MetaCon: Meta Contrastive Learning for Microsatellite Instability Detection
MEDICAL IMAGE COMPUTING AND COMPUTER ASSISTED INTERVENTION - MICCAI 2021, PT VIII(2021)
摘要
Colorectal cancer (CRC) patients who are detected as microsatellite instability (MSI) can receive precise targeted therapies, but existing MSI detection methods are not available to all patients due to various restrictions. The achievements of deep learning in image processing provide the possibility of using pathological images for MSI detection. However, traditional deep networks can not achieve satisfied performance due to discrepancies between MSI patients, which reduces the generalization ability of deep learning models. Noisy labels also hinder the learning of an accurate model. To address these issues, we propose a model in a meta contrastive learning framework (MetaCon) accompanied with an attention-based feature fusion block. In MetaCon, we iteratively train a backbone with a cross entropy loss and a contrastive loss to learn a patient-independent MSI classifier for patches segmented from pathological images. We then blend features of patches from the same patient in an attention-based way, automatically focusing on reliable patches. Finally, we make a patient-level prediction by voting. Experiments on two public datasets from The Cancer Genome Atlas show superiority of our model over previous methods. The patient-level AUC is improved by 8% on average compared to the baseline model. Ablation studies prove effectiveness of each component in our model.
更多查看译文
关键词
Microsatellite instability detection, Meta-learning, Contrastive learning, Pathological images analysis
AI 理解论文
溯源树
样例
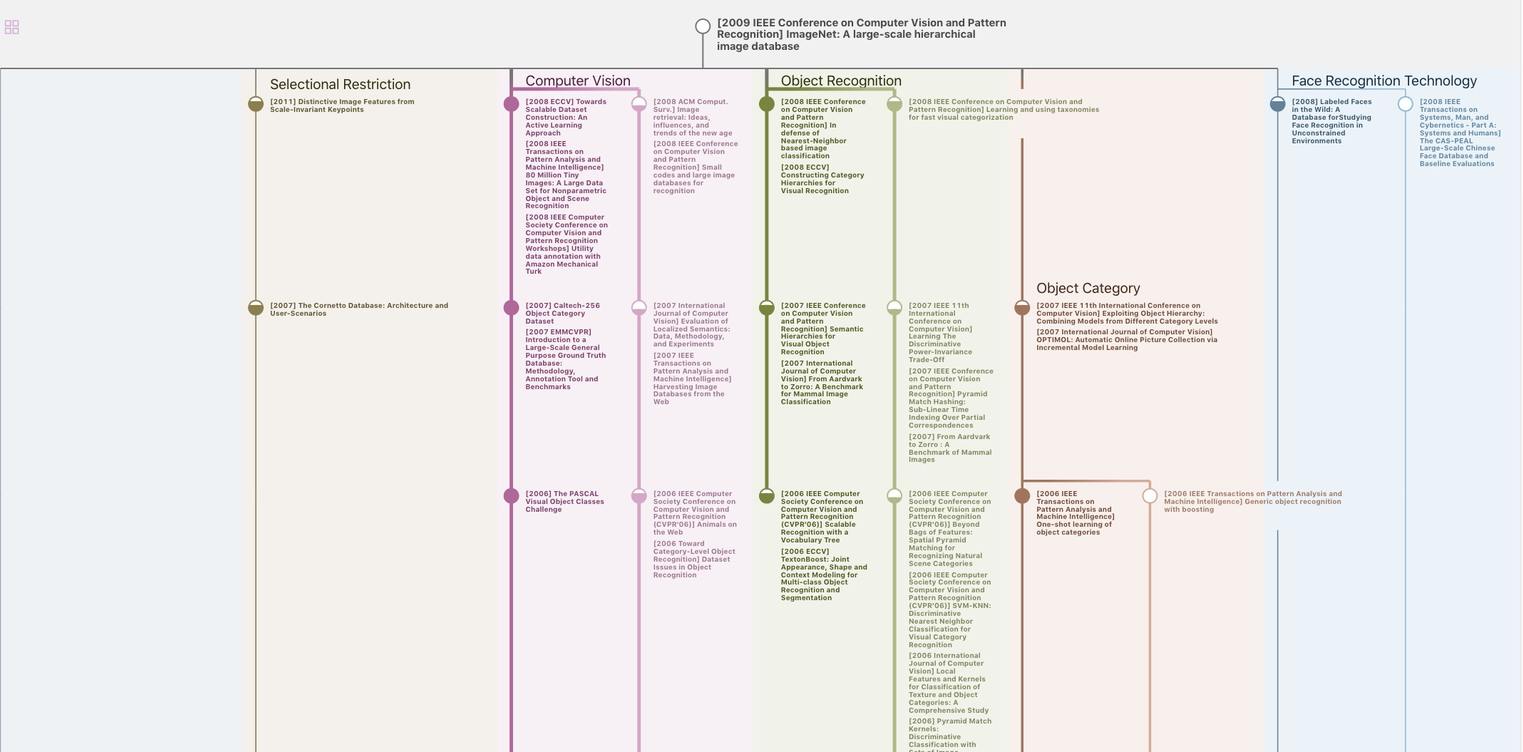
生成溯源树,研究论文发展脉络
Chat Paper
正在生成论文摘要