Efficient Text-based Reinforcement Learning by Jointly Leveraging State and Commonsense Graph Representations.
ACL-IJCNLP 2021: THE 59TH ANNUAL MEETING OF THE ASSOCIATION FOR COMPUTATIONAL LINGUISTICS AND THE 11TH INTERNATIONAL JOINT CONFERENCE ON NATURAL LANGUAGE PROCESSING, VOL 2(2021)
摘要
Text-based games (TBGs) have emerged as useful benchmarks for evaluating progress at the intersection of grounded language understanding and reinforcement learning (RL). Recent work has proposed the use of external knowledge to improve the efficiency of RL agents for TBGs. In this paper, we posit that to act efficiently in TBGs, an agent must be able to track the state of the game while retrieving and using relevant commonsense knowledge. Thus, we propose an agent for TBGs that induces a graph representation of the game state and jointly grounds it with a graph of commonsense knowledge from ConceptNet. This combination is achieved through bidirectional knowledge graph attention between the two symbolic representations. We show that agents that incorporate commonsense into the game state graph outperform baseline agents.
更多查看译文
关键词
Commonsense knowledge,Graph (abstract data type),Reinforcement learning,Artificial intelligence,Intersection (set theory),Computer science,State (computer science),Baseline (configuration management),Language understanding,State graph
AI 理解论文
溯源树
样例
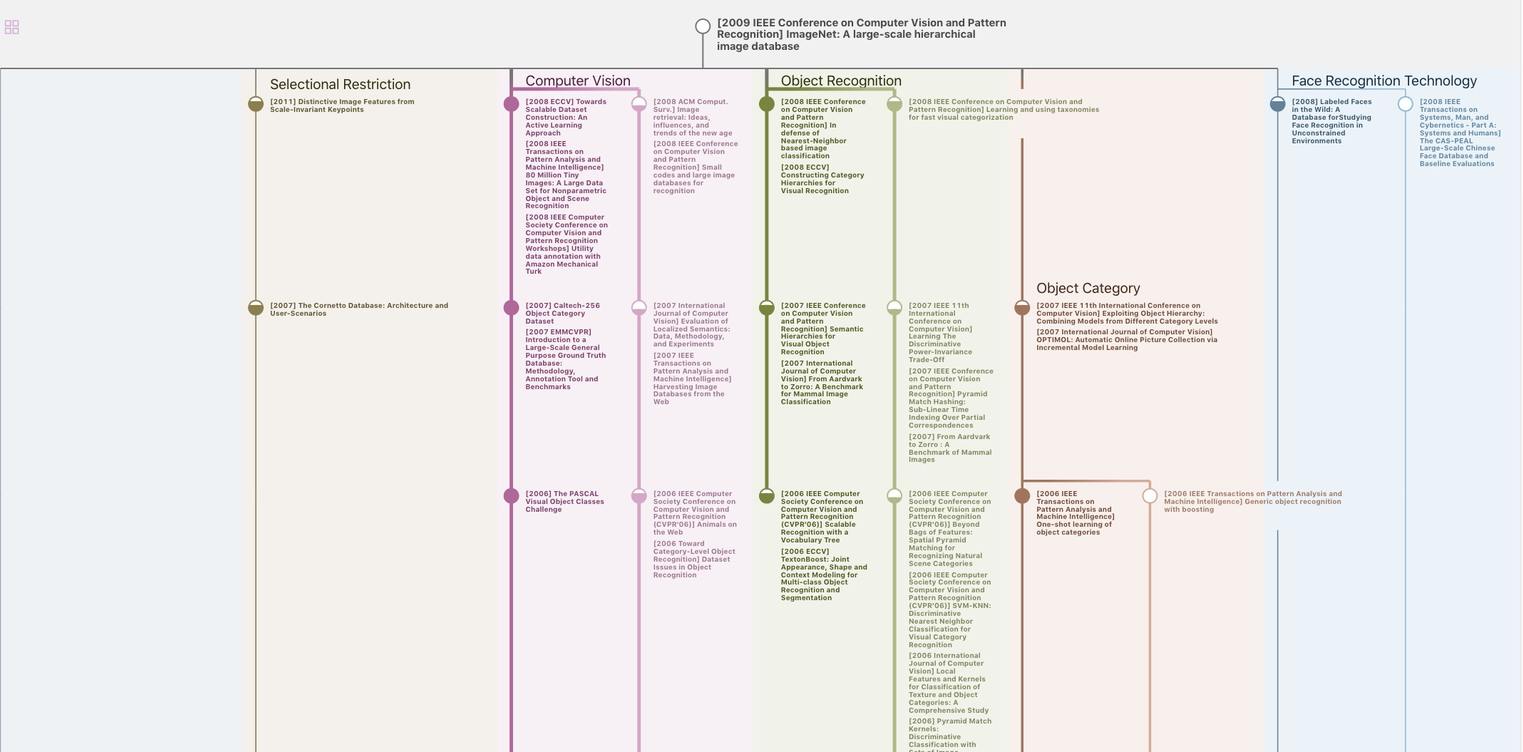
生成溯源树,研究论文发展脉络
Chat Paper
正在生成论文摘要