Neural Embedded Dirichlet Processes for Topic Modeling.
MDAI(2021)
摘要
This paper presents two novel models: the neural Embedded Dirichlet Process and its hierarchical version, the neural Embedded Hierarchical Dirichlet Process. Both methods extend the Embedded Topic Model (ETM) to nonparametric settings, thus simultaneously learning the number of topics, latent representations of documents, and topic and word embeddings from data. To achieve this, we replace ETM's logistic normal prior over a Gaussian with a Dirichlet Process and a Hierarchical Dirichlet Process in a variational autoencoding inference setting. We test our models on the 20 Newsgroups and on the Humanitarian Assistance and Disaster Relief datasets. Our models present the advantage of maintaining low perplexity while providing analysts with meaningful document, topic and word representations that outperform other state of the art methods, while avoiding costly reruns on large datasets, even in a multilingual context.
更多查看译文
关键词
Topic modeling,Text mining,Natural language processing,Deep learning
AI 理解论文
溯源树
样例
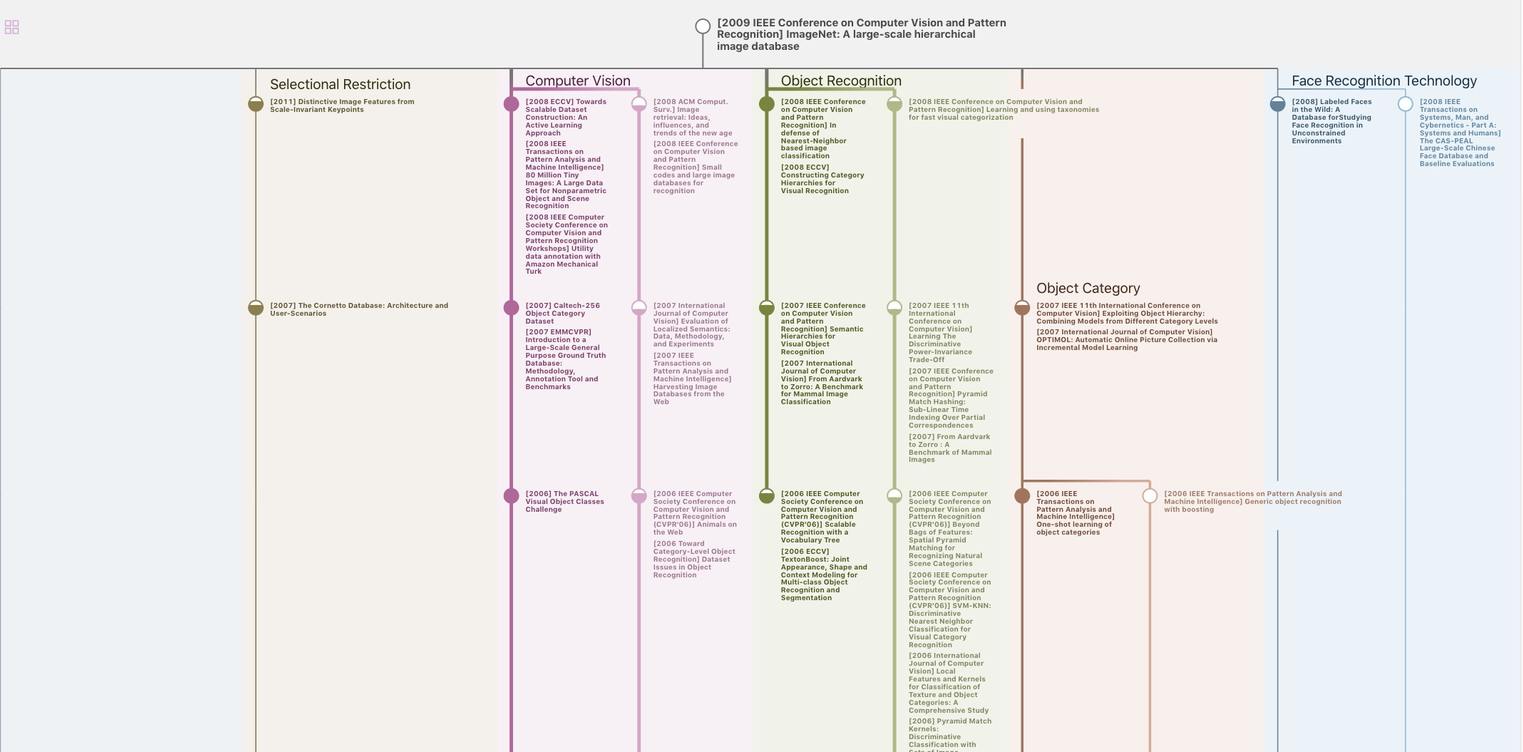
生成溯源树,研究论文发展脉络
Chat Paper
正在生成论文摘要