Improving the Accuracy of Ballot Scanners Using Supervised Learning
ELECTRONIC VOTING, E-VOTE-ID 2021(2021)
摘要
Most U.S. voters cast hand-marked paper ballots that are counted by optical scanners. Deployed ballot scanners typically utilize simplistic mark-detection methods, based on comparing the measured intensity of target areas to preset thresholds, but this technique is known to sometimes misread "marginal" marks that deviate from ballot instructions. We investigate the feasibility of improving scanner accuracy using supervised learning. We train a convolutional neural network to classify various styles of marks extracted from a large corpus of voted ballots. This approach achieves higher accuracy than a naive intensity threshold while requiring far fewer ballots to undergo manual adjudication. It is robust to imperfect feature extraction, as may be experienced in ballots that lack timing marks, and efficient enough to be performed in real time using contemporary central-count scanner hardware.
更多查看译文
关键词
ballot scanners,supervised learning,accuracy
AI 理解论文
溯源树
样例
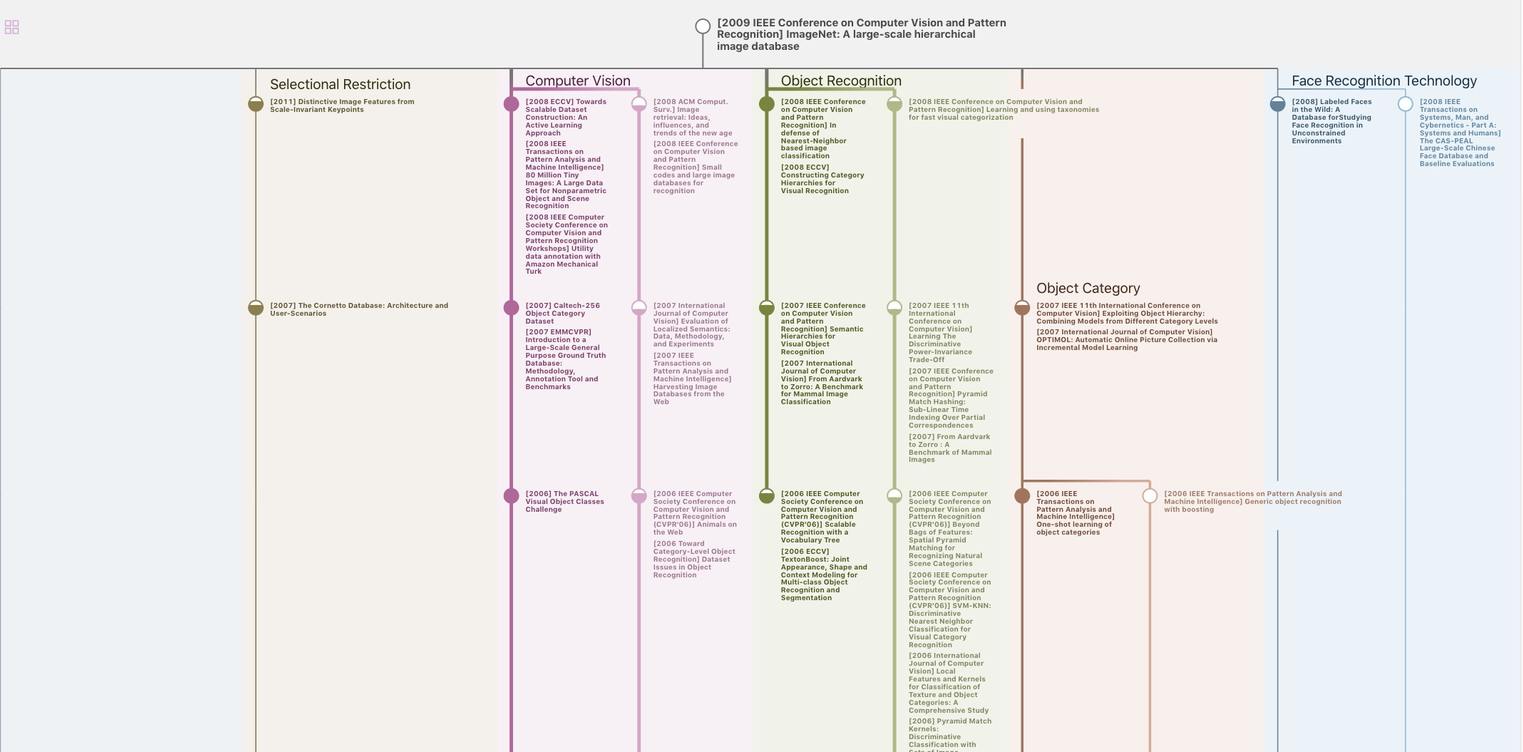
生成溯源树,研究论文发展脉络
Chat Paper
正在生成论文摘要