Revisiting Contour-Driven and Knowledge-Based Deformable Models: Application to 2D-3D Proximal Femur Reconstruction from X-ray Images
MEDICAL IMAGE COMPUTING AND COMPUTER ASSISTED INTERVENTION - MICCAI 2021, PT VI(2021)
摘要
In many clinical applications, 3D reconstruction of patient-specific structures is of major interest. Despite great effort put in 2D-3D reconstruction, gold standard bone reconstruction obtained by segmentation on CT images is still mostly used - at the expense of exposing patients to significant ionizing radiation and increased health costs. State-of-the-art 2D-3D reconstruction methods are based on non-rigid registration of digitally reconstructed radiographs (DRR) - aiming at full automation - but with varying accuracy often exceeding clinical requirements. Conversely, contour-based approaches can lead to accurate results but strongly depend on the quality of extracted contours and have been left aside in recent years. In this study, we revisit a patient-specific 2D-3D reconstruction method for the proximal femur based on contours, image cues, and knowledge-based deformable models. 3D statistical shape models were built using 199 CT scans from THA patients that were used to generate pairs of high fidelity DRRs. Convolutional neural networks were trained using the DRRs to investigate automatic contouring. Experiments were conducted on the DRRs, and calibrated radiographs of a pelvis phantom and volunteers - with an analysis of the quality of contouring and its automatization. Using manual contours and DRR, the best reconstruction error was 1.02 mm With state-of-the-art results for 2D-3D reconstruction of the proximal femur, we highlighted the relevance and challenges of using contour-driven reconstruction to yield patient-specific models.
更多查看译文
关键词
2D-3D X-ray reconstruction, Shape priors, Deformable model
AI 理解论文
溯源树
样例
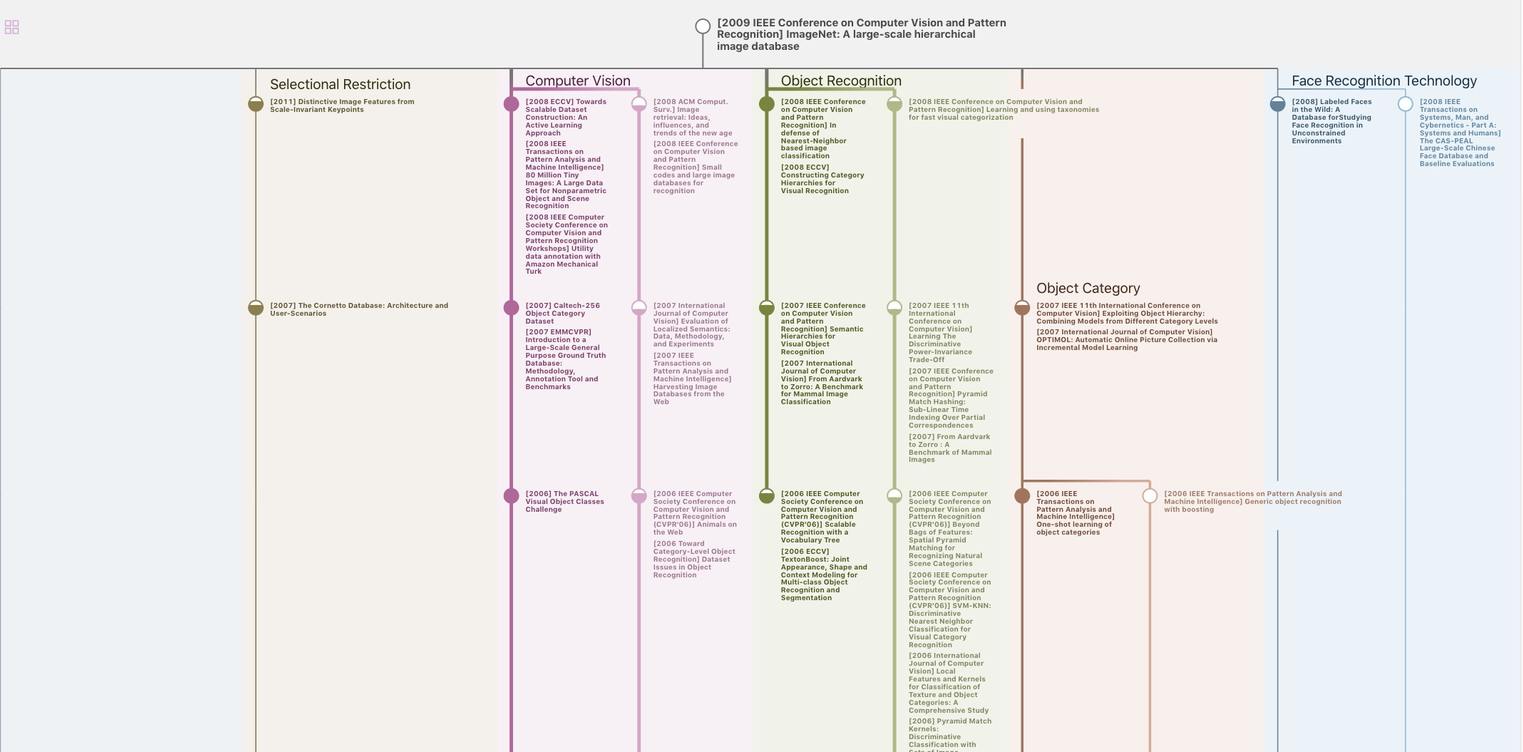
生成溯源树,研究论文发展脉络
Chat Paper
正在生成论文摘要