Learning Online for Unified Segmentation and Tracking Models
2021 INTERNATIONAL JOINT CONFERENCE ON NEURAL NETWORKS (IJCNN)(2021)
Abstract
Tracking requires building a discriminative model for the target in the inference stage. An effective way to achieve this is online learning, which can comfortably outperform models that are only trained offline. Recent research shows that visual tracking benefits significantly from the unification of visual tracking and segmentation due to its pixel-level discrimination. However, it imposes a great challenge to perform online learning for such a unified model. A segmentation model cannot easily learn from prior information given in the visual tracking scenario. In this paper, we propose TrackMLP: a novel meta-learning method optimized to learn from only partial information to resolve the imposed challenge. Our model is capable of extensively exploiting limited prior information hence possesses much stronger target-background discriminability than other online learning methods. Empirically, we show that our model achieves state-of-the-art performance and tangible improvement over competing models. Our model achieves improved average overlaps of 66:0%, 67:1%, and 68:5% in VOT2019, VOT2018, and VOT2016 datasets, which are 6:4%, 7:3%, and 6:4% higher than our baseline. Code will be made publicly available.
MoreTranslated text
Key words
unified segmentation,tracking,learning,models
AI Read Science
Must-Reading Tree
Example
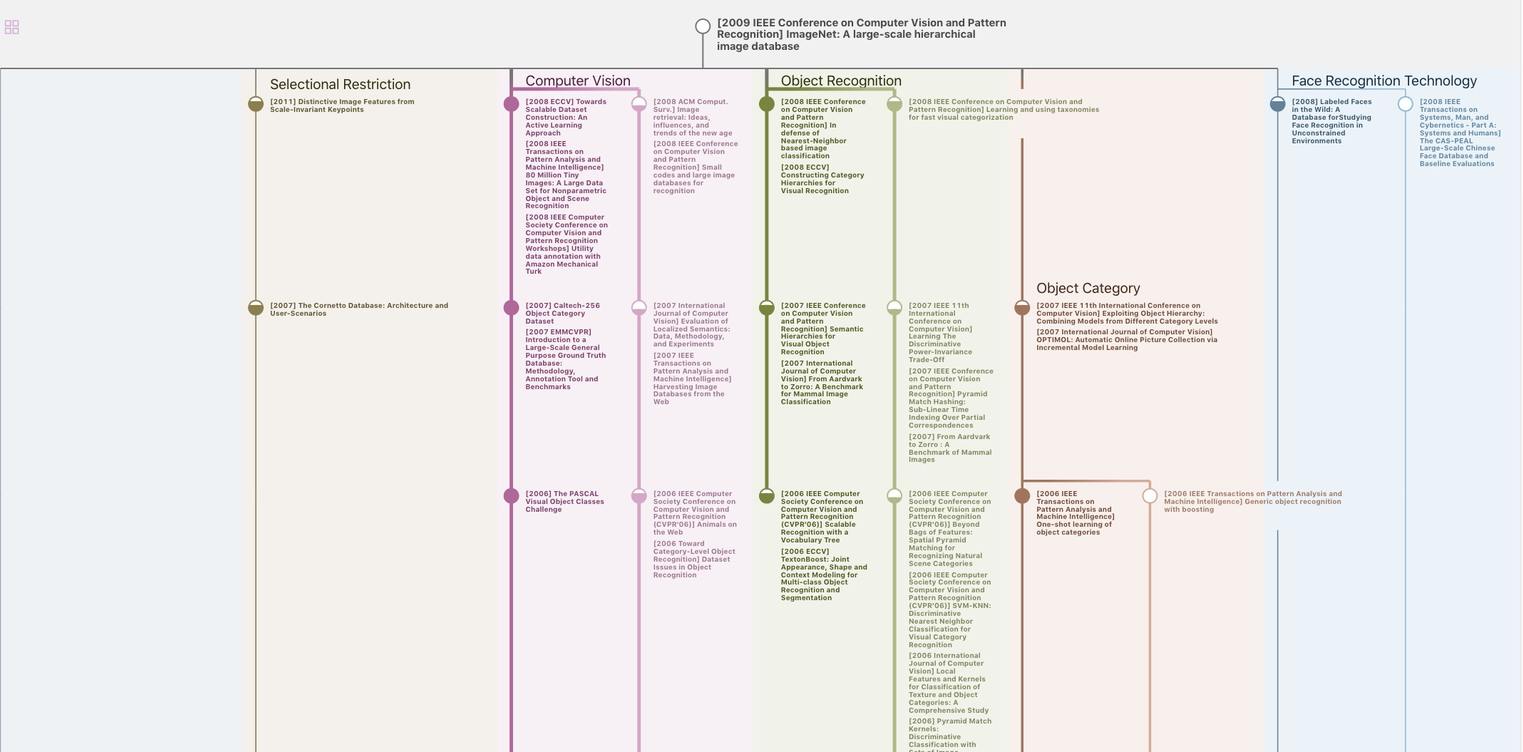
Generate MRT to find the research sequence of this paper
Chat Paper
Summary is being generated by the instructions you defined