Noise Space Optimization for GANs
2021 INTERNATIONAL JOINT CONFERENCE ON NEURAL NETWORKS (IJCNN)(2021)
摘要
Traditional Generative Adversarial Networks (GANs) sample from a continuous stochastic noise distribution, and then treating that sample as a constant, optimize the parameters of a generator network on the average loss over the sample. In other words, the generator must take samples from a continuous input distribution, and on average, match a non-continuous target distribution (the training data). The result is that, as the generator transitions from one realistic image to another, some regions of the noise space correspond to relatively high quality images and other regions correspond to relatively low quality images. To avoid these relatively low quality areas, and allow the generator to optimize the shape of its noise distribution in a data-aware way, we present a novel sampling procedure: alternating (a) the traditional approach of optimizing the generator while holding the noise space sample constant, but then also (b) moving points in the noise space to improve the loss, using the direction of the gradient, while holding the generator parameters constant. We demonstrate that this procedure, which we call noise space optimization, improves the overall quality of samples obtained from an identical model without it on a wide array of canonical datasets and training paradigms.
更多查看译文
关键词
noise space optimization,GANs,continuous stochastic noise distribution,generator network,average loss,continuous input distribution,noncontinuous target distribution,training data,generator transitions,noise space correspond,relatively high quality images,relatively low quality images,relatively low quality areas,sampling procedure,noise space sample constant,generator parameters
AI 理解论文
溯源树
样例
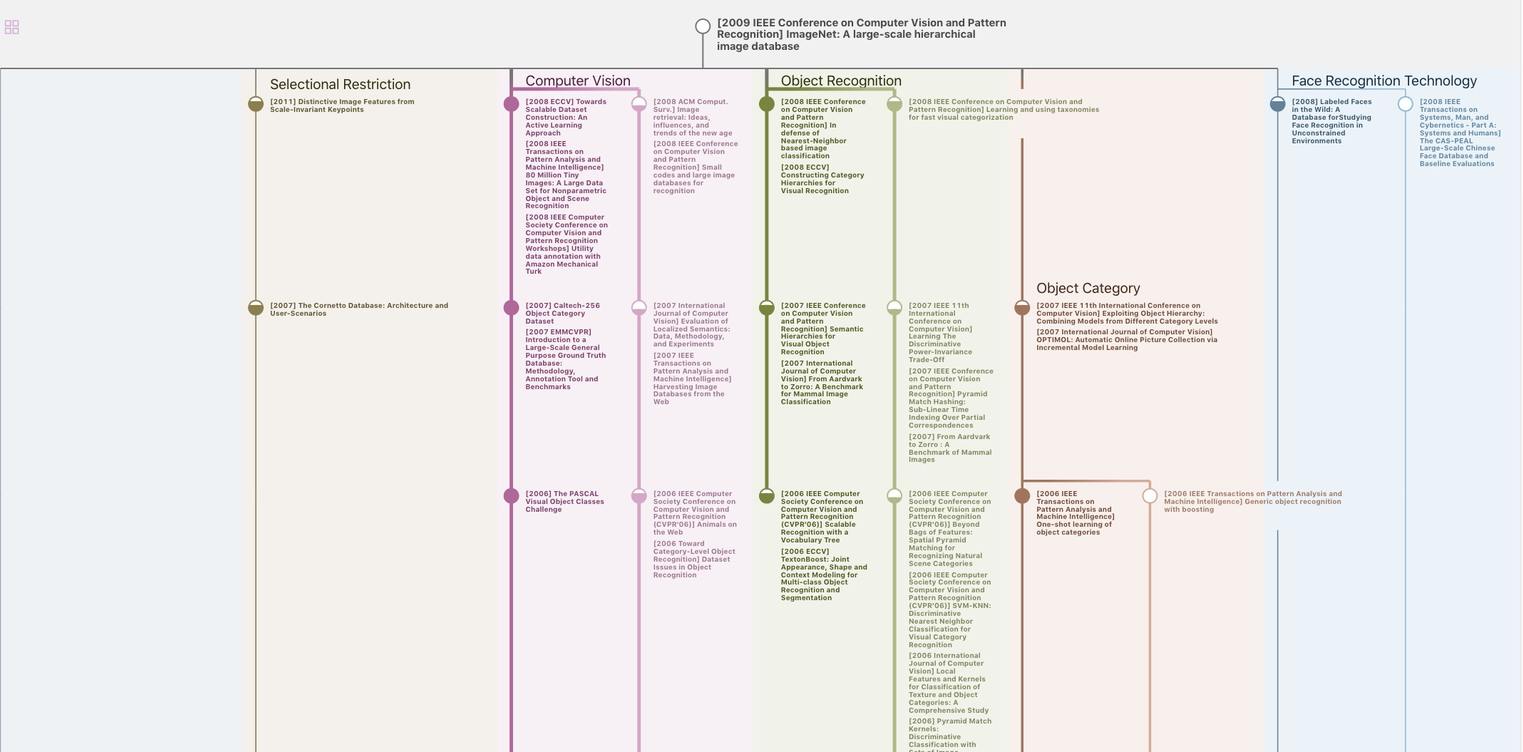
生成溯源树,研究论文发展脉络
Chat Paper
正在生成论文摘要