MorphSet: Improving Renal Histopathology Case Assessment Through Learned Prognostic Vectors
MEDICAL IMAGE COMPUTING AND COMPUTER ASSISTED INTERVENTION - MICCAI 2021, PT VIII(2021)
摘要
Computer Aided Diagnosis (CAD) systems for renal histopathology applications aim to understand and replicate nephropathologists' assessments of individual morphological compartments (e.g. glomeruli) to render case-level histological diagnoses. Deep neural networks (DNNs) hold great promise in addressing the poor intra- and interobserver agreement between pathologists. This being said, the generalization ability of DNNs heavily depends on the quality and quantity of training labels. Current "consensus" labeling strategies require multiple pathologists to evaluate every compartment unit over thousands of crops, resulting in enormous annotative costs. Additionally, these techniques fail to address the underlying reproducibility issues we observe across various diagnostic feature assessment tasks. To address both of these limitations, we introduce MorphSet, an end-to-end architecture inspired by Set Transformers which maps the combined encoded representations of Monte Carlo (MC) sampled glomerular compartment crops to produce Whole Slide Image (WSI) predictions on a case basis without the need for expensive fine-grained morphological feature labels. To evaluate performance, we use a kidney transplant Antibody Mediated Rejection (AMR) dataset, and show that we are able to achieve 98.9% case level accuracy, outperforming the consensus label baseline. Finally, we generate a visualization of prediction confidence derived from our MC evaluation experiments, which provides physicians with valuable feedback.
更多查看译文
关键词
Self attention, Antibody Mediated Rejection, Morphology
AI 理解论文
溯源树
样例
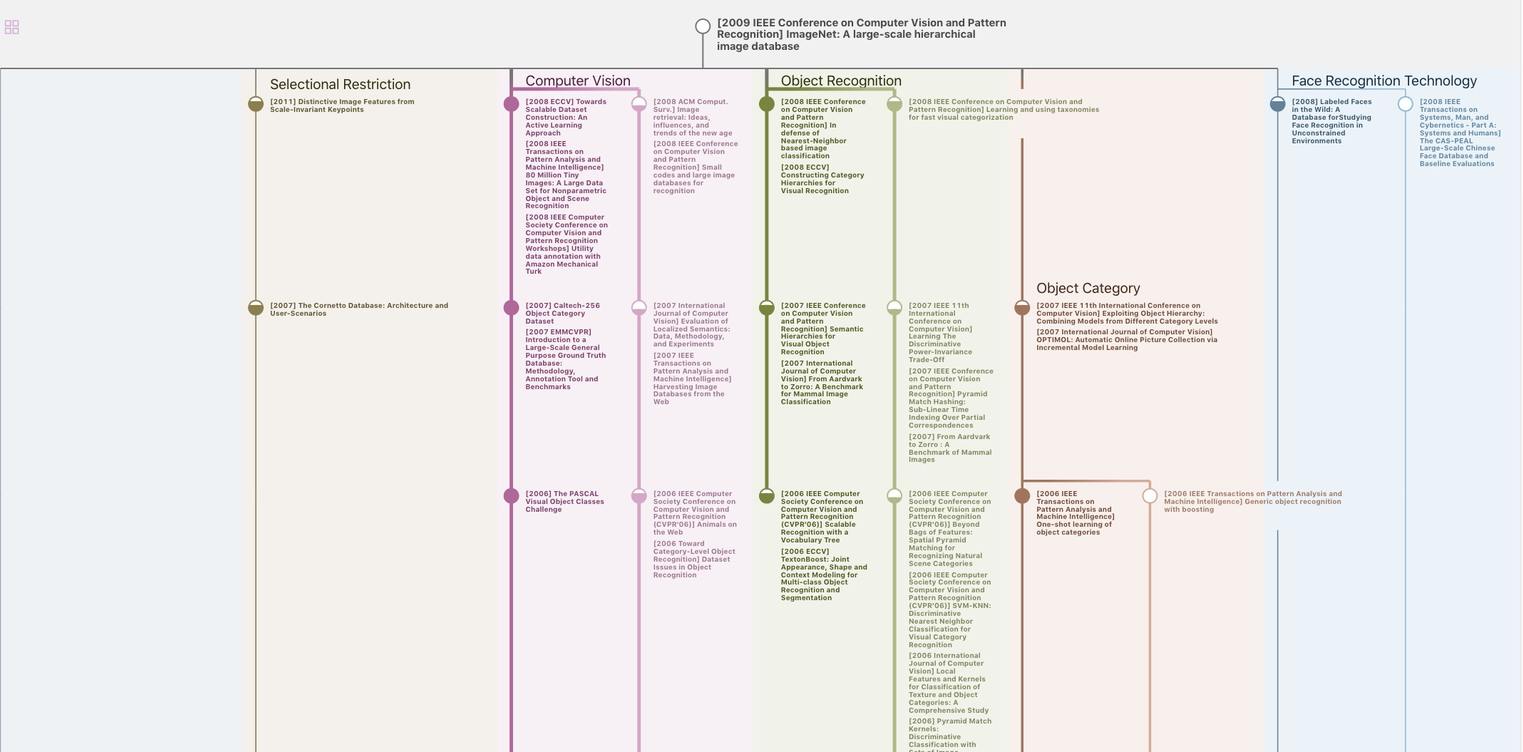
生成溯源树,研究论文发展脉络
Chat Paper
正在生成论文摘要