A High-Density Fish School Segmentation Framework For Biomass Statistics In A Deep-Sea Cage
ECOLOGICAL INFORMATICS(2021)
摘要
Video monitoring systems are successfully widely used in many on-land artificial intelligence applications. They have been introduced into fishery production in recent years, such as video-based live fish detection and biomass estimation. Such ways help protect the sea environment by avoiding overstocking and pollution by ocean disasters or human mistakes in the production. However, underwater detection and segmentation are now still challenging because of the complex and volatile environment. The paper proposes an efficient underwater fish school segmentation framework for live fish detection and counting in the high-density cage. Adaptive multiscale Gaussian background models are first constructed frame by frame to separate the foreground fish groups from the background seawater. The fish groups are then divided into individual fish by density estimation using directional weighted convolution kernels. No other underwater video pre-processing algorithms are introduced in the framework. The framework only needs real-time video frames as input. It uses online segmentation algorithms to detect and count live fish. No other pre-collected labeled videos are used to train and fine-tune the framework. It shows robust detection and statistics results in a natural aquaculture deep-sea cage.
更多查看译文
关键词
High-density fish school segmentation, Multi-scale Gaussian background model, Directional weighted convolution kernel, Automated fish counting
AI 理解论文
溯源树
样例
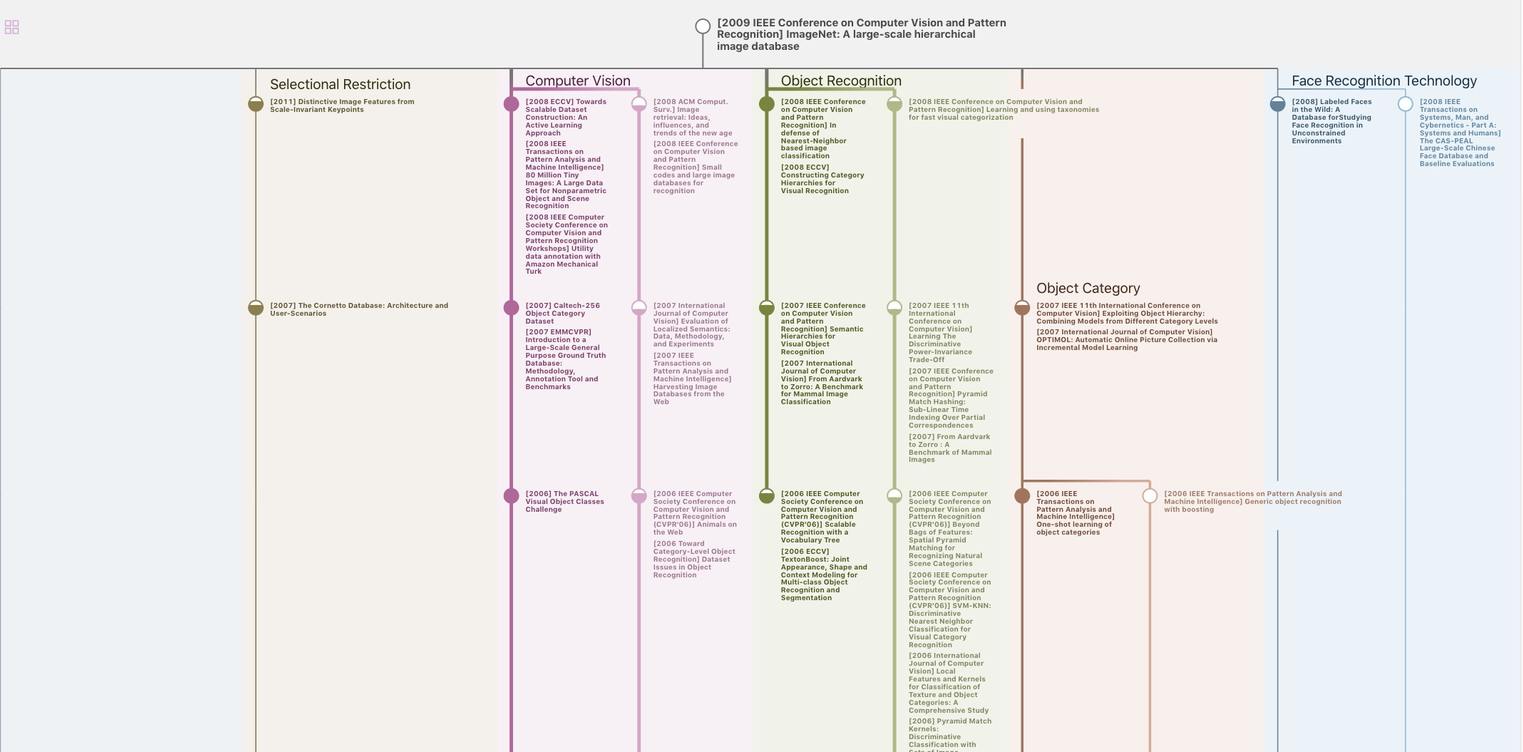
生成溯源树,研究论文发展脉络
Chat Paper
正在生成论文摘要