Canvas GAN: Bootstrapped Image-Conditional Models
2021 INTERNATIONAL JOINT CONFERENCE ON NEURAL NETWORKS (IJCNN)(2021)
摘要
Generative Adversarial Networks (GANs) learn generating functions that map a random noise distribution Z to a target data distribution. Usually, little attention is paid to the form of Z, resulting in nearly all models assuming Z follows a convenient parametric form (almost always Z similar to Normal(0, 1) or Z similar to Uniform(-1, 1)). However, we observe that imageconditional generators, such as those used in the CycleGAN, produce better quality generation than comparable single domain GANs can currently achieve. This is true even when the images being conditioned upon are substantially different from the target images. We hypothesize these models benefit from input which already has the general structure of images, even if their semantic content is different. As a result, we propose the Canvas GAN: using just a small handful of real images ("canvases"), we create random input for an image-conditional generator by randomly cropping, flipping along either or both axis, coloring, and resizing the canvas. These diverse samples allow the generator to edit an input that already exhibits natural image structure, as opposed to having to generate it from scratch from independent white noise.
更多查看译文
关键词
Canvas GAN,bootstrapped image-conditional models,generative adversarial networks,random noise distribution,target data distribution,parametric form,image-conditional generator,quality generation,target images,random input,natural image structure,single domain GANs
AI 理解论文
溯源树
样例
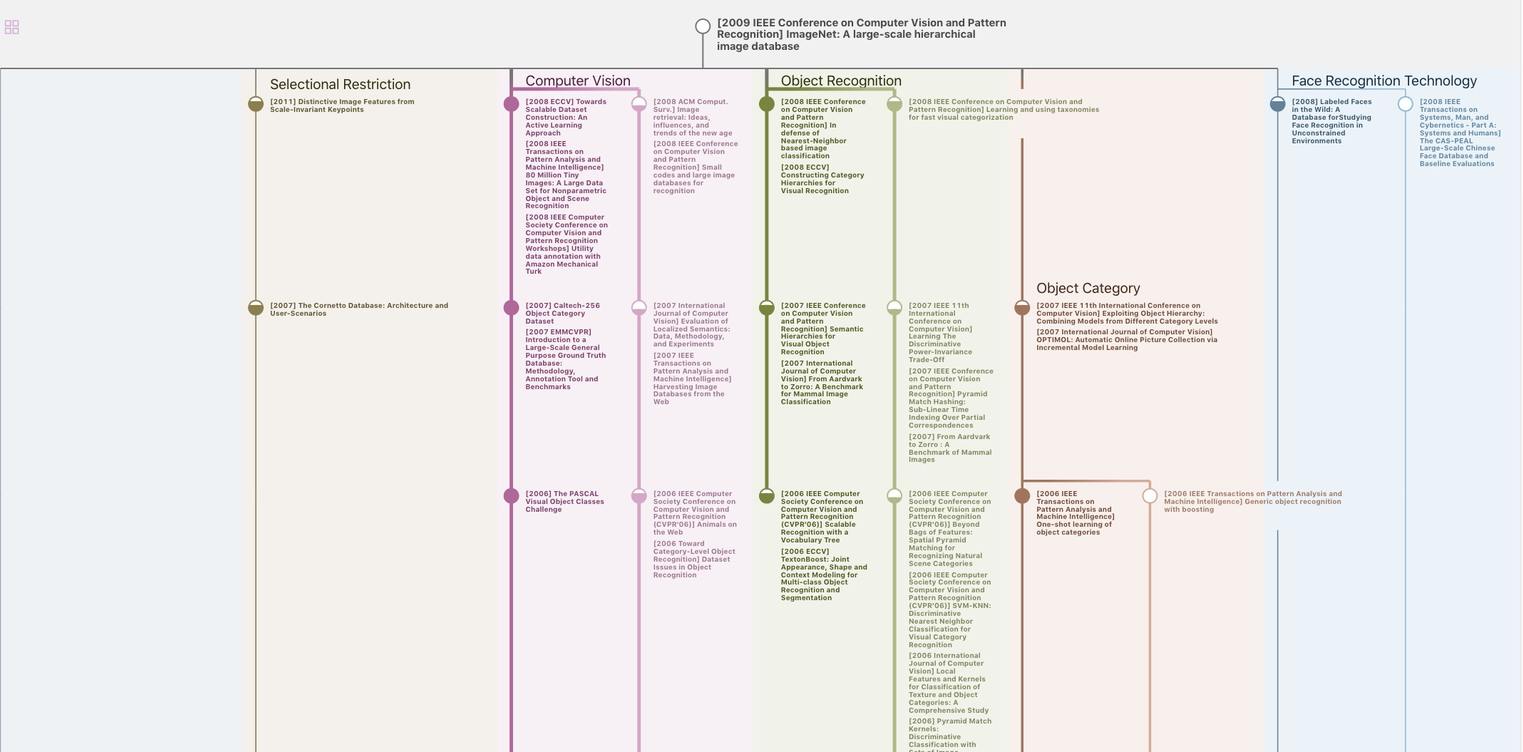
生成溯源树,研究论文发展脉络
Chat Paper
正在生成论文摘要