Learning Infant Brain Developmental Connectivity for Cognitive Score Prediction.
MLMI@MICCAI(2021)
摘要
During infancy, the human brain develops rapidly in terms of structure, function and cognition. The tight connection between cognitive skills and brain morphology motivates us to focus on individual level cognitive score prediction using longitudinal structural MRI data. In the early postnatal stage, the massive brain region connections contain some intrinsic topologies, such as small-worldness and modular organization. Accordingly, graph convolutional networks can be used to incorporate different region combinations to predict the infant cognitive scores. Nevertheless, the definition of the brain region connectivity remains a problem. In this work, we propose a crafted layer, the Inter-region Connectivity Module (ICM), to effectively build brain region connections in a data-driven manner. To further leverage the critical cues hidden in the development patterns, we choose path signature as the sequential data descriptor to extract the essential dynamic information of the region-wise growth trajectories. With these region-wise developmental features and the inter-region connectivity, a novel Cortical Developmental Connectivity Network (CDC-Net) is built. Experiments on a longitudinal infant dataset within 3 time points and hundreds of subjects show our superior performance, outperforming classical machine learning based methods and deep learning based algorithms.
更多查看译文
关键词
Infant cognition prediction,Brain region connectivity,Longitudinal analysis
AI 理解论文
溯源树
样例
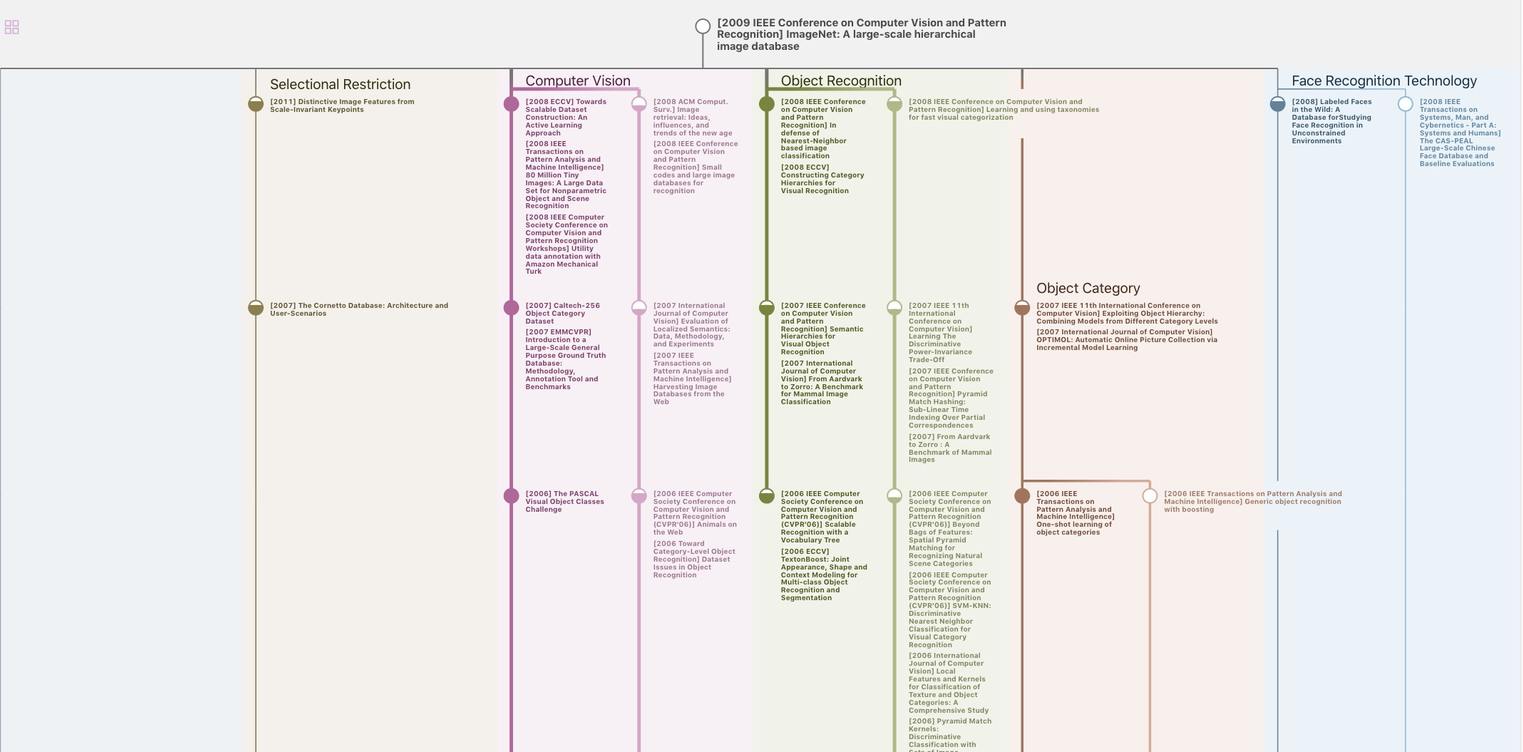
生成溯源树,研究论文发展脉络
Chat Paper
正在生成论文摘要