Deep Learning Based Automatic Detection of Adequately Positioned Mammograms
DOMAIN ADAPTATION AND REPRESENTATION TRANSFER, AND AFFORDABLE HEALTHCARE AND AI FOR RESOURCE DIVERSE GLOBAL HEALTH (DART 2021)(2021)
摘要
Screening mammograms are a routine imaging exam performed to detect breast cancer in its early stages to reduce morbidity and mortality attributed to this disease. In the United States alone, approximately 40 million mammograms are performed each year. In order to maximize the efficacy of breast cancer screening programs proper mammographic positioning is paramount. Proper positioning ensures adequate visualization of breast tissue and is necessary for breast cancer detection. Therefore, breast imaging radiologists must assess each mammogram for the adequacy of positioning before providing a final interpretation of the exam. In this paper we propose a method that mimics and automates the decision-making process performed by breast imaging radiologists to identify adequately positioned mammograms. Our objective is to improve the quality of mammographic positioning and performance, and ultimately reduce repeat visits for patients whose imaging is technically inadequate. If the method is not able to identify the adequately positioned mammogram, the patient will be scanned again. This AI driven method will be useful in reducing the cost and anxiety associated with mammogram scanning. The method can be very useful in serving the needs in developing countries, where mammogram scanning is not considered a routine procedure due to increased cost to the patient. In addition, the methodology can also be used for training the mammogram techs by providing actionable feedback during scan. The proposed method has a true positive rate of 91.4% for detecting a correctly positioned mediolateral oblique view. For detecting a correctly positioned craniocaudal view, the true positive rate is 95.11%.
更多查看译文
关键词
Mammogram, Mammography positioning, Breast cancer, Deep learning, MQSA
AI 理解论文
溯源树
样例
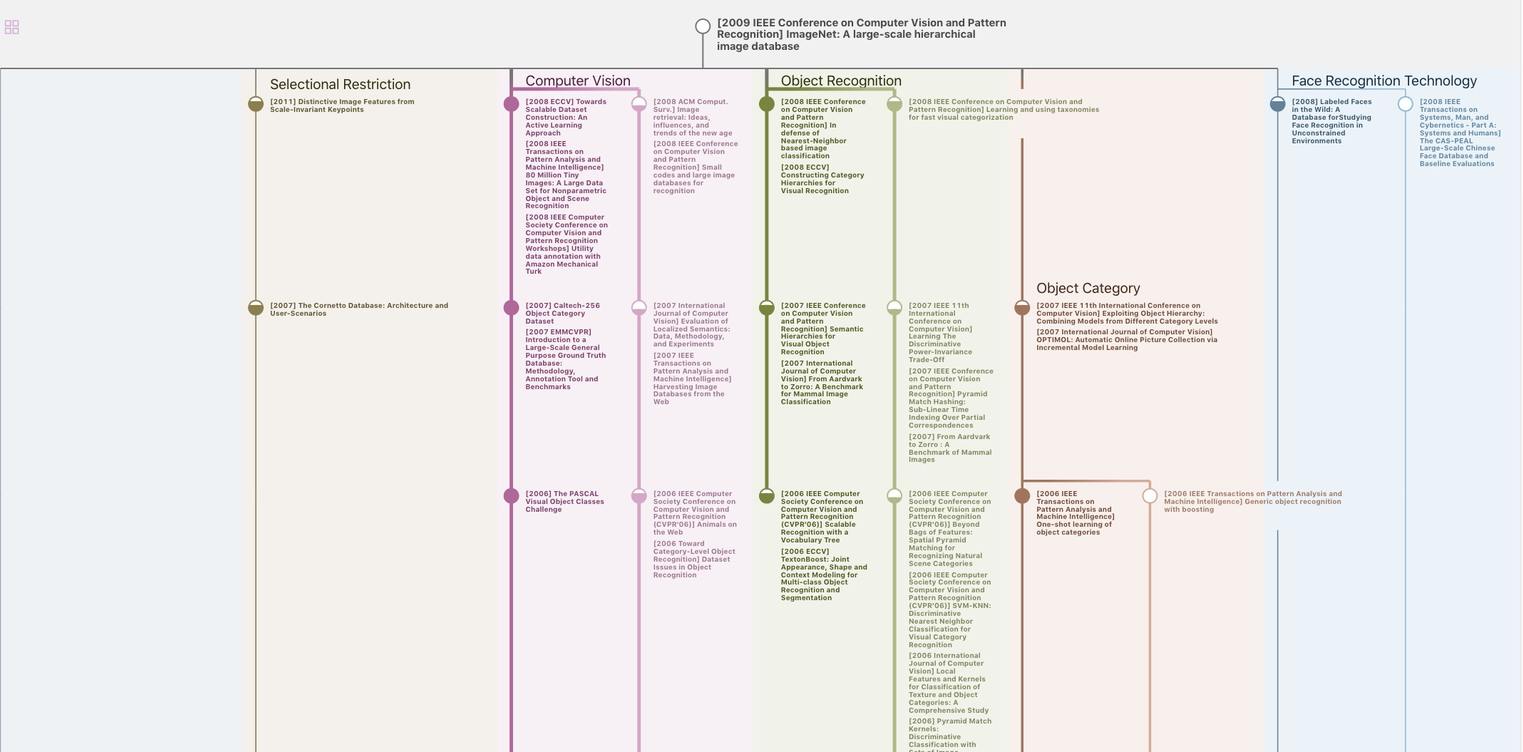
生成溯源树,研究论文发展脉络
Chat Paper
正在生成论文摘要