An Episodic Learning based Geolocation Detection Framework for Imbalanced Data
2021 INTERNATIONAL JOINT CONFERENCE ON NEURAL NETWORKS (IJCNN)(2021)
摘要
A social media user's geographical location is vital to many applications like local search and event detection. The scarcity of publicly available location information motivates researchers to predict user geolocation based on information such as tweet text and social interaction data. In this paper, we investigate and improve on the task of predicting a Twitter user's city-level location based on the content of the user's historical tweets. In order to train a reliable location classifier, previous studies on this topic have typically assumed that there are sufficient amount of users living in each cities. However, they simply ignore the fact that different demographic groups may participate in social media platforms, which results in a highly imbalanced data distribution. Being aware of this population imbalance issue, we propose an episodic learning based framework to extract a single representative for each class (location), so that classifiers can later be trained on a balanced class distribution. To examine the effectiveness of our method, we design experiments which involve two kinds of baselines, the state-of-the-art geolocation detection methods and the well-known approaches handling imbalanced data in classification. The results of experiments on the data collected from Twitter demonstrated the superiority of our method when compared with baselines.
更多查看译文
关键词
geolocation detection, imbalance classification, episodic training
AI 理解论文
溯源树
样例
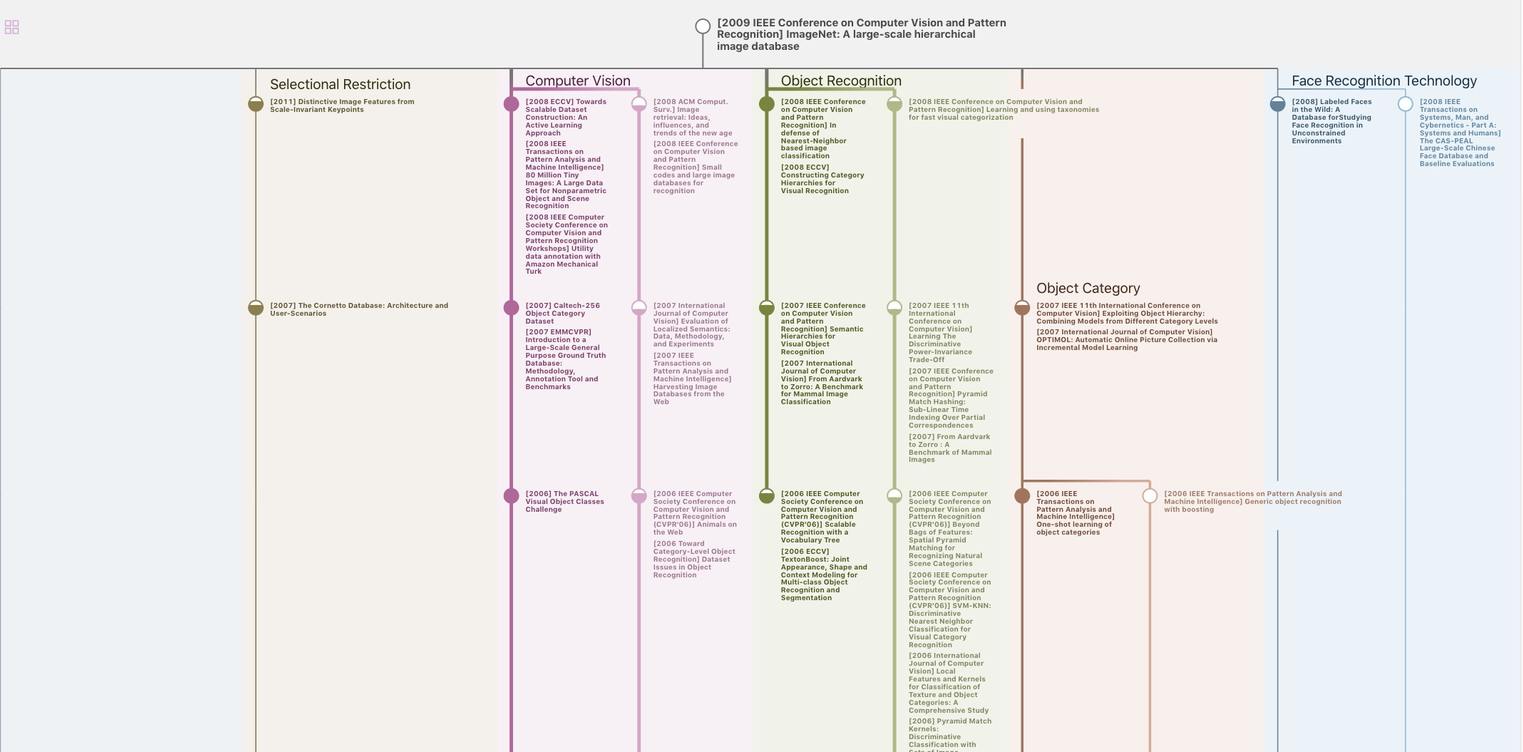
生成溯源树,研究论文发展脉络
Chat Paper
正在生成论文摘要