Annotation-Efficient Cell Counting
MEDICAL IMAGE COMPUTING AND COMPUTER ASSISTED INTERVENTION - MICCAI 2021, PT VIII(2021)
摘要
Recent advances in deep learning have achieved impressive results on microscopy cell counting tasks. The success of deep learning models usually needs sufficient training data with manual annotations, which can be time-consuming and costly. In this paper, we propose an annotation-efficient cell counting approach which injects cell counting networks into an active learning framework. By designing a multi-task learning in the cell counter network model, we leverage unlabeled data for feature representation learning and use deep clustering to group unlabeled data. Rather than labeling every cell in each training image, the deep active learning only suggests the most uncertain, diverse, representative and rare image regions for annotation. Evaluated on four widely used cell counting datasets, our cell counter trained by a small subset of training data suggested by the deep active learning, achieves superior performance compared to state-of-the-arts with full training or other suggestive annotations. Our code is available at htips://github.com/cybmi-research/AnnotationEfficient-CellCounting.
更多查看译文
关键词
Cell counting, Suggestive annotation, Active learning
AI 理解论文
溯源树
样例
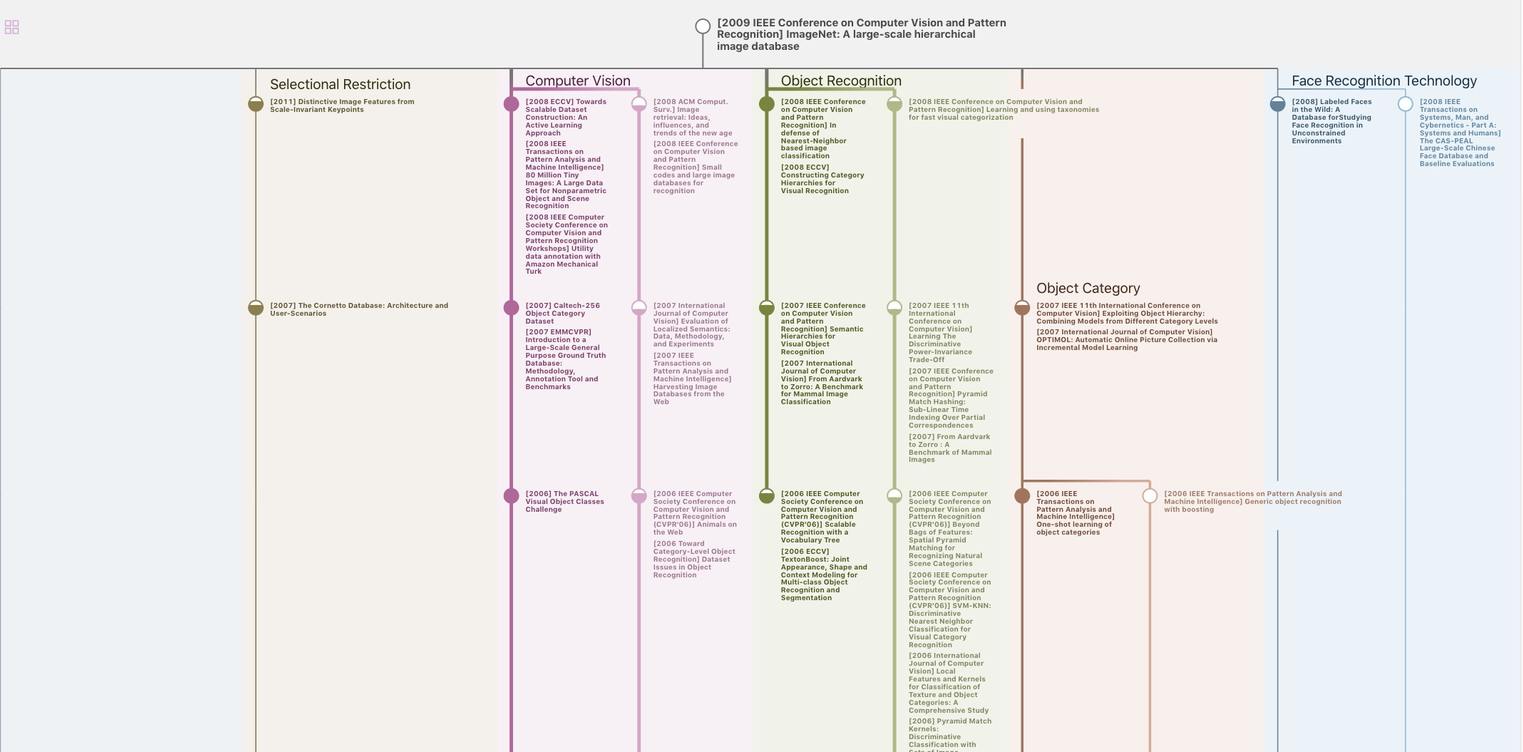
生成溯源树,研究论文发展脉络
Chat Paper
正在生成论文摘要