Influence of Binomial Crossover on Approximation Error of Evolutionary Algorithms
arxiv(2022)
摘要
Although differential evolution (DE) algorithms perform well on a large variety of complicated optimization problems, only a few theoretical studies are focused on the working principle of DE algorithms. To make the first attempt to reveal the function of binomial crossover, this paper aims to answer whether it can reduce the approximation error of evolutionary algorithms. By investigating the expected approximation error and the probability of not finding the optimum, we conduct a case study comparing two evolutionary algorithms with and without binomial crossover on two classical benchmark problems: OneMax and Deceptive. It is proven that using binomial crossover leads to the dominance of transition matrices. As a result, the algorithm with binomial crossover asymptotically outperforms that without crossover on both OneMax and Deceptive, and outperforms on OneMax, however, not on Deceptive. Furthermore, an adaptive parameter strategy is proposed which can strengthen the superiority of binomial crossover on Deceptive.
更多查看译文
关键词
binomial crossover, differential evolution, fixed-budget analysis, evolutionary computation, approximation error
AI 理解论文
溯源树
样例
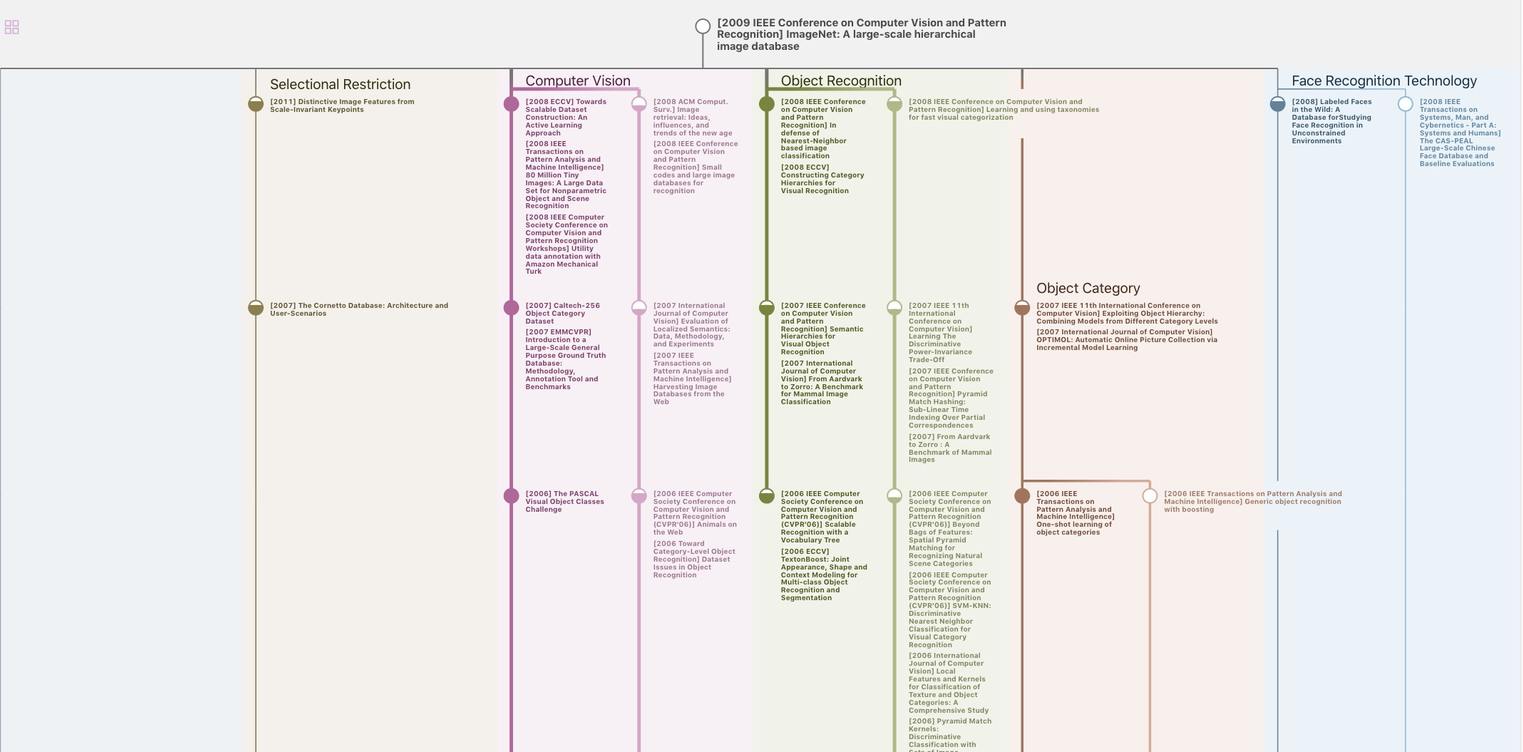
生成溯源树,研究论文发展脉络
Chat Paper
正在生成论文摘要