Self-supervised Visual Representation Learning for Histopathological Images
MEDICAL IMAGE COMPUTING AND COMPUTER ASSISTED INTERVENTION - MICCAI 2021, PT II(2021)
Abstract
Self-supervised learning provides a possible solution to extract effective visual representations from unlabeled histopathological images. However, existing methods either fail to make good use of domain-specific knowledge, or rely on side information like spatial proximity and magnification. In this paper, we propose CS-CO, a hybrid self-supervised visual representation learning method tailored for histopathological images, which integrates advantages of both generative and discriminative models. The proposed method consists of two self-supervised learning stages: cross-stain prediction (CS) and contrastive learning (CO), both of which are designed based on domain-specific knowledge and do not require side information. A novel data augmentation approach, stain vector perturbation, is specifically proposed to serve contrastive learning. Experimental results on the public dataset NCT-CRC-HE-100K demonstrate the superiority of the proposed method for histopathological image visual representation. Under the common linear evaluation protocol, our method achieves 0.915 eight-class classification accuracy with only 1,000 labeled data, which is about 1.3% higher than the fully-supervised ResNet18 classifier trained with the whole 89,434 labeled training data. Our code is available at https://github.com/easonyang1996/CS-CO.
MoreTranslated text
Key words
Self-supervised learning, Stain separation, Contrastive representation learning, Histopathological images
AI Read Science
Must-Reading Tree
Example
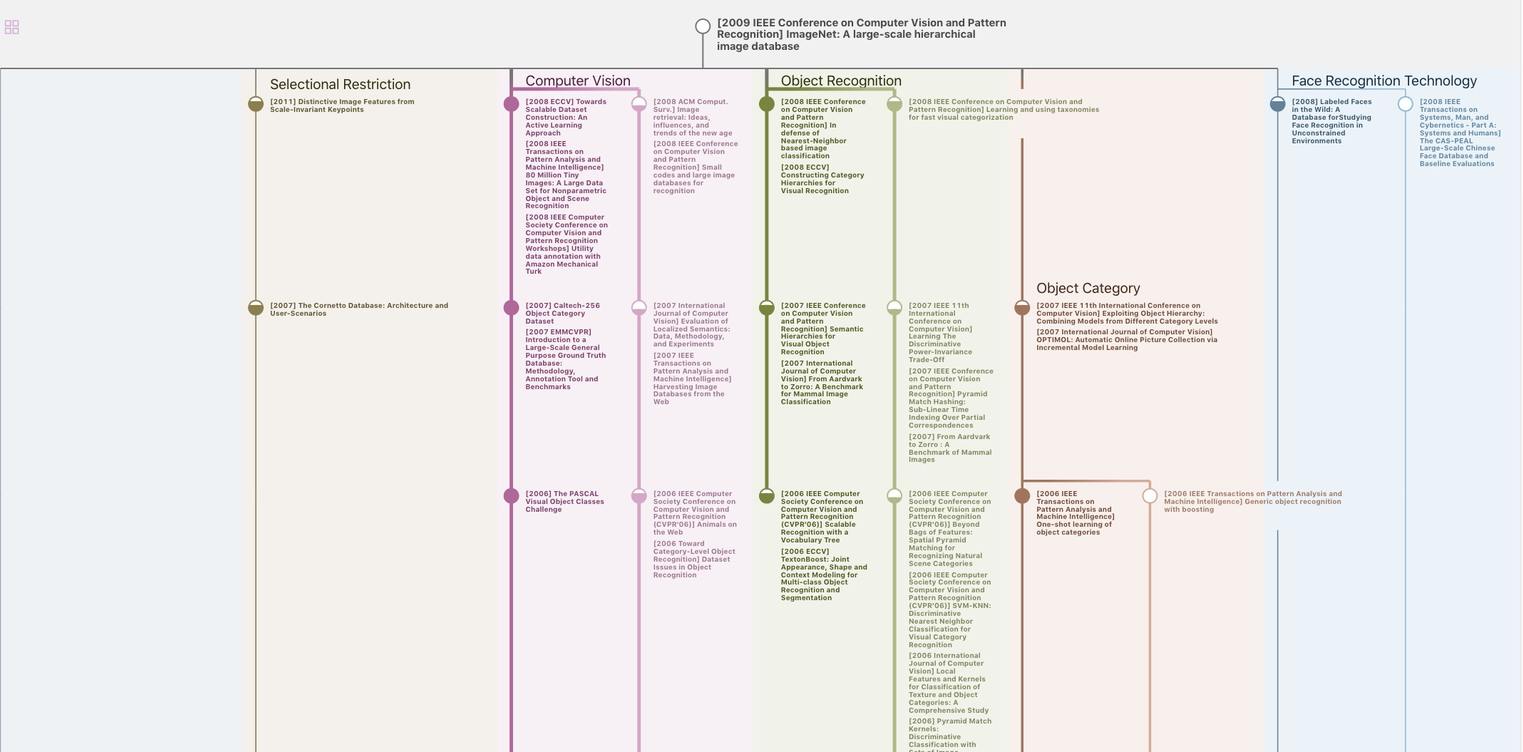
Generate MRT to find the research sequence of this paper
Chat Paper
Summary is being generated by the instructions you defined