Adaptive Multi-layer Contrastive Graph Neural Networks
ArXiv(2022)
摘要
Inspired by recent success of graph contrastive learning methods, we propose a self-supervised learning framework for Graph Neural Networks (GNNs) named Adaptive Multi-layer Contrastive Graph Neural Networks (AMC-GNN). Specifically, AMC-GNN generates different graph views through data augmentation and compares the output embeddings at different layers of graph neural network encoders to obtain feature representations for downstream tasks. Meanwhile, AMC-GNN learns the importance weights of embeddings at different layers adaptively through the attention mechanism, and an auxiliary encoder is adopted to train graph contrastive encoders better. The accuracy is improved by maximizing the representation’s consistency of positive pairs in the intermediate layers and the final embedding space. Experiments on node classification and link prediction demonstrate that the AMC-GNN framework outperforms state-of-the-art contrastive learning methods and even sometimes outperforms supervised methods.
更多查看译文
关键词
Graph neural network,Contrastive learning,Node representation,Adaptive multi-layer contrastive loss
AI 理解论文
溯源树
样例
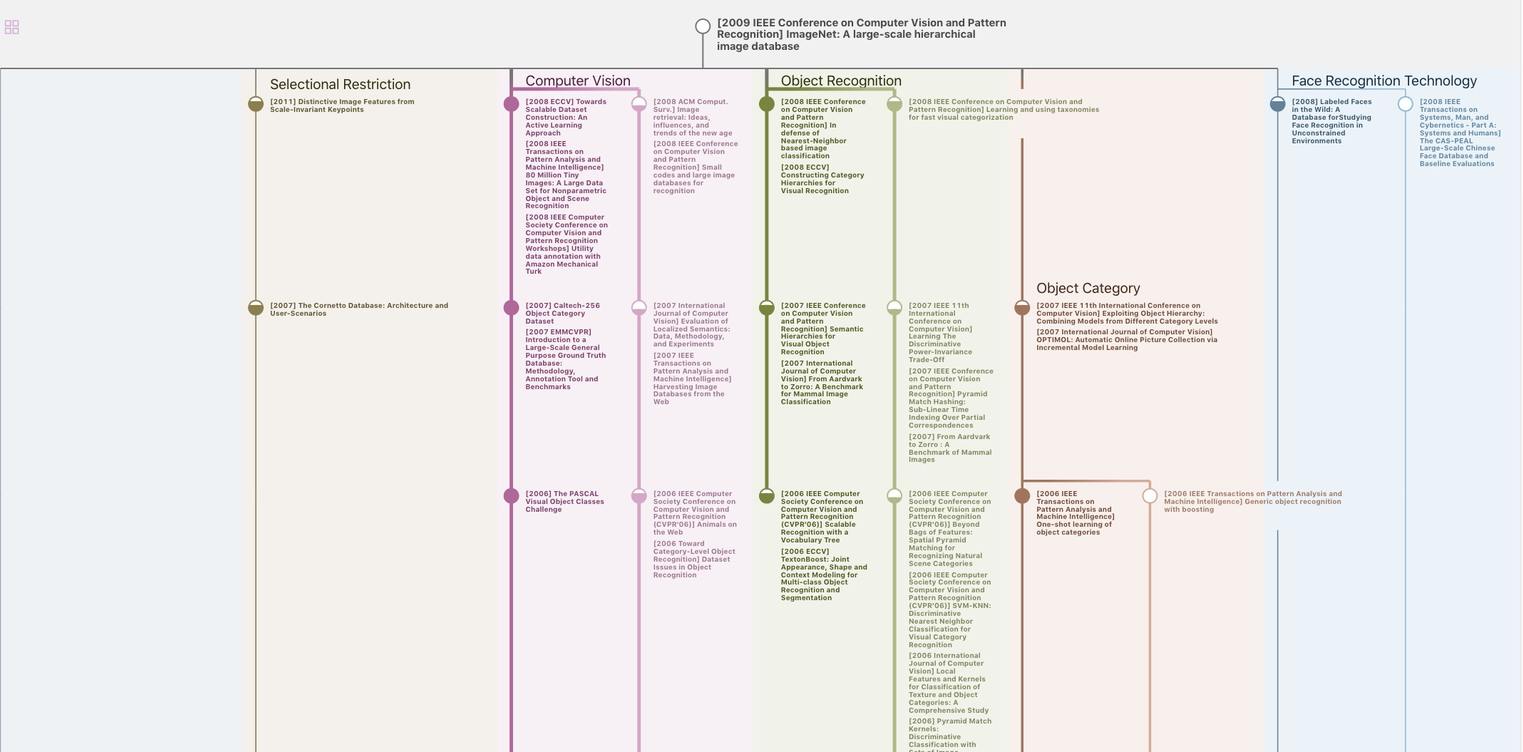
生成溯源树,研究论文发展脉络
Chat Paper
正在生成论文摘要