USET : A network based on Utterance hidden State transfEr for Task-oriented dialogue
2021 INTERNATIONAL JOINT CONFERENCE ON NEURAL NETWORKS (IJCNN)(2021)
摘要
Multi-turn dialogue is challenging because semantic information is not only contained in the current utterance, but also in the dialogue context. In fact, understanding multi-turn dialogue is a dynamic process. With the increase of dialogue turn, users' understanding is also changing. In this case, we propose a network based on utterance hidden state transfer for task-oriented dialogue (USET). In our model, we first extract the hidden state of previous utterance as the previous comprehension. Then, this comprehension is passed to the next turn. We take the comprehension as a prior knowledge to understand the semantic information in the dialogue context. Finally, we put the previous comprehension and current utterance together to understand current utterance. In order to realize the transfer of comprehension in dialogue, we propose a continuous sample training method: CST, which takes a multi-turn dialogue as a whole to understand. All the sentences in a dialogue are put into a batch for training. Our method makes use of previous comprehension and achieves the information exchange among dialogue. Experimental results on Stanford Multi-Domain dataset demonstrate that our model is superior to existing models.
更多查看译文
关键词
task-oriented dialogue, utterance hidden state, dynamic comprehension, training method
AI 理解论文
溯源树
样例
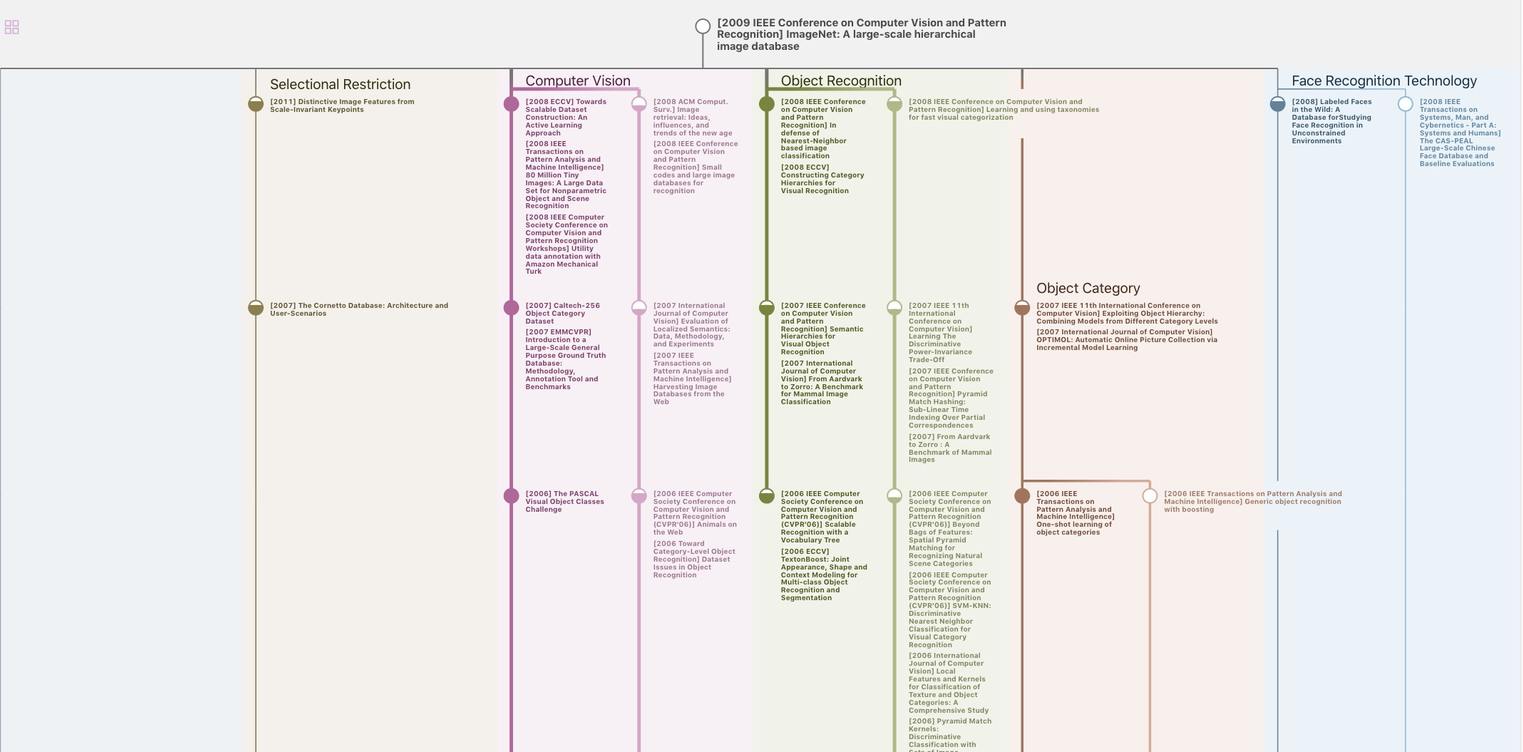
生成溯源树,研究论文发展脉络
Chat Paper
正在生成论文摘要