Automatic discovery and description of human planning strategies
Behavior research methods(2021)
摘要
Scientific discovery concerns finding patterns in data and creating insightful hypotheses that explain these patterns. Traditionally, this process required human ingenuity, but with the galloping advances in artificial intelligence (AI) it becomes feasible to automate some parts of scientific discovery. In this work we leverage AI for strategy discovery for understanding human planning. In the state-of-the-art methods data about the process of human planning is often used to group similar behaviors together and formulate verbal descriptions of the strategies which might underlie those groups. Here, we automate these two steps. Our algorithm, called Human-Interpret, uses imitation learning to describe process-tracing data collected in psychological experiments with the Mouselab-MDP paradigm in terms of a procedural formula. Then, it translates that formula to natural language using a pre-defined predicate dictionary. We test our method on a benchmark data set that researchers have previously scrutinized manually. We find that the descriptions of human planning strategies obtained automatically are about as understandable as human-generated descriptions. They also cover a substantial proportion of all types of human planning strategies that had been discovered manually. Our method saves scientists' time and effort as all the reasoning about human planning is done automatically. This might make it feasible to more rapidly scale up the search for yet undiscovered cognitive strategies to many new decision environments, populations, tasks, and domains. Given these results, we believe that the presented work may accelerate scientific discovery in psychology, and due to its generality, extend to problems from other fields.
更多查看译文
AI 理解论文
溯源树
样例
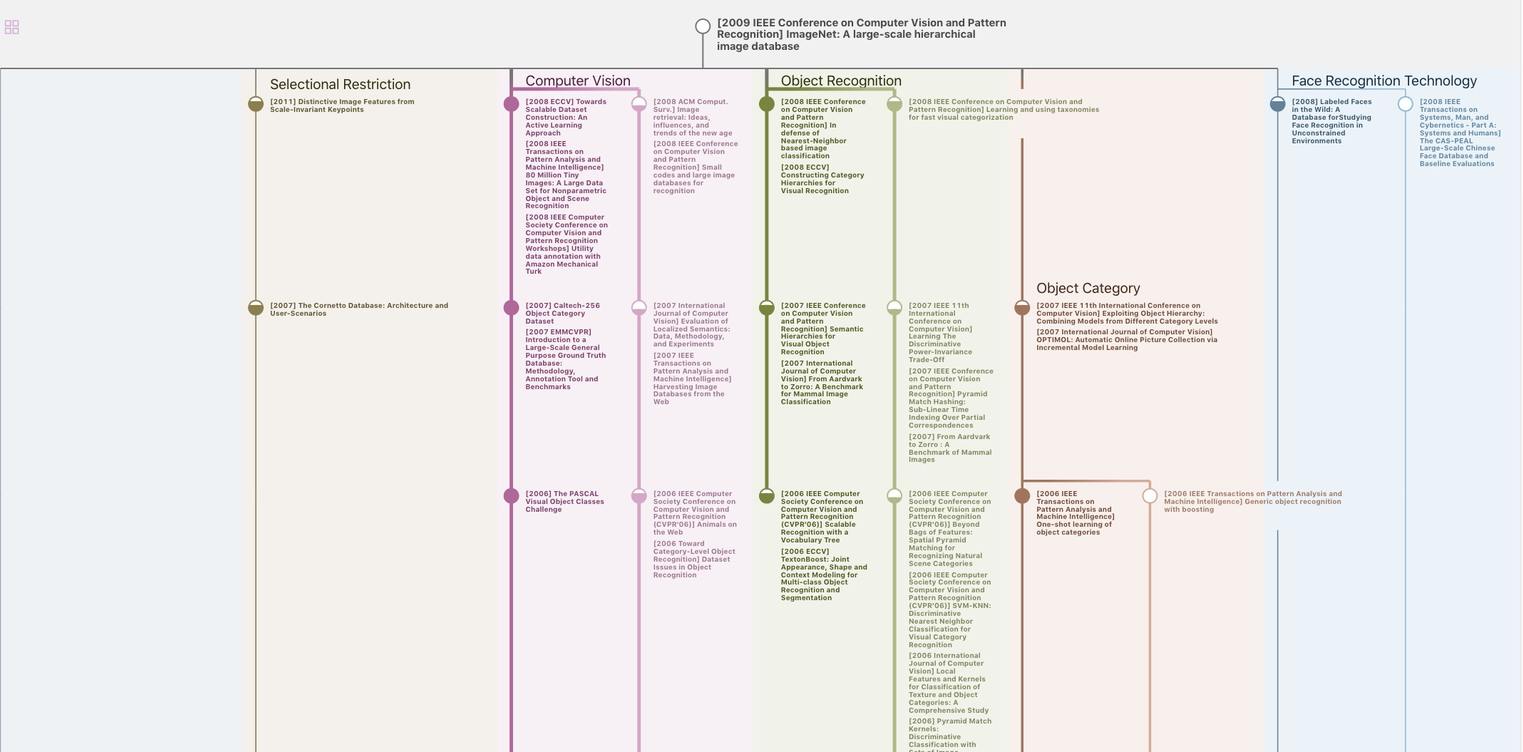
生成溯源树,研究论文发展脉络
Chat Paper
正在生成论文摘要