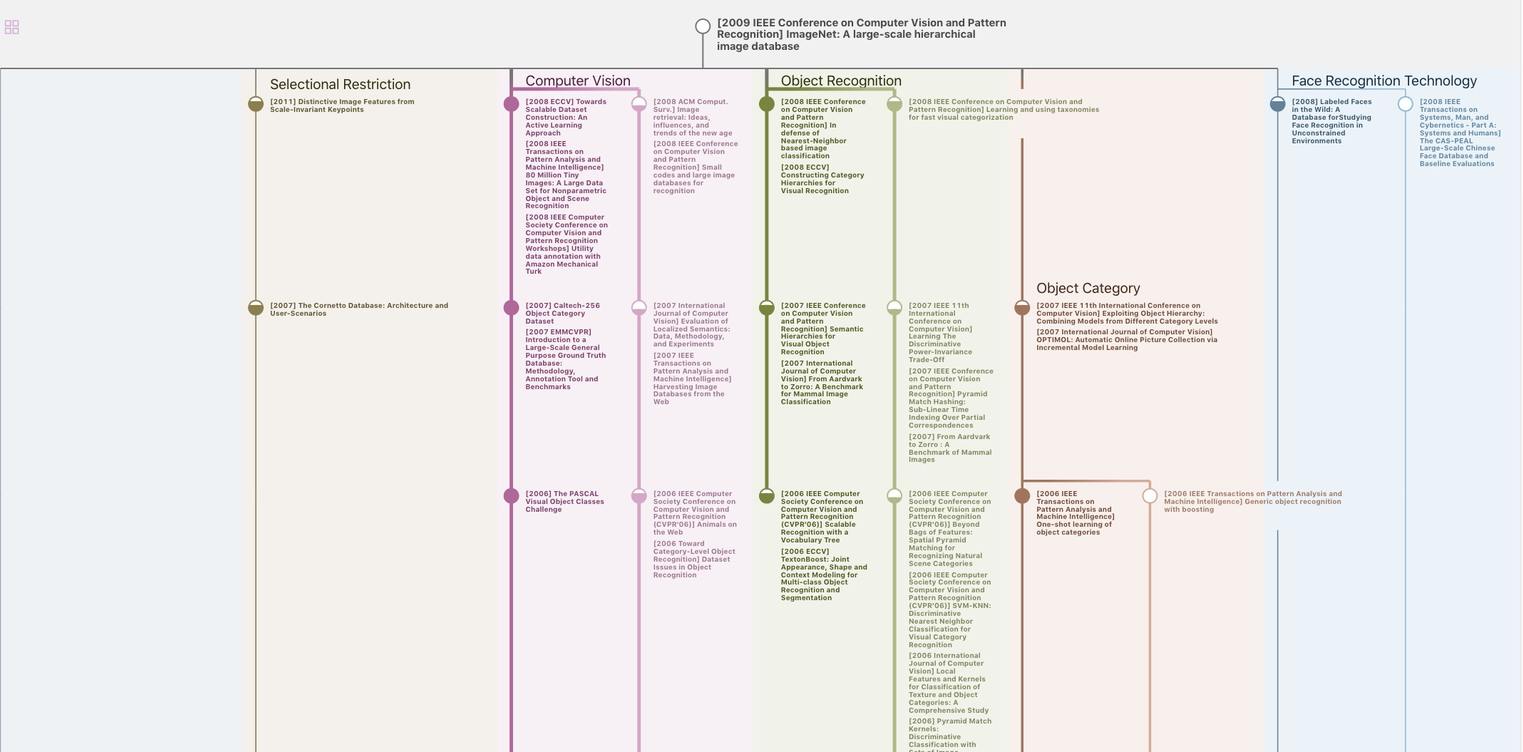
生成溯源树,研究论文发展脉络
Chat Paper
正在生成论文摘要