Robust Temporal Ensembling for Learning with Noisy Labels
arxiv(2021)
摘要
Successful training of deep neural networks with noisy labels is an essential capability as most real-world datasets contain some amount of mislabeled data. Left unmitigated, label noise can sharply degrade typical supervised learning approaches. In this paper, we present robust temporal ensembling (RTE), which combines robust loss with semi-supervised regularization methods to achieve noise-robust learning. We demonstrate that RTE achieves state-of-the-art performance across the CIFAR-10, CIFAR-100, ImageNet, WebVision, and Food-101N datasets, while forgoing the recent trend of label filtering and/or fixing. Finally, we show that RTE also retains competitive corruption robustness to unforeseen input noise using CIFAR-10-C, obtaining a mean corruption error (mCE) of 13.50% even in the presence of an 80% noise ratio, versus 26.9% mCE with standard methods on clean data.
更多查看译文
关键词
robust,learning,labels
AI 理解论文
溯源树
样例
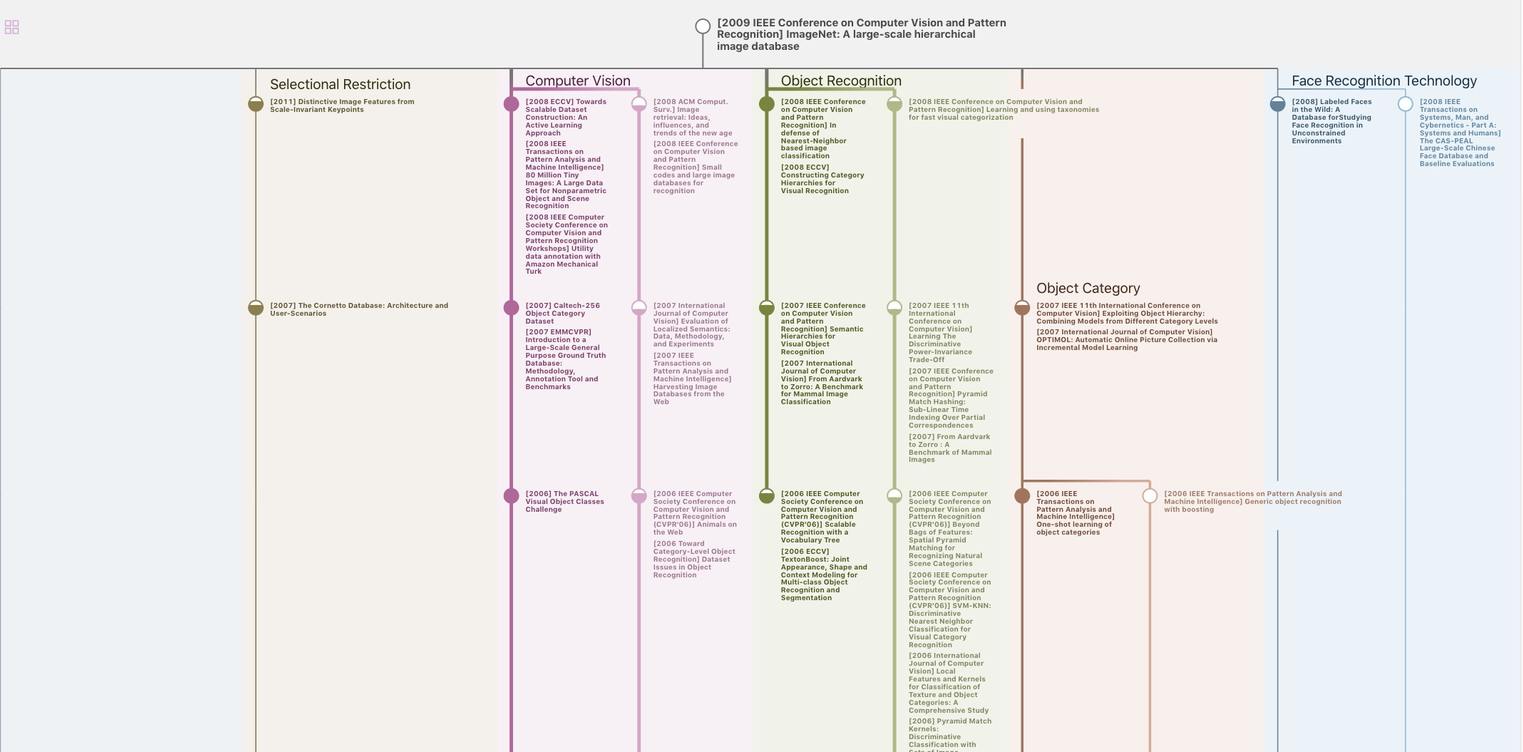
生成溯源树,研究论文发展脉络
Chat Paper
正在生成论文摘要