A Quantum Activation Function for Neural Networks: Proposal and Implementation
2021 INTERNATIONAL JOINT CONFERENCE ON NEURAL NETWORKS (IJCNN)(2021)
摘要
A non-linear activation function is an integral component of neural network algorithms used for various tasks such as data classification and pattern recognition. In neuromorphic/emerging-hardware-based implementations of neural network algorithms, the non-linear activation function is often implemented through dedicated analog electronics. This enables faster execution of the activation function during training and inference of neural networks compared to conventional digital implementation. Here, with a similar motivation, we propose a novel non-linear activation function that can be used in a neural network for data classification. Our activation function can be implemented by taking advantage of the inherent non-linearity in qubit preparation and SU(2) operation in quantum mechanics. These operations are directly implementable on quantum hardware through single-qubit quantum gates as we show here. In addition, the SU(2) parameters are adjustable here making the activation function adaptable; we adjust the parameters through classical feedback like in a variational algorithm in quantum machine learning. Using our proposed quantum activation function, we report accurate classification using popular machine learning data sets like Fisher's Iris, Wisconsin's Breast Cancer (WBC), Abalone, and MNIST on three different platforms: simulations on a classical computer, simulations on a quantum simulation framework like Qiskit, and experimental implementation on quantum hardware (IBM-Q). Then we use a Bloch-sphere-based approach to intuitively explain how our proposed quantum activation function, with its adaptability, helps in data classification.
更多查看译文
关键词
neuromorphic computing, quantum machine learning, neural network
AI 理解论文
溯源树
样例
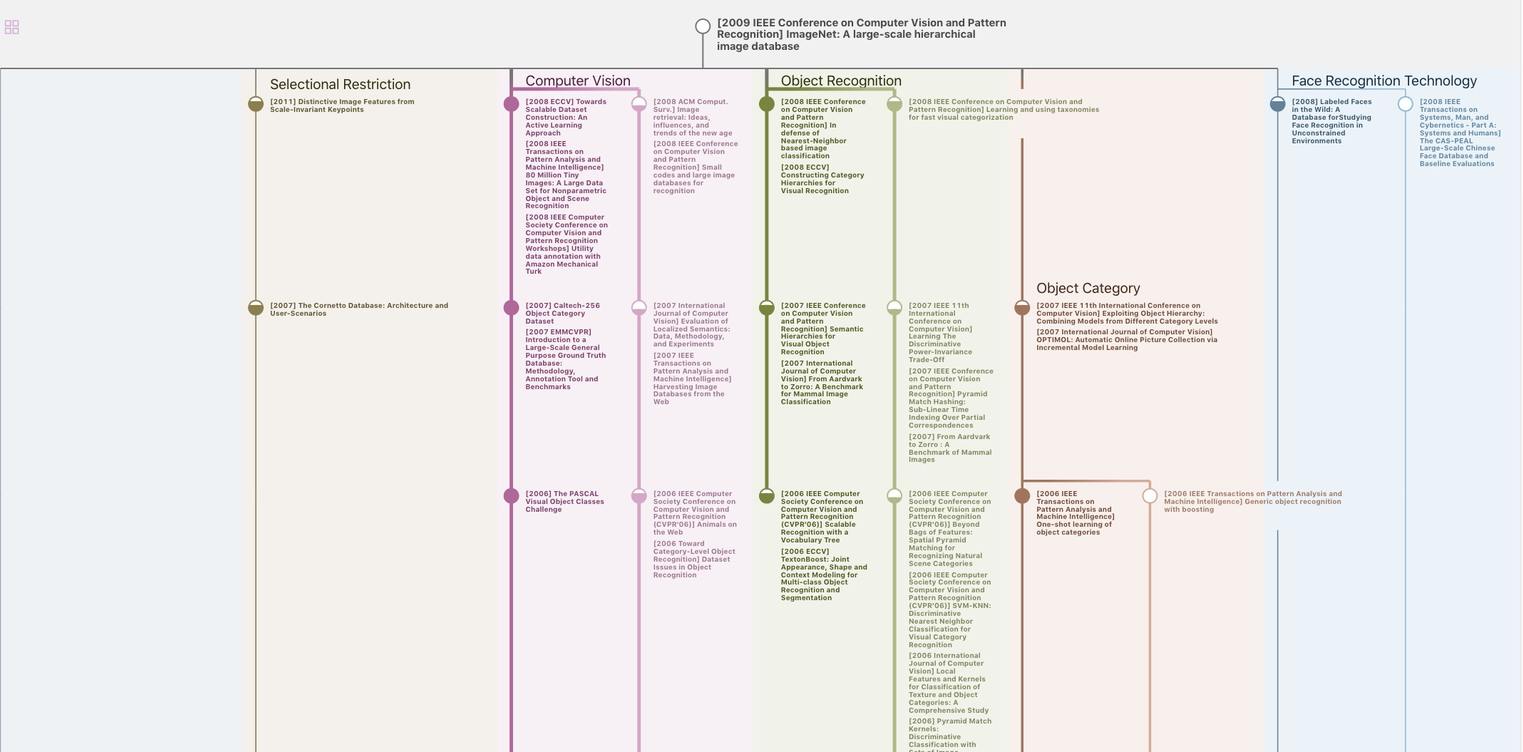
生成溯源树,研究论文发展脉络
Chat Paper
正在生成论文摘要