Adversarial Domain Adaptation with Correlation-Based Association Networks for Longitudinal Disk Fault Prediction
2021 INTERNATIONAL JOINT CONFERENCE ON NEURAL NETWORKS (IJCNN)(2021)
摘要
Disk fault is known to be the key cause of data loss in the modern large-scale data center, which affects the reliability and stability of the server and even the whole IT infrastructure, resulting in high financial cost. Recent works on disk fault prediction demonstrate the ability of machine learning techniques in the early prediction of disk failure. However, two limitations hinder the real-world application of current methods. First, they ignore the data heterogeneity in the data center, where distribution shifts commonly exist across different disk model types that decrease the model's performance. Second, the number of disks of different disk model types varies greatly in the data center, and current methods failed to deliver an acceptable performance over disk model types with few training samples. To address these limitations, we propose adversarial domain adaptation with correlation-based association networks (ADA-CBAN) to both mitigate the distribution shift problem and also to boost the performance on small-scaled data. Extensive experiments prove that our proposed model is effective and achieves new state-of-the-art. In addition, our post model analysis can also reveal important feature interactions and highlight the crucial period before disk faults, where both are useful information in real-world applications.
更多查看译文
关键词
domain adaptation, disk fault prediction, adversarial learning
AI 理解论文
溯源树
样例
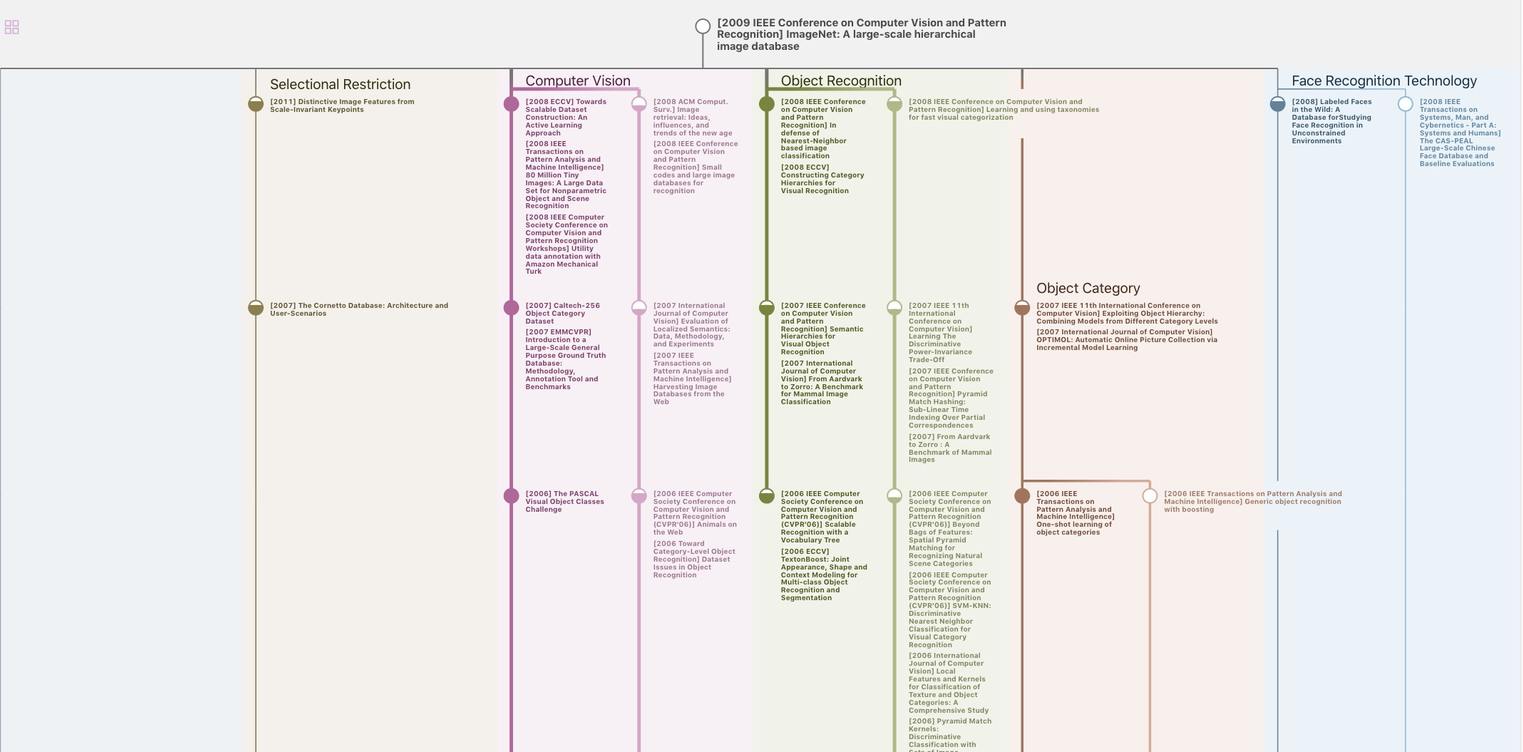
生成溯源树,研究论文发展脉络
Chat Paper
正在生成论文摘要