Automatic estimation of ulcerative colitis severity from endoscopy videos using ordinal multi-instance learning
COMPUTER METHODS IN BIOMECHANICS AND BIOMEDICAL ENGINEERING-IMAGING AND VISUALIZATION(2022)
摘要
Ulcerative colitis (UC) is an inflammatory bowel disease characterised by inflammation of the large intestine. UC severity is often represented by the Mayo Endoscopic Subscore (MES) which quantifies disease activity from endoscopy videos. In clinical trials, an endoscopy video is assigned an MES based upon the most severe inflammation observed in the video. For this reason, severe inflammation spread throughout the colon will receive the same MES as an otherwise healthy colon with a small localized inflammation. Therefore, the extent of disease activity throughout the colon may not be completely captured by the MES. In this work, we aim to automatically estimate MES for each frame in an endoscopy video to provide a higher resolution assessment of UC. Because annotating severity at the frame-level is highly expensive and labour-intensive, we propose a novel weakly supervised, ordinal classification method to estimate frame severity from video labels alone. Using clinical trial data, we demonstrate that our models achieve substantial agreement with ground truth labels, comparable to the agreement between expert clinicians. These findings indicate that our framework could serve as a foundation for novel clinical endpoints, based on amore localised scoring system, to better evaluate UC drug efficacy in clinical trials.
更多查看译文
关键词
IBD, Ulcerative Colitis, endoscopy video, severity estimation, weak supervision, multi-instance learning, ordinal classification, deep learning, convolutional neural networks
AI 理解论文
溯源树
样例
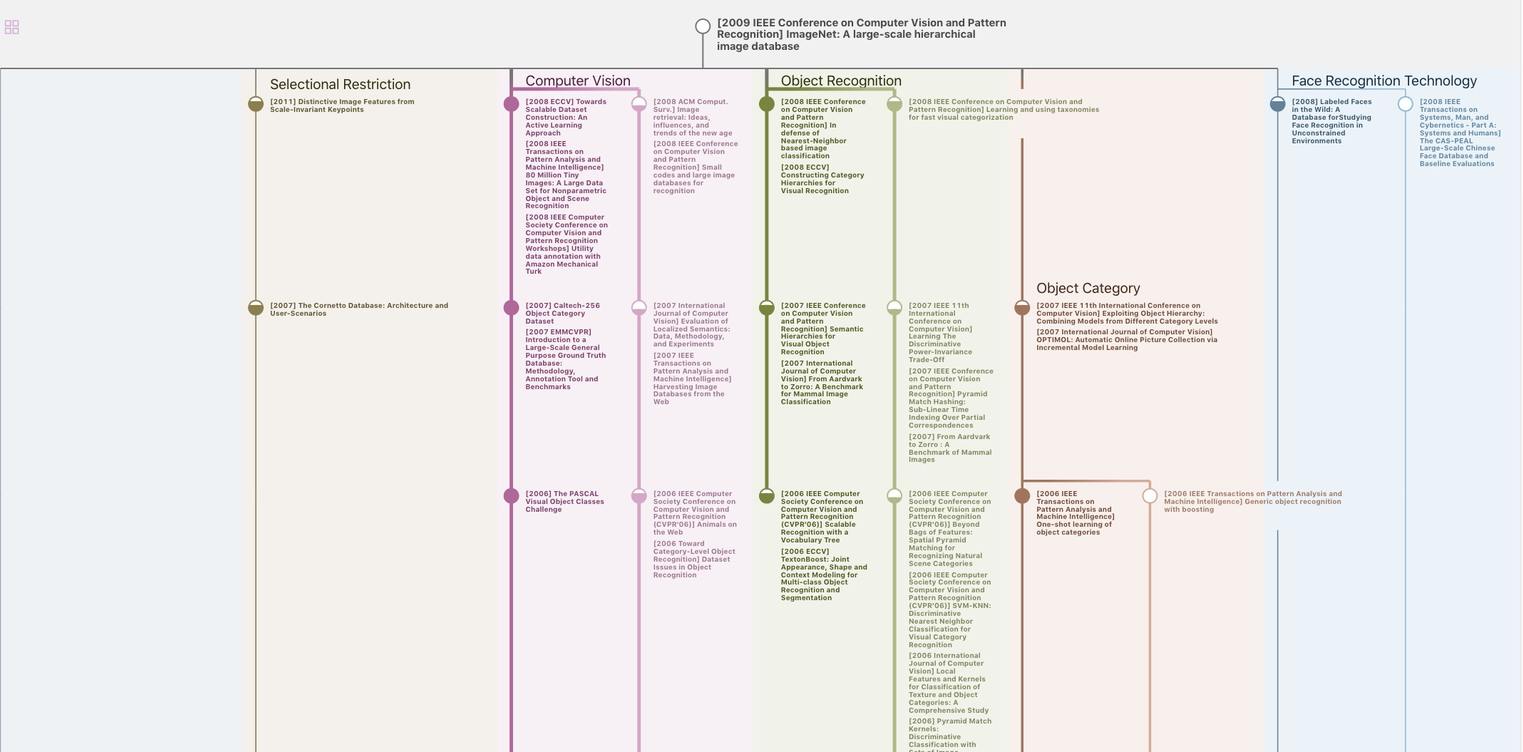
生成溯源树,研究论文发展脉络
Chat Paper
正在生成论文摘要