Multi‐agent spectrum access with sensing skipping based on reinforcement learning
Periodicals(2021)
摘要
AbstractAbstractThe spectrum sharing among high‐density users in the dynamic spectrum environment of future mobile communication systems is analyzed in this article. First, the channel competitions of multiple users are modeled by multi‐player multi‐armed bandit. Then, a channel selection strategy based on the distributed reinforcement learning is proposed, with which the channel selection could be optimized online in accordance to the real‐time competition condition. Thus the collisions of channel access can be effectively reduced with relatively less cooperation overhead. Furthermore, a Bayesian estimation‐based spectrum sensing skipping scheme is designed, which avoids unnecessary spectrum sensing through predicting the idle time of channels. Simulation results demonstrate that the the spectrum sensing times can be reduced by at least 37% and the system throughput can be improved by 1.5 times compared to existing solutions.For the problem of multi‐channel spectrum sharing, a channel selection strategy based on distributed reinforcement learning is proposed, which takes both channel sensing times and channel collisions into account. Simulation results demonstrate that the numbers of the spectrum sensing times can be reduced by at least 37% and the system throughput can be improved by 1.5 times compared to existing solutions. View Figure
更多查看译文
AI 理解论文
溯源树
样例
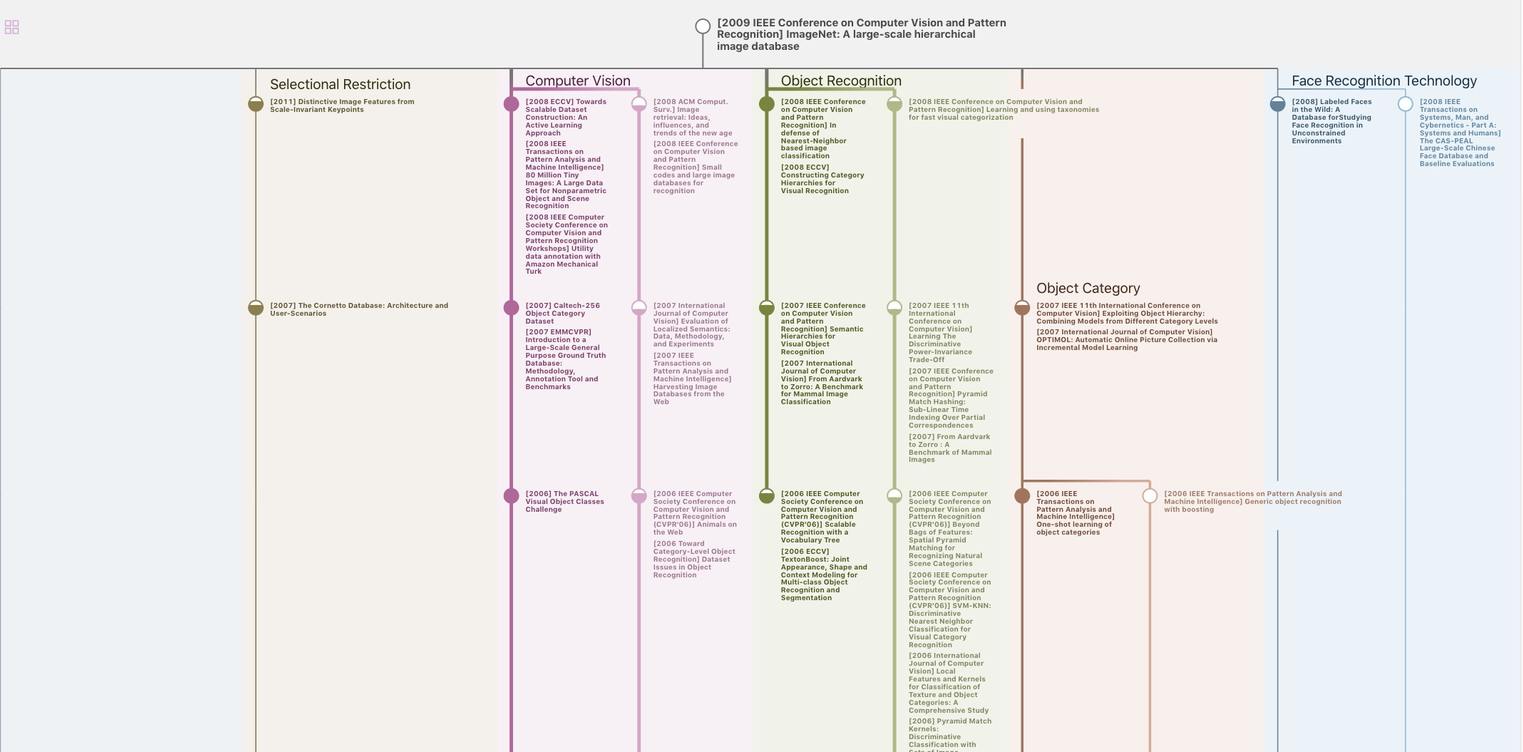
生成溯源树,研究论文发展脉络
Chat Paper
正在生成论文摘要