An Efficient Bayesian Neural Network for Multiple Data Streams
2021 INTERNATIONAL JOINT CONFERENCE ON NEURAL NETWORKS (IJCNN)(2021)
摘要
Spatial and temporal data such as multiple data streams often have concept drift problems, which refers to changes of the data distributions over time. Once concept drift occurs, a stationary machine learning predictor will probably be invalid because the testing data have a different data distribution from that of the training data. Recent studies in data streams aim to address this issue by concept drift adaptation techniques. Concept drift adaptation methods update the predictor by time and have been validated to provide accurate real-time prediction for a single data stream. However, handling multiple relevant data streams is still an unsolved challenge in this area, considering many real-world applications generate multiple data streams that are simultaneously evolving. To fill this gap, we here present a predicting network for multiple data streams, named MuNet. MuNet leverages the dependency between data streams to efficiently lower down the computational cost of real-time predictions for multiple data streams. In MuNet, an online-learned Bayesian neural network (BNN) is designed as a connector between streams. The BNN-connector can continuously use the real-time information from only one base stream to correct the stationary predictor for the other streams, which avoids the high cost caused by repeated adaptation.
更多查看译文
关键词
Bayesian Neural Network, Spatial and temporal data mining, concept drift, multiple streams
AI 理解论文
溯源树
样例
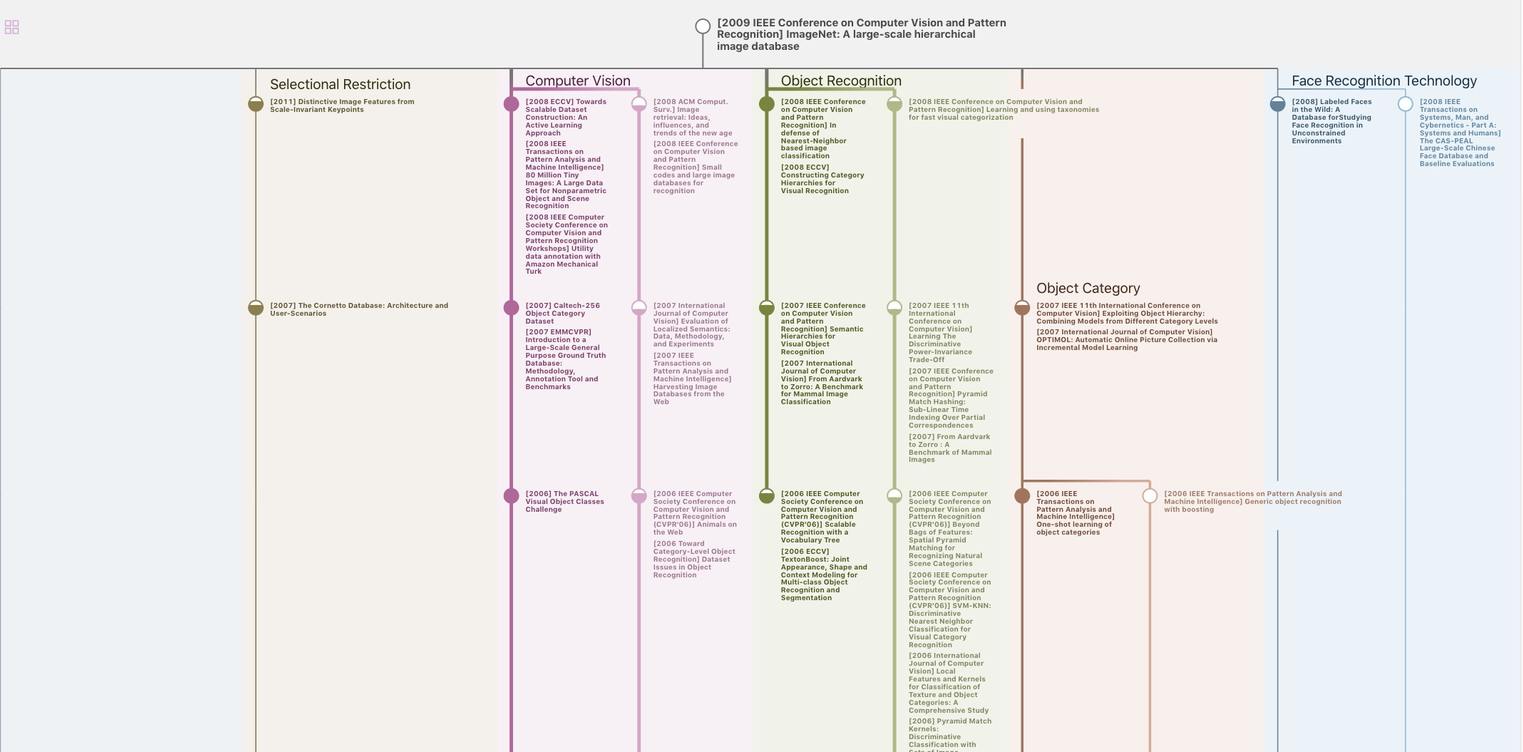
生成溯源树,研究论文发展脉络
Chat Paper
正在生成论文摘要