Is Ai Ground Truth Really True? The Dangers Of Training And Evaluating Ai Tools Based On Experts' Know-What1
MIS QUARTERLY(2021)
摘要
Organizational decision-makers need to evaluate AI tools in light of increasing claims that such tools outperform human experts. Yet, measuring the quality of knowledge work is challenging, raising the question of how to evaluate AI performance in such contexts. We investigate this question through a field study of a major U.S. hospital, observing how managers evaluated five different machine-learning (ML) based AI tools. Each tool reported high performance according to standard AI accuracy measures, which were based on ground truth labels provided by qualified experts. Trying these tools out in practice, however, revealed that none of them met expectations. Searching for explanations, managers began confronting the high uncertainty of experts' know-what knowledge captured in ground truth labels used to train and validate ML models. In practice, experts address this uncertainty by drawing on rich know-how practices, which were not incorporated into these ML-based tools. Discovering the disconnect between AI's know-what and experts' know-how enabled managers to better understand the risks and benefits of each tool. This study shows dangers of treating ground truth labels used in ML models objectively when the underlying knowledge is uncertain. We outline implications of our study for developing, training, and evaluating AI for knowledge work.
更多查看译文
关键词
Artificial intelligence, evaluation , uncertainty , new technology , professional , knowledge, work innovation , know-how , medical diagnosis, ground truth
AI 理解论文
溯源树
样例
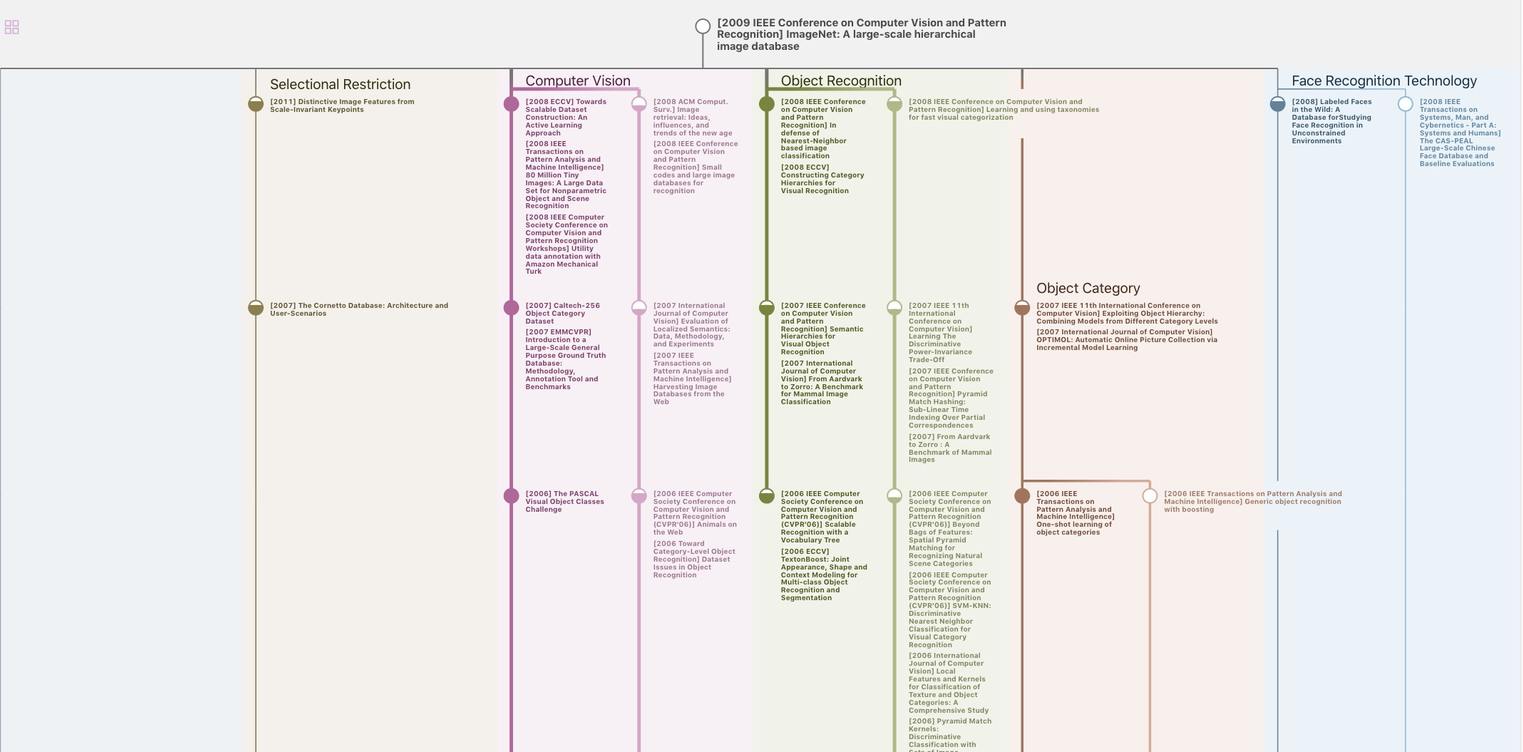
生成溯源树,研究论文发展脉络
Chat Paper
正在生成论文摘要